Open Access
REVIEW
A Systematic Literature Review of Machine Learning and Deep Learning Approaches for Spectral Image Classification in Agricultural Applications Using Aerial Photography
1 College of Computing and Information Sciences, Karachi Institute of Economics and Technology, Karachi, 75190, Pakistan
2 Faculty of Computing and Informatics (FCI), Multimedia University, Cyberjaya, 63100, Malaysia
3 Faculty of Computing, Riphah International University, Islamabad, 46000, Pakistan
4 Faculty of Engineering and Information Technology, School of Computer Science, University of Technology Sydney, Sydney, Australia
* Corresponding Authors: Muhammad Naveed. Email: ; Mazliham Mohd Su’ud. Email:
(This article belongs to the Special Issue: Machine Vision Detection and Intelligent Recognition)
Computers, Materials & Continua 2024, 78(3), 2967-3000. https://doi.org/10.32604/cmc.2024.045101
Received 17 August 2023; Accepted 10 November 2023; Issue published 26 March 2024
Abstract
Recently, there has been a notable surge of interest in scientific research regarding spectral images. The potential of these images to revolutionize the digital photography industry, like aerial photography through Unmanned Aerial Vehicles (UAVs), has captured considerable attention. One encouraging aspect is their combination with machine learning and deep learning algorithms, which have demonstrated remarkable outcomes in image classification. As a result of this powerful amalgamation, the adoption of spectral images has experienced exponential growth across various domains, with agriculture being one of the prominent beneficiaries. This paper presents an extensive survey encompassing multispectral and hyperspectral images, focusing on their applications for classification challenges in diverse agricultural areas, including plants, grains, fruits, and vegetables. By meticulously examining primary studies, we delve into the specific agricultural domains where multispectral and hyperspectral images have found practical use. Additionally, our attention is directed towards utilizing machine learning techniques for effectively classifying hyperspectral images within the agricultural context. The findings of our investigation reveal that deep learning and support vector machines have emerged as widely employed methods for hyperspectral image classification in agriculture. Nevertheless, we also shed light on the various issues and limitations of working with spectral images. This comprehensive analysis aims to provide valuable insights into the current state of spectral imaging in agriculture and its potential for future advancements.Keywords
Cite This Article
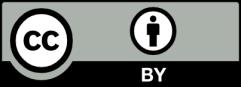