Open Access
ARTICLE
Adaptation of Federated Explainable Artificial Intelligence for Efficient and Secure E-Healthcare Systems
1 Department of Computer Science, Kinnaird College for Women, Lahore, Pakistan
2 College of Science and Engineering, University of Derby, Derby, DE221GB, UK
3 Department of Computer Science, College of Computer, Qassim University, Buraydah, 51452, Saudi Arabia
4 Department of Information Technology, College of Computer, Qassim University, Buraydah, 51452, Saudi Arabia
5 Department of Information Technology, College of Computer and Information Sciences, Princess Nourah bint Abdulrahman University, Riyadh, 84428, Saudi Arabia
6 STI Laboratory, IDMS Team, Faculty of Sciences and Techniques, Moulay Ismail University of Meknès, Meknes, Morocco
* Corresponding Author: Abdulatif Alabdulatif. Email:
Computers, Materials & Continua 2024, 78(3), 3413-3429. https://doi.org/10.32604/cmc.2024.046880
Received 18 October 2023; Accepted 05 January 2024; Issue published 26 March 2024
Abstract
Explainable Artificial Intelligence (XAI) has an advanced feature to enhance the decision-making feature and improve the rule-based technique by using more advanced Machine Learning (ML) and Deep Learning (DL) based algorithms. In this paper, we chose e-healthcare systems for efficient decision-making and data classification, especially in data security, data handling, diagnostics, laboratories, and decision-making. Federated Machine Learning (FML) is a new and advanced technology that helps to maintain privacy for Personal Health Records (PHR) and handle a large amount of medical data effectively. In this context, XAI, along with FML, increases efficiency and improves the security of e-healthcare systems. The experiments show efficient system performance by implementing a federated averaging algorithm on an open-source Federated Learning (FL) platform. The experimental evaluation demonstrates the accuracy rate by taking epochs size 5, batch size 16, and the number of clients 5, which shows a higher accuracy rate (19, 104). We conclude the paper by discussing the existing gaps and future work in an e-healthcare system.Keywords
Cite This Article
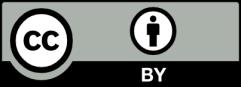
This work is licensed under a Creative Commons Attribution 4.0 International License , which permits unrestricted use, distribution, and reproduction in any medium, provided the original work is properly cited.