Open Access
ARTICLE
Prediction of Bandwidth of Metamaterial Antenna Using Pearson Kernel-Based Techniques
1 School of Computer Science and Engineering, Vellore Institute of Technology, Chennai, 600127, India
2 School of Electronics Engineering, Vellore Institute of Technology, Chennai, 600127, India
* Corresponding Author: Sherly Alphonse. Email:
Computers, Materials & Continua 2024, 78(3), 3449-3467. https://doi.org/10.32604/cmc.2024.046403
Received 29 September 2023; Accepted 11 December 2023; Issue published 26 March 2024
Abstract
The use of metamaterial enhances the performance of a specific class of antennas known as metamaterial antennas. The radiation cost and quality factor of the antenna are influenced by the size of the antenna. Metamaterial antennas allow for the circumvention of the bandwidth restriction for small antennas. Antenna parameters have recently been predicted using machine learning algorithms in existing literature. Machine learning can take the place of the manual process of experimenting to find the ideal simulated antenna parameters. The accuracy of the prediction will be primarily dependent on the model that is used. In this paper, a novel method for forecasting the bandwidth of the metamaterial antenna is proposed, based on using the Pearson Kernel as a standard kernel. Along with these new approaches, this paper suggests a unique hypersphere-based normalization to normalize the values of the dataset attributes and a dimensionality reduction method based on the Pearson kernel to reduce the dimension. A novel algorithm for optimizing the parameters of Convolutional Neural Network (CNN) based on improved Bat Algorithm-based Optimization with Pearson Mutation (BAO-PM) is also presented in this work. The prediction results of the proposed work are better when compared to the existing models in the literature.Keywords
Cite This Article
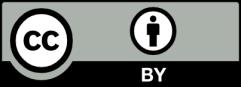