Open Access
ARTICLE
Intelligent Machine Learning Based Brain Tumor Segmentation through Multi-Layer Hybrid U-Net with CNN Feature Integration
Department of Information Technology, Faculty of Computing and Information Technology, King Abdulaziz University, P.O. Box 344, Rabigh, 21911, Saudia Arabia
* Corresponding Author: Sharaf J. Malebary. Email:
(This article belongs to the Special Issue: Intelligent Management and Machine Learning for Big Data in IoT-Enabled Pervasive Computing)
Computers, Materials & Continua 2024, 79(1), 1301-1317. https://doi.org/10.32604/cmc.2024.047917
Received 22 November 2023; Accepted 08 March 2024; Issue published 25 April 2024
Abstract
Brain tumors are a pressing public health concern, characterized by their high mortality and morbidity rates. Nevertheless, the manual segmentation of brain tumors remains a laborious and error-prone task, necessitating the development of more precise and efficient methodologies. To address this formidable challenge, we propose an advanced approach for segmenting brain tumor Magnetic Resonance Imaging (MRI) images that harnesses the formidable capabilities of deep learning and convolutional neural networks (CNNs). While CNN-based methods have displayed promise in the realm of brain tumor segmentation, the intricate nature of these tumors, marked by irregular shapes, varying sizes, uneven distribution, and limited available data, poses substantial obstacles to achieving accurate semantic segmentation. In our study, we introduce a pioneering Hybrid U-Net framework that seamlessly integrates the U-Net and CNN architectures to surmount these challenges. Our proposed approach encompasses preprocessing steps that enhance image visualization, a customized layered U-Net model tailored for precise segmentation, and the inclusion of dropout layers to mitigate overfitting during the training process. Additionally, we leverage the CNN mechanism to exploit contextual information within brain tumor MRI images, resulting in a substantial enhancement in segmentation accuracy. Our experimental results attest to the exceptional performance of our framework, with accuracy rates surpassing 97% across diverse datasets, showcasing the robustness and effectiveness of our approach. Furthermore, we conduct a comprehensive assessment of our method’s capabilities by evaluating various performance measures, including the sensitivity, Jaccard-index, and specificity. Our proposed model achieved 99% accuracy. The implications of our findings are profound. The proposed Hybrid U-Net model emerges as a highly promising diagnostic tool, poised to revolutionize brain tumor image segmentation for radiologists and clinicians.Keywords
Cite This Article
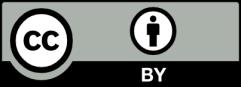