Open Access
ARTICLE
Aspect-Level Sentiment Analysis Based on Deep Learning
1 Department of Communication, University of New South Wales, Sydney, 2052, Australia
2 Higher Vocational Education Publishing Division, Higher Education Press, Beijing, 100029, China
3 State Key Laboratory of Media Convergence and Communication, Communication University of China, Beijing, 100020, China
4 Key Lab of Education Blockchain and Intelligent Technology, Ministry of Education, Guangxi Normal University, Guilin, 541004, China
* Corresponding Author: Tong Yi. Email:
(This article belongs to the Special Issue: The Next-generation Deep Learning Approaches to Emerging Real-world Applications)
Computers, Materials & Continua 2024, 78(3), 3743-3762. https://doi.org/10.32604/cmc.2024.048486
Received 09 December 2023; Accepted 22 January 2024; Issue published 26 March 2024
Abstract
In recent years, deep learning methods have developed rapidly and found application in many fields, including natural language processing. In the field of aspect-level sentiment analysis, deep learning methods can also greatly improve the performance of models. However, previous studies did not take into account the relationship between user feature extraction and contextual terms. To address this issue, we use data feature extraction and deep learning combined to develop an aspect-level sentiment analysis method. To be specific, we design user comment feature extraction (UCFE) to distill salient features from users’ historical comments and transform them into representative user feature vectors. Then, the aspect-sentence graph convolutional neural network (ASGCN) is used to incorporate innovative techniques for calculating adjacency matrices; meanwhile, ASGCN emphasizes capturing nuanced semantics within relationships among aspect words and syntactic dependency types. Afterward, three embedding methods are devised to embed the user feature vector into the ASGCN model. The empirical validations verify the effectiveness of these models, consistently surpassing conventional benchmarks and reaffirming the indispensable role of deep learning in advancing sentiment analysis methodologies.Keywords
Cite This Article
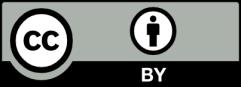
This work is licensed under a Creative Commons Attribution 4.0 International License , which permits unrestricted use, distribution, and reproduction in any medium, provided the original work is properly cited.