Open Access
ARTICLE
Enhanced Differentiable Architecture Search Based on Asymptotic Regularization
1 School of Computer Science and Technology, Harbin University of Science and Technology, Harbin, 150006, China
2 School of Automation, Harbin University of Science and Technology, Harbin, 150006, China
* Corresponding Author: Jinjie Huang. Email:
Computers, Materials & Continua 2024, 78(2), 1547-1568. https://doi.org/10.32604/cmc.2023.047489
Received 07 November 2023; Accepted 09 December 2023; Issue published 27 February 2024
Abstract
In differentiable search architecture search methods, a more efficient search space design can significantly improve the performance of the searched architecture, thus requiring people to carefully define the search space with different complexity according to various operations. Meanwhile rationalizing the search strategies to explore the well-defined search space will further improve the speed and efficiency of architecture search. With this in mind, we propose a faster and more efficient differentiable architecture search method, AllegroNAS. Firstly, we introduce a more efficient search space enriched by the introduction of two redefined convolution modules. Secondly, we utilize a more efficient architectural parameter regularization method, mitigating the overfitting problem during the search process and reducing the error brought about by gradient approximation. Meanwhile, we introduce a natural exponential cosine annealing method to make the learning rate of the neural network training process more suitable for the search procedure. Moreover, group convolution and data augmentation are employed to reduce the computational cost. Finally, through extensive experiments on several public datasets, we demonstrate that our method can more swiftly search for better-performing neural network architectures in a more efficient search space, thus validating the effectiveness of our approach.Keywords
Cite This Article
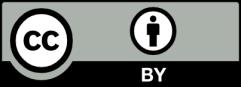
This work is licensed under a Creative Commons Attribution 4.0 International License , which permits unrestricted use, distribution, and reproduction in any medium, provided the original work is properly cited.