Open Access
ARTICLE
Olive Leaf Disease Detection via Wavelet Transform and Feature Fusion of Pre-Trained Deep Learning Models
1 Department of Information Systems, College of Computer and Information Sciences, Jouf University, Sakakah, Kingdom of Saudi Arabia
2 Department of Information Systems and Technology, Faculty of Graduate Studies and Research, Cairo University, Giza, Egypt
* Corresponding Author: Mahmood A. Mahmood. Email:
(This article belongs to the Special Issue: Deep Learning in Computer-Aided Diagnosis Based on Medical Image)
Computers, Materials & Continua 2024, 78(3), 3431-3448. https://doi.org/10.32604/cmc.2024.047604
Received 10 November 2023; Accepted 27 December 2023; Issue published 26 March 2024
Abstract
Olive trees are susceptible to a variety of diseases that can cause significant crop damage and economic losses. Early detection of these diseases is essential for effective management. We propose a novel transformed wavelet, feature-fused, pre-trained deep learning model for detecting olive leaf diseases. The proposed model combines wavelet transforms with pre-trained deep-learning models to extract discriminative features from olive leaf images. The model has four main phases: preprocessing using data augmentation, three-level wavelet transformation, learning using pre-trained deep learning models, and a fused deep learning model. In the preprocessing phase, the image dataset is augmented using techniques such as resizing, rescaling, flipping, rotation, zooming, and contrasting. In wavelet transformation, the augmented images are decomposed into three frequency levels. Three pre-trained deep learning models, EfficientNet-B7, DenseNet-201, and ResNet-152-V2, are used in the learning phase. The models were trained using the approximate images of the third-level sub-band of the wavelet transform. In the fused phase, the fused model consists of a merge layer, three dense layers, and two dropout layers. The proposed model was evaluated using a dataset of images of healthy and infected olive leaves. It achieved an accuracy of 99.72% in the diagnosis of olive leaf diseases, which exceeds the accuracy of other methods reported in the literature. This finding suggests that our proposed method is a promising tool for the early detection of olive leaf diseases.Keywords
Cite This Article
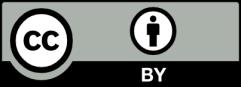
This work is licensed under a Creative Commons Attribution 4.0 International License , which permits unrestricted use, distribution, and reproduction in any medium, provided the original work is properly cited.