Open Access
REVIEW
A Review of Computing with Spiking Neural Networks
College of Electronic Science and Technology, National University of Defense Technology, Changsha, 410073, China
* Corresponding Authors: Yinan Wang. Email: ; Zhiwei Li. Email:
Computers, Materials & Continua 2024, 78(3), 2909-2939. https://doi.org/10.32604/cmc.2024.047240
Received 30 October 2023; Accepted 19 January 2024; Issue published 26 March 2024
Abstract
Artificial neural networks (ANNs) have led to landmark changes in many fields, but they still differ significantly from the mechanisms of real biological neural networks and face problems such as high computing costs, excessive computing power, and so on. Spiking neural networks (SNNs) provide a new approach combined with brain-like science to improve the computational energy efficiency, computational architecture, and biological credibility of current deep learning applications. In the early stage of development, its poor performance hindered the application of SNNs in real-world scenarios. In recent years, SNNs have made great progress in computational performance and practicability compared with the earlier research results, and are continuously producing significant results. Although there are already many pieces of literature on SNNs, there is still a lack of comprehensive review on SNNs from the perspective of improving performance and practicality as well as incorporating the latest research results. Starting from this issue, this paper elaborates on SNNs along the complete usage process of SNNs including network construction, data processing, model training, development, and deployment, aiming to provide more comprehensive and practical guidance to promote the development of SNNs. Therefore, the connotation and development status of SNN computing is reviewed systematically and comprehensively from four aspects: composition structure, data set, learning algorithm, software/hardware development platform. Then the development characteristics of SNNs in intelligent computing are summarized, the current challenges of SNNs are discussed and the future development directions are also prospected. Our research shows that in the fields of machine learning and intelligent computing, SNNs have comparable network scale and performance to ANNs and the ability to challenge large datasets and a variety of tasks. The advantages of SNNs over ANNs in terms of energy efficiency and spatial-temporal data processing have been more fully exploited. And the development of programming and deployment tools has lowered the threshold for the use of SNNs. SNNs show a broad development prospect for brain-like computing.Keywords
Cite This Article
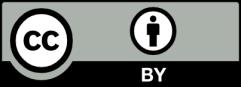