Open Access
ARTICLE
Double DQN Method For Botnet Traffic Detection System
1 School of Information Science and Engineering, Shenyang Ligong University, Shenyang, 110159, China
2 Graduate School, Shenyang Ligong University, Shenyang, 110159, China
* Corresponding Author: Yuntao Zhao. Email:
Computers, Materials & Continua 2024, 79(1), 509-530. https://doi.org/10.32604/cmc.2024.042216
Received 23 May 2023; Accepted 19 February 2024; Issue published 25 April 2024
Abstract
In the face of the increasingly severe Botnet problem on the Internet, how to effectively detect Botnet traffic in real-time has become a critical problem. Although the existing deep Q network (DQN) algorithm in Deep reinforcement learning can solve the problem of real-time updating, its prediction results are always higher than the actual results. In Botnet traffic detection, although it performs well in the training set, the accuracy rate of predicting traffic is as high as%; however, in the test set, its accuracy has declined, and it is impossible to adjust its prediction strategy on time based on new data samples. However, in the new dataset, its accuracy has declined significantly. Therefore, this paper proposes a Botnet traffic detection system based on double-layer DQN (DDQN). Two Q-values are designed to adjust the model in policy and action, respectively, to achieve real-time model updates and improve the universality and robustness of the model under different data sets. Experiments show that compared with the DQN model, when using DDQN, the Q-value is not too high, and the detection model has improved the accuracy and precision of Botnet traffic. Moreover, when using Botnet data sets other than the test set, the accuracy and precision of the DDQN model are still higher than DQN.Keywords
Cite This Article
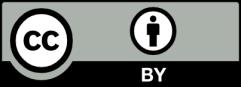
This work is licensed under a Creative Commons Attribution 4.0 International License , which permits unrestricted use, distribution, and reproduction in any medium, provided the original work is properly cited.