Open Access
ARTICLE
An Enhanced Ensemble-Based Long Short-Term Memory Approach for Traffic Volume Prediction
1 Faculty of Civil Engineering, Nha Trang University, Nha Trang, 650000, Vietnam
2 Robotics and Mechatronics Research Group, Faculty of Engineering and Technology, Nguyen Tat Thanh University, Ho Chi Minh City, 700000, Vietnam
3 Department of Civil Engineering, Ho Chi Minh City University of Technology and Education, Ho Chi Minh City, 700000, Vietnam
* Corresponding Author: Huy Q. Tran. Email:
Computers, Materials & Continua 2024, 78(3), 3585-3602. https://doi.org/10.32604/cmc.2024.047760
Received 16 November 2023; Accepted 04 January 2024; Issue published 26 March 2024
Abstract
With the advancement of artificial intelligence, traffic forecasting is gaining more and more interest in optimizing route planning and enhancing service quality. Traffic volume is an influential parameter for planning and operating traffic structures. This study proposed an improved ensemble-based deep learning method to solve traffic volume prediction problems. A set of optimal hyperparameters is also applied for the suggested approach to improve the performance of the learning process. The fusion of these methodologies aims to harness ensemble empirical mode decomposition’s capacity to discern complex traffic patterns and long short-term memory’s proficiency in learning temporal relationships. Firstly, a dataset for automatic vehicle identification is obtained and utilized in the preprocessing stage of the ensemble empirical mode decomposition model. The second aspect involves predicting traffic volume using the long short-term memory algorithm. Next, the study employs a trial-and-error approach to select a set of optimal hyperparameters, including the lookback window, the number of neurons in the hidden layers, and the gradient descent optimization. Finally, the fusion of the obtained results leads to a final traffic volume prediction. The experimental results show that the proposed method outperforms other benchmarks regarding various evaluation measures, including mean absolute error, root mean squared error, mean absolute percentage error, and R-squared. The achieved R-squared value reaches an impressive 98%, while the other evaluation indices surpass the competing. These findings highlight the accuracy of traffic pattern prediction. Consequently, this offers promising prospects for enhancing transportation management systems and urban infrastructure planning.Keywords
Cite This Article
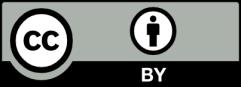
This work is licensed under a Creative Commons Attribution 4.0 International License , which permits unrestricted use, distribution, and reproduction in any medium, provided the original work is properly cited.