Open Access
ARTICLE
TSCND: Temporal Subsequence-Based Convolutional Network with Difference for Time Series Forecasting
MOE Research Center of Software/Hardware Co-Design Engineering, East China Normal University, Shanghai, 200062, China
* Corresponding Author: Weiting Chen. Email:
Computers, Materials & Continua 2024, 78(3), 3665-3681. https://doi.org/10.32604/cmc.2024.048008
Received 24 November 2023; Accepted 11 January 2024; Issue published 26 March 2024
Abstract
Time series forecasting plays an important role in various fields, such as energy, finance, transport, and weather. Temporal convolutional networks (TCNs) based on dilated causal convolution have been widely used in time series forecasting. However, two problems weaken the performance of TCNs. One is that in dilated casual convolution, causal convolution leads to the receptive fields of outputs being concentrated in the earlier part of the input sequence, whereas the recent input information will be severely lost. The other is that the distribution shift problem in time series has not been adequately solved. To address the first problem, we propose a subsequence-based dilated convolution method (SDC). By using multiple convolutional filters to convolve elements of neighboring subsequences, the method extracts temporal features from a growing receptive field via a growing subsequence rather than a single element. Ultimately, the receptive field of each output element can cover the whole input sequence. To address the second problem, we propose a difference and compensation method (DCM). The method reduces the discrepancies between and within the input sequences by difference operations and then compensates the outputs for the information lost due to difference operations. Based on SDC and DCM, we further construct a temporal subsequence-based convolutional network with difference (TSCND) for time series forecasting. The experimental results show that TSCND can reduce prediction mean squared error by 7.3% and save runtime, compared with state-of-the-art models and vanilla TCN.Keywords
Cite This Article
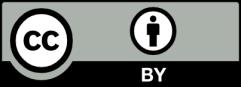
This work is licensed under a Creative Commons Attribution 4.0 International License , which permits unrestricted use, distribution, and reproduction in any medium, provided the original work is properly cited.