Open Access
ARTICLE
VKFQ: A Verifiable Keyword Frequency Query Framework with Local Differential Privacy in Blockchain
School of Computer and Communication Engineering, Changsha University of Science and Technology, Changsha, 410114, China
* Corresponding Author: Bo Yin. Email:
Computers, Materials & Continua 2024, 78(3), 4205-4223. https://doi.org/10.32604/cmc.2024.049086
Received 27 December 2023; Accepted 08 February 2024; Issue published 26 March 2024
Abstract
With its untameable and traceable properties, blockchain technology has been widely used in the field of data sharing. How to preserve individual privacy while enabling efficient data queries is one of the primary issues with secure data sharing. In this paper, we study verifiable keyword frequency (KF) queries with local differential privacy in blockchain. Both the numerical and the keyword attributes are present in data objects; the latter are sensitive and require privacy protection. However, prior studies in blockchain have the problem of trilemma in privacy protection and are unable to handle KF queries. We propose an efficient framework that protects data owners’ privacy on keyword attributes while enabling quick and verifiable query processing for KF queries. The framework computes an estimate of a keyword’s frequency and is efficient in query time and verification object (VO) size. A utility-optimized local differential privacy technique is used for privacy protection. The data owner adds noise locally into data based on local differential privacy so that the attacker cannot infer the owner of the keywords while keeping the difference in the probability distribution of the KF within the privacy budget. We propose the VB-cm tree as the authenticated data structure (ADS). The VB-cm tree combines the Verkle tree and the Count-Min sketch (CM-sketch) to lower the VO size and query time. The VB-cm tree uses the vector commitment to verify the query results. The fixed-size CM-sketch, which summarizes the frequency of multiple keywords, is used to estimate the KF via hashing operations. We conduct an extensive evaluation of the proposed framework. The experimental results show that compared to the Merkle B+ tree, the query time is reduced by 52.38%, and the VO size is reduced by more than one order of magnitude.Keywords
Cite This Article
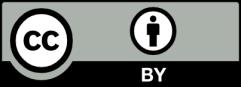
This work is licensed under a Creative Commons Attribution 4.0 International License , which permits unrestricted use, distribution, and reproduction in any medium, provided the original work is properly cited.