Open Access
ARTICLE
A Simple and Effective Surface Defect Detection Method of Power Line Insulators for Difficult Small Objects
1 State Grid Jiangsu Electric Power Co., Ltd., Nanjing, 210024, China
2 State Grid Changzhou Power Supply Company, Changzhou, 213003, China
* Corresponding Author: Xiao Lu. Email:
(This article belongs to the Special Issue: Machine Vision Detection and Intelligent Recognition)
Computers, Materials & Continua 2024, 79(1), 373-390. https://doi.org/10.32604/cmc.2024.047469
Received 06 November 2023; Accepted 02 February 2024; Issue published 25 April 2024
Abstract
Insulator defect detection plays a vital role in maintaining the secure operation of power systems. To address the issues of the difficulty of detecting small objects and missing objects due to the small scale, variable scale, and fuzzy edge morphology of insulator defects, we construct an insulator dataset with 1600 samples containing flashovers and breakages. Then a simple and effective surface defect detection method of power line insulators for difficult small objects is proposed. Firstly, a high-resolution feature map is introduced and a small object prediction layer is added so that the model can detect tiny objects. Secondly, a simplified adaptive spatial feature fusion (S-ASFF) module is introduced to perform cross-scale spatial fusion to improve adaptability to variable multi-scale features. Finally, we propose an enhanced deformable attention mechanism (EDAM) module. By integrating a gating activation function, the model is further inspired to learn a small number of critical sampling points near reference points. And the module can improve the perception of object morphology. The experimental results indicate that concerning the dataset of flashover and breakage defects, this method improves the performance of YOLOv5, YOLOv7, and YOLOv8. In practical application, it can simply and effectively improve the precision of power line insulator defect detection and reduce missing detection for difficult small objects.Keywords
Cite This Article
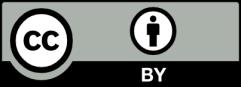
This work is licensed under a Creative Commons Attribution 4.0 International License , which permits unrestricted use, distribution, and reproduction in any medium, provided the original work is properly cited.