Open Access
ARTICLE
Coal/Gangue Volume Estimation with Convolutional Neural Network and Separation Based on Predicted Volume and Weight
1 School of Mechatronic Engineering, China University of Mining and Technology, Xuzhou, 221116, China
2 China National Coal Group Corp., Beijing, 100029, China
3 Information Institute, Ministry of Emergency Management of the China, Beijing, 100029, China
4 School of Mechanical Engineering, Sana’a University, Sana’a, 31220, Yemen
* Corresponding Authors: Murad S. Alfarzaeai. Email: ; Eryi Hu. Email:
(This article belongs to the Special Issue: Advanced Computer Technology for Materials Characterization, Properties Prediction, Design and Discovery)
Computers, Materials & Continua 2024, 79(1), 279-306. https://doi.org/10.32604/cmc.2024.047159
Received 27 October 2023; Accepted 23 January 2024; Issue published 25 April 2024
Abstract
In the coal mining industry, the gangue separation phase imposes a key challenge due to the high visual similarity between coal and gangue. Recently, separation methods have become more intelligent and efficient, using new technologies and applying different features for recognition. One such method exploits the difference in substance density, leading to excellent coal/gangue recognition. Therefore, this study uses density differences to distinguish coal from gangue by performing volume prediction on the samples. Our training samples maintain a record of 3-side images as input, volume, and weight as the ground truth for the classification. The prediction process relies on a Convolutional neural network (CGVP-CNN) model that receives an input of a 3-side image and then extracts the needed features to estimate an approximation for the volume. The classification was comparatively performed via ten different classifiers, namely, K-Nearest Neighbors (KNN), Linear Support Vector Machines (Linear SVM), Radial Basis Function (RBF) SVM, Gaussian Process, Decision Tree, Random Forest, Multi-Layer Perceptron (MLP), Adaptive Boosting (AdaBosst), Naive Bayes, and Quadratic Discriminant Analysis (QDA). After several experiments on testing and training data, results yield a classification accuracy of 100%, 92%, 95%, 96%, 100%, 100%, 100%, 96%, 81%, and 92%, respectively. The test reveals the best timing with KNN, which maintained an accuracy level of 100%. Assessing the model generalization capability to new data is essential to ensure the efficiency of the model, so by applying a cross-validation experiment, the model generalization was measured. The used dataset was isolated based on the volume values to ensure the model generalization not only on new images of the same volume but with a volume outside the trained range. Then, the predicted volume values were passed to the classifiers group, where classification reported accuracy was found to be (100%, 100%, 100%, 98%, 88%, 87%, 100%, 87%, 97%, 100%), respectively. Although obtaining a classification with high accuracy is the main motive, this work has a remarkable reduction in the data preprocessing time compared to related works. The CGVP-CNN model managed to reduce the data preprocessing time of previous works to 0.017 s while maintaining high classification accuracy using the estimated volume value.Keywords
Cite This Article
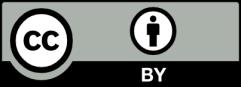
This work is licensed under a Creative Commons Attribution 4.0 International License , which permits unrestricted use, distribution, and reproduction in any medium, provided the original work is properly cited.