Open Access
ARTICLE
Boosting Adversarial Training with Learnable Distribution
1 School of Electronics and Information Engineering, Nanjing University of Information Science and Technology, Nanjing, 210044, China
2 Key Laboratory of Intelligent Support Technology for Complex Environments, Ministry of Education, Nanjing, 210044, China
3 School of Computer and Software, Nanjing University of Information Science and Technology, Nanjing, 210044, China
4 Nanjing Center for Applied Mathematics, Nanjing, 211135, China
* Corresponding Author: Guangjie Liu. Email:
Computers, Materials & Continua 2024, 78(3), 3247-3265. https://doi.org/10.32604/cmc.2024.046082
Received 18 September 2023; Accepted 25 December 2023; Issue published 26 March 2024
Abstract
In recent years, various adversarial defense methods have been proposed to improve the robustness of deep neural networks. Adversarial training is one of the most potent methods to defend against adversarial attacks. However, the difference in the feature space between natural and adversarial examples hinders the accuracy and robustness of the model in adversarial training. This paper proposes a learnable distribution adversarial training method, aiming to construct the same distribution for training data utilizing the Gaussian mixture model. The distribution centroid is built to classify samples and constrain the distribution of the sample features. The natural and adversarial examples are pushed to the same distribution centroid to improve the accuracy and robustness of the model. The proposed method generates adversarial examples to close the distribution gap between the natural and adversarial examples through an attack algorithm explicitly designed for adversarial training. This algorithm gradually increases the accuracy and robustness of the model by scaling perturbation. Finally, the proposed method outputs the predicted labels and the distance between the sample and the distribution centroid. The distribution characteristics of the samples can be utilized to detect adversarial cases that can potentially evade the model defense. The effectiveness of the proposed method is demonstrated through comprehensive experiments.Keywords
Cite This Article
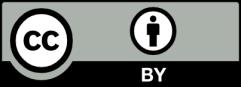
This work is licensed under a Creative Commons Attribution 4.0 International License , which permits unrestricted use, distribution, and reproduction in any medium, provided the original work is properly cited.