Open Access
ARTICLE
Multimodality Medical Image Fusion Based on Pixel Significance with Edge-Preserving Processing for Clinical Applications
1 Department of ECE and UCRD, Chandigarh University, Mohali, Punjab, 140413, India
2 Chitkara University Institute of Engineering and Technology, Chitkara University, Punjab, 140401, India
3 Department of ECE, Uttaranchal Institute of Technology, Uttaranchal University, Dehradun, 248007, India
4 IES College of Technology, IES University, Bhopal, 462044, India
5 College of Technical Engineering, The Islamic University, Najaf, 54001, Iraq
6 Department of Electrical Engineering, University of North Florida, Jacksonville, FL, 32224, USA
* Corresponding Author: Manob Jyoti Saikia. Email:
Computers, Materials & Continua 2024, 78(3), 4317-4342. https://doi.org/10.32604/cmc.2024.047256
Received 31 October 2023; Accepted 11 January 2024; Issue published 26 March 2024
Abstract
Multimodal medical image fusion has attained immense popularity in recent years due to its robust technology for clinical diagnosis. It fuses multiple images into a single image to improve the quality of images by retaining significant information and aiding diagnostic practitioners in diagnosing and treating many diseases. However, recent image fusion techniques have encountered several challenges, including fusion artifacts, algorithm complexity, and high computing costs. To solve these problems, this study presents a novel medical image fusion strategy by combining the benefits of pixel significance with edge-preserving processing to achieve the best fusion performance. First, the method employs a cross-bilateral filter (CBF) that utilizes one image to determine the kernel and the other for filtering, and vice versa, by considering both geometric closeness and the gray-level similarities of neighboring pixels of the images without smoothing edges. The outputs of CBF are then subtracted from the original images to obtain detailed images. It further proposes to use edge-preserving processing that combines linear lowpass filtering with a non-linear technique that enables the selection of relevant regions in detailed images while maintaining structural properties. These regions are selected using morphologically processed linear filter residuals to identify the significant regions with high-amplitude edges and adequate size. The outputs of low-pass filtering are fused with meaningfully restored regions to reconstruct the original shape of the edges. In addition, weight computations are performed using these reconstructed images, and these weights are then fused with the original input images to produce a final fusion result by estimating the strength of horizontal and vertical details. Numerous standard quality evaluation metrics with complementary properties are used for comparison with existing, well-known algorithms objectively to validate the fusion results. Experimental results from the proposed research article exhibit superior performance compared to other competing techniques in the case of both qualitative and quantitative evaluation. In addition, the proposed method advocates less computational complexity and execution time while improving diagnostic computing accuracy. Nevertheless, due to the lower complexity of the fusion algorithm, the efficiency of fusion methods is high in practical applications. The results reveal that the proposed method exceeds the latest state-of-the-art methods in terms of providing detailed information, edge contour, and overall contrast.Keywords
Cite This Article
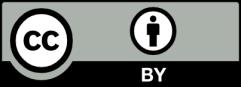
This work is licensed under a Creative Commons Attribution 4.0 International License , which permits unrestricted use, distribution, and reproduction in any medium, provided the original work is properly cited.