Open Access
ARTICLE
CL2ES-KDBC: A Novel Covariance Embedded Selection Based on Kernel Distributed Bayes Classifier for Detection of Cyber-Attacks in IoT Systems
Computer Science Department, College of Computer and Information Sciences, Imam Mohammad Ibn Saud Islamic University (IMSIU), Riyadh, 11432, Saudi Arabia
* Corresponding Author: P. Ganeshkumar. Email:
Computers, Materials & Continua 2024, 78(3), 3511-3528. https://doi.org/10.32604/cmc.2024.046396
Received 28 September 2023; Accepted 11 January 2024; Issue published 26 March 2024
Abstract
The Internet of Things (IoT) is a growing technology that allows the sharing of data with other devices across wireless networks. Specifically, IoT systems are vulnerable to cyberattacks due to its opennes The proposed work intends to implement a new security framework for detecting the most specific and harmful intrusions in IoT networks. In this framework, a Covariance Linear Learning Embedding Selection (CL2ES) methodology is used at first to extract the features highly associated with the IoT intrusions. Then, the Kernel Distributed Bayes Classifier (KDBC) is created to forecast attacks based on the probability distribution value precisely. In addition, a unique Mongolian Gazellas Optimization (MGO) algorithm is used to optimize the weight value for the learning of the classifier. The effectiveness of the proposed CL2ES-KDBC framework has been assessed using several IoT cyber-attack datasets, The obtained results are then compared with current classification methods regarding accuracy (97%), precision (96.5%), and other factors. Computational analysis of the CL2ES-KDBC system on IoT intrusion datasets is performed, which provides valuable insight into its performance, efficiency, and suitability for securing IoT networks.Keywords
Cite This Article
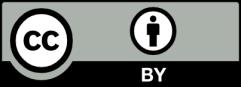
This work is licensed under a Creative Commons Attribution 4.0 International License , which permits unrestricted use, distribution, and reproduction in any medium, provided the original work is properly cited.