Open Access
ARTICLE
BCCLR: A Skeleton-Based Action Recognition with Graph Convolutional Network Combining Behavior Dependence and Context Clues
1 College of Information Science and Engineering, Institute of Interdisciplinary Studies, Hunan Normal University, Changsha, 410081, China
2 School of Educational Sciences, Institute of Interdisciplinary Studies, Hunan Normal University, Changsha, 410081, China
* Corresponding Author: Shuai Liu. Email:
(This article belongs to the Special Issue: Multimodal Learning in Image Processing)
Computers, Materials & Continua 2024, 78(3), 4489-4507. https://doi.org/10.32604/cmc.2024.048813
Received 19 December 2023; Accepted 04 March 2024; Issue published 26 March 2024
Abstract
In recent years, skeleton-based action recognition has made great achievements in Computer Vision. A graph convolutional network (GCN) is effective for action recognition, modelling the human skeleton as a spatio-temporal graph. Most GCNs define the graph topology by physical relations of the human joints. However, this predefined graph ignores the spatial relationship between non-adjacent joint pairs in special actions and the behavior dependence between joint pairs, resulting in a low recognition rate for specific actions with implicit correlation between joint pairs. In addition, existing methods ignore the trend correlation between adjacent frames within an action and context clues, leading to erroneous action recognition with similar poses. Therefore, this study proposes a learnable GCN based on behavior dependence, which considers implicit joint correlation by constructing a dynamic learnable graph with extraction of specific behavior dependence of joint pairs. By using the weight relationship between the joint pairs, an adaptive model is constructed. It also designs a self-attention module to obtain their inter-frame topological relationship for exploring the context of actions. Combining the shared topology and the multi-head self-attention map, the module obtains the context-based clue topology to update the dynamic graph convolution, achieving accurate recognition of different actions with similar poses. Detailed experiments on public datasets demonstrate that the proposed method achieves better results and realizes higher quality representation of actions under various evaluation protocols compared to state-of-the-art methods.Keywords
Cite This Article
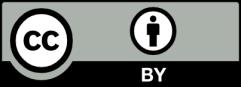
This work is licensed under a Creative Commons Attribution 4.0 International License , which permits unrestricted use, distribution, and reproduction in any medium, provided the original work is properly cited.