Open Access
ARTICLE
Restoration of the JPEG Maximum Lossy Compressed Face Images with Hourglass Block-GAN
1 Department of Computer AI Convergence Engineering, Kumoh National Institute of Technology, Gumi, 39177, Korea
2 Department of Computer Engineering, Kumoh National Institute of Technology, Gumi, 39177, Korea
* Corresponding Author: Sungyoung Kim. Email:
(This article belongs to the Special Issue: Advanced Artificial Intelligence and Machine Learning Frameworks for Signal and Image Processing Applications)
Computers, Materials & Continua 2024, 78(3), 2893-2908. https://doi.org/10.32604/cmc.2023.046081
Received 18 September 2023; Accepted 28 November 2023; Issue published 26 March 2024
Abstract
In the context of high compression rates applied to Joint Photographic Experts Group (JPEG) images through lossy compression techniques, image-blocking artifacts may manifest. This necessitates the restoration of the image to its original quality. The challenge lies in regenerating significantly compressed images into a state in which these become identifiable. Therefore, this study focuses on the restoration of JPEG images subjected to substantial degradation caused by maximum lossy compression using Generative Adversarial Networks (GAN). The generator in this network is based on the U-Net architecture. It features a new hourglass structure that preserves the characteristics of the deep layers. In addition, the network incorporates two loss functions to generate natural and high-quality images: Low Frequency (LF) loss and High Frequency (HF) loss. HF loss uses a pretrained VGG-16 network and is configured using a specific layer that best represents features. This can enhance the performance in the high-frequency region. In contrast, LF loss is used to handle the low-frequency region. The two loss functions facilitate the generation of images by the generator, which can mislead the discriminator while accurately generating high- and low-frequency regions. Consequently, by removing the blocking effects from maximum lossy compressed images, images in which identities could be recognized are generated. This study represents a significant improvement over previous research in terms of the image resolution performance.Keywords
Cite This Article
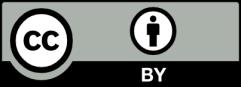
This work is licensed under a Creative Commons Attribution 4.0 International License , which permits unrestricted use, distribution, and reproduction in any medium, provided the original work is properly cited.