Open Access
ARTICLE
Audio-Text Multimodal Speech Recognition via Dual-Tower Architecture for Mandarin Air Traffic Control Communications
1 School of Computer Science and Software Engineering, University of Science and Technology Liaoning, Anshan, 114051, China
2 Institute of Applied Artificial Intelligence of the Guangdong-Hong Kong-Macao Greater Bay Area, Shenzhen Polytechnic University, Shenzhen, 518055, China
3 Shenzhen Institutes of Advanced Technology, Chinese Academy of Sciences, Shenzhen, 518055, China
4 Industrial Training Centre, Shenzhen Polytechnic University, Shenzhen, 518055, China
* Corresponding Author: Jin Ren. Email:
Computers, Materials & Continua 2024, 78(3), 3215-3245. https://doi.org/10.32604/cmc.2023.046746
Received 13 October 2023; Accepted 18 December 2023; Issue published 26 March 2024
Abstract
In air traffic control communications (ATCC), misunderstandings between pilots and controllers could result in fatal aviation accidents. Fortunately, advanced automatic speech recognition technology has emerged as a promising means of preventing miscommunications and enhancing aviation safety. However, most existing speech recognition methods merely incorporate external language models on the decoder side, leading to insufficient semantic alignment between speech and text modalities during the encoding phase. Furthermore, it is challenging to model acoustic context dependencies over long distances due to the longer speech sequences than text, especially for the extended ATCC data. To address these issues, we propose a speech-text multimodal dual-tower architecture for speech recognition. It employs cross-modal interactions to achieve close semantic alignment during the encoding stage and strengthen its capabilities in modeling auditory long-distance context dependencies. In addition, a two-stage training strategy is elaborately devised to derive semantics-aware acoustic representations effectively. The first stage focuses on pre-training the speech-text multimodal encoding module to enhance inter-modal semantic alignment and aural long-distance context dependencies. The second stage fine-tunes the entire network to bridge the input modality variation gap between the training and inference phases and boost generalization performance. Extensive experiments demonstrate the effectiveness of the proposed speech-text multimodal speech recognition method on the ATCC and AISHELL-1 datasets. It reduces the character error rate to 6.54% and 8.73%, respectively, and exhibits substantial performance gains of 28.76% and 23.82% compared with the best baseline model. The case studies indicate that the obtained semantics-aware acoustic representations aid in accurately recognizing terms with similar pronunciations but distinctive semantics. The research provides a novel modeling paradigm for semantics-aware speech recognition in air traffic control communications, which could contribute to the advancement of intelligent and efficient aviation safety management.Keywords
Cite This Article
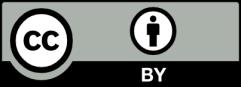
This work is licensed under a Creative Commons Attribution 4.0 International License , which permits unrestricted use, distribution, and reproduction in any medium, provided the original work is properly cited.