Open Access
ARTICLE
Nonparametric Statistical Feature Scaling Based Quadratic Regressive Convolution Deep Neural Network for Software Fault Prediction
School of Computer Science Engineering and Information Systems, Vellore Institute of Technology, Vellore, 632014, India
* Corresponding Author: Venkatesh Palanisamy. Email:
Computers, Materials & Continua 2024, 78(3), 3469-3487. https://doi.org/10.32604/cmc.2024.047407
Received 04 November 2023; Accepted 08 January 2024; Issue published 26 March 2024
Abstract
The development of defect prediction plays a significant role in improving software quality. Such predictions are used to identify defective modules before the testing and to minimize the time and cost. The software with defects negatively impacts operational costs and finally affects customer satisfaction. Numerous approaches exist to predict software defects. However, the timely and accurate software bugs are the major challenging issues. To improve the timely and accurate software defect prediction, a novel technique called Nonparametric Statistical feature scaled QuAdratic regressive convolution Deep nEural Network (SQADEN) is introduced. The proposed SQADEN technique mainly includes two major processes namely metric or feature selection and classification. First, the SQADEN uses the nonparametric statistical Torgerson–Gower scaling technique for identifying the relevant software metrics by measuring the similarity using the dice coefficient. The feature selection process is used to minimize the time complexity of software fault prediction. With the selected metrics, software fault perdition with the help of the Quadratic Censored regressive convolution deep neural network-based classification. The deep learning classifier analyzes the training and testing samples using the contingency correlation coefficient. The softstep activation function is used to provide the final fault prediction results. To minimize the error, the Nelder–Mead method is applied to solve non-linear least-squares problems. Finally, accurate classification results with a minimum error are obtained at the output layer. Experimental evaluation is carried out with different quantitative metrics such as accuracy, precision, recall, F-measure, and time complexity. The analyzed results demonstrate the superior performance of our proposed SQADEN technique with maximum accuracy, sensitivity and specificity by 3%, 3%, 2% and 3% and minimum time and space by 13% and 15% when compared with the two state-of-the-art methods.Keywords
Cite This Article
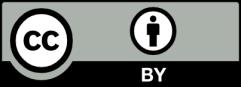