Open Access
ARTICLE
Nodule Detection Using Local Binary Pattern Features to Enhance Diagnostic Decisions
1 Faculty of Computer Science and Information Technology, The Superior University, Lahore, 54600, Pakistan
2 Intelligent Data Visual Computing Research (IDVCR), Lahore, 54600, Pakistan
3 Department of Computer Science, National University of Technology, Islamabad, 45000, Pakistan
4 Department of Radiological Sciences and Medical Imaging, College of Applied, Medical Sciences, Prince Sattam Bin Abdulaziz University, Al-Kharj, 16278, Saudi Arabia
5 Information Systems Department, College of Computer and Information Sciences, Imam Mohammad Ibn Saud Islamic University (IMSIU), Riyadh, 12571, Saudi Arabia
* Corresponding Author: Umar Rashid. Email:
(This article belongs to the Special Issue: Deep Learning in Medical Imaging-Disease Segmentation and Classification)
Computers, Materials & Continua 2024, 78(3), 3377-3390. https://doi.org/10.32604/cmc.2024.046320
Received 26 September 2023; Accepted 28 December 2023; Issue published 26 March 2024
Abstract
Pulmonary nodules are small, round, or oval-shaped growths on the lungs. They can be benign (noncancerous) or malignant (cancerous). The size of a nodule can range from a few millimeters to a few centimeters in diameter. Nodules may be found during a chest X-ray or other imaging test for an unrelated health problem. In the proposed methodology pulmonary nodules can be classified into three stages. Firstly, a 2D histogram thresholding technique is used to identify volume segmentation. An ant colony optimization algorithm is used to determine the optimal threshold value. Secondly, geometrical features such as lines, arcs, extended arcs, and ellipses are used to detect oval shapes. Thirdly, Histogram Oriented Surface Normal Vector (HOSNV) feature descriptors can be used to identify nodules of different sizes and shapes by using a scaled and rotation-invariant texture description. Smart nodule classification was performed with the XGBoost classifier. The results are tested and validated using the Lung Image Consortium Database (LICD). The proposed method has a sensitivity of 98.49% for nodules sized 3–30 mm.Keywords
Cite This Article
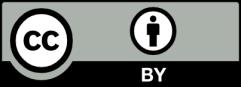