Open Access
ARTICLE
An Enhanced Multiview Transformer for Population Density Estimation Using Cellular Mobility Data in Smart City
1 College of Computer Science and Software Engineering, Shenzhen University, Shenzhen, 518060, China
2 College of Management, Shenzhen University, Shenzhen, 518060, China
3 Information Center, The First Affiliated Hospital of Dalian Medical University, Dalian, 116011, China
* Corresponding Author: Dandan Yu. Email:
(This article belongs to the Special Issue: The Next-generation Deep Learning Approaches to Emerging Real-world Applications)
Computers, Materials & Continua 2024, 79(1), 161-182. https://doi.org/10.32604/cmc.2024.047836
Received 20 November 2023; Accepted 08 January 2024; Issue published 25 April 2024
Abstract
This paper addresses the problem of predicting population density leveraging cellular station data. As wireless communication devices are commonly used, cellular station data has become integral for estimating population figures and studying their movement, thereby implying significant contributions to urban planning. However, existing research grapples with issues pertinent to preprocessing base station data and the modeling of population prediction. To address this, we propose methodologies for preprocessing cellular station data to eliminate any irregular or redundant data. The preprocessing reveals a distinct cyclical characteristic and high-frequency variation in population shift. Further, we devise a multi-view enhancement model grounded on the Transformer (MVformer), targeting the improvement of the accuracy of extended time-series population predictions. Comparative experiments, conducted on the above-mentioned population dataset using four alternate Transformer-based models, indicate that our proposed MVformer model enhances prediction accuracy by approximately 30% for both univariate and multivariate time-series prediction assignments. The performance of this model in tasks pertaining to population prediction exhibits commendable results.Keywords
Cite This Article
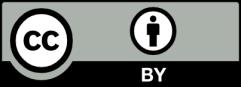
This work is licensed under a Creative Commons Attribution 4.0 International License , which permits unrestricted use, distribution, and reproduction in any medium, provided the original work is properly cited.