Open Access
ARTICLE
Identification of Software Bugs by Analyzing Natural Language-Based Requirements Using Optimized Deep Learning Features
1 Department of Computer Science and Engineering and International Bachelor’s Program in Informatics, Yuan Ze University, Zhongli, Taoyuan, 320315, Taiwan
2 Department of Computer Science, National University of Pakistan, Rawalpindi, Punjab, 46000, Pakistan
3 Department of Computer Software Engineering, MCS, National University of Sciences and Technology, Islamabad, 44000, Pakistan
4 Department of Computer Engineering, College of Computer and Information Sciences, King Saud University, P. O. Box 51178, Riyadh, 11543, Saudi Arabia
5 Department of Computer Science, School of Physics, Engineering and Computer Science, University of Hertfordshire, Hatfield, AL10 9AB, UK
6 Department of Computer Engineering, College of Computing and Informatics, University of Sharjah, Sharjah, 27272, United Arab Emirates
7 Department of AI and Software, Gachon University, Seongnam-SI, 13120, South Korea
* Corresponding Author: Muhammad Shahid Anwar. Email:
(This article belongs to the Special Issue: Requirements Engineering: Bridging Theory, Research and Practice)
Computers, Materials & Continua 2024, 78(3), 4379-4397. https://doi.org/10.32604/cmc.2024.047172
Received 27 October 2023; Accepted 11 December 2023; Issue published 26 March 2024
Abstract
Software project outcomes heavily depend on natural language requirements, often causing diverse interpretations and issues like ambiguities and incomplete or faulty requirements. Researchers are exploring machine learning to predict software bugs, but a more precise and general approach is needed. Accurate bug prediction is crucial for software evolution and user training, prompting an investigation into deep and ensemble learning methods. However, these studies are not generalized and efficient when extended to other datasets. Therefore, this paper proposed a hybrid approach combining multiple techniques to explore their effectiveness on bug identification problems. The methods involved feature selection, which is used to reduce the dimensionality and redundancy of features and select only the relevant ones; transfer learning is used to train and test the model on different datasets to analyze how much of the learning is passed to other datasets, and ensemble method is utilized to explore the increase in performance upon combining multiple classifiers in a model. Four National Aeronautics and Space Administration (NASA) and four Promise datasets are used in the study, showing an increase in the model’s performance by providing better Area Under the Receiver Operating Characteristic Curve (AUC-ROC) values when different classifiers were combined. It reveals that using an amalgam of techniques such as those used in this study, feature selection, transfer learning, and ensemble methods prove helpful in optimizing the software bug prediction models and providing high-performing, useful end mode.Keywords
Cite This Article
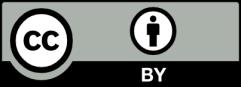
This work is licensed under a Creative Commons Attribution 4.0 International License , which permits unrestricted use, distribution, and reproduction in any medium, provided the original work is properly cited.