Open Access
ARTICLE
A Cover-Independent Deep Image Hiding Method Based on Domain Attention Mechanism
1 School of Computer and Software, Nanjing University of Information Science and Technology, Nanjing, 210044, China
2 School of Electronics and Information Engineering, Nanjing University of Information Science and Technology, Nanjing, 210044, China
* Corresponding Author: Xianyi Chen. Email:
Computers, Materials & Continua 2024, 78(3), 3001-3019. https://doi.org/10.32604/cmc.2023.045311
Received 23 August 2023; Accepted 21 November 2023; Issue published 26 March 2024
Abstract
Recently, deep image-hiding techniques have attracted considerable attention in covert communication and high-capacity information hiding. However, these approaches have some limitations. For example, a cover image lacks self-adaptability, information leakage, or weak concealment. To address these issues, this study proposes a universal and adaptable image-hiding method. First, a domain attention mechanism is designed by combining the Atrous convolution, which makes better use of the relationship between the secret image domain and the cover image domain. Second, to improve perceived human similarity, perceptual loss is incorporated into the training process. The experimental results are promising, with the proposed method achieving an average pixel discrepancy (APD) of 1.83 and a peak signal-to-noise ratio (PSNR) value of 40.72 dB between the cover and stego images, indicative of its high-quality output. Furthermore, the structural similarity index measure (SSIM) reaches 0.985 while the learned perceptual image patch similarity (LPIPS) remarkably registers at 0.0001. Moreover, self-testing and cross-experiments demonstrate the model’s adaptability and generalization in unknown hidden spaces, making it suitable for diverse computer vision tasks.Keywords
Cite This Article
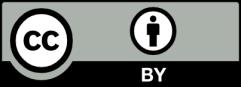
This work is licensed under a Creative Commons Attribution 4.0 International License , which permits unrestricted use, distribution, and reproduction in any medium, provided the original work is properly cited.