Open Access
ARTICLE
Unknown DDoS Attack Detection with Fuzzy C-Means Clustering and Spatial Location Constraint Prototype Loss
1 Department of Electronic Engineering, National Kaohsiung University of Science and Technology, Kaohsiung, 807618, Taiwan
2 Department of Electronic and Automation Engineering, Nha Trang University, Nha Trang, 650000, Vietnam
* Corresponding Authors: Mong-Fong Horng. Email: ; Chin-Shiuh Shieh. Email:
(This article belongs to the Special Issue: Cybersecurity for Cyber-attacks in Critical Applications in Industry)
Computers, Materials & Continua 2024, 78(2), 2181-2205. https://doi.org/10.32604/cmc.2024.047387
Received 04 November 2023; Accepted 25 December 2023; Issue published 27 February 2024
Abstract
Since its inception, the Internet has been rapidly evolving. With the advancement of science and technology and the explosive growth of the population, the demand for the Internet has been on the rise. Many applications in education, healthcare, entertainment, science, and more are being increasingly deployed based on the internet. Concurrently, malicious threats on the internet are on the rise as well. Distributed Denial of Service (DDoS) attacks are among the most common and dangerous threats on the internet today. The scale and complexity of DDoS attacks are constantly growing. Intrusion Detection Systems (IDS) have been deployed and have demonstrated their effectiveness in defense against those threats. In addition, the research of Machine Learning (ML) and Deep Learning (DL) in IDS has gained effective results and significant attention. However, one of the challenges when applying ML and DL techniques in intrusion detection is the identification of unknown attacks. These attacks, which are not encountered during the system’s training, can lead to misclassification with significant errors. In this research, we focused on addressing the issue of Unknown Attack Detection, combining two methods: Spatial Location Constraint Prototype Loss (SLCPL) and Fuzzy C-Means (FCM). With the proposed method, we achieved promising results compared to traditional methods. The proposed method demonstrates a very high accuracy of up to 99.8% with a low false positive rate for known attacks on the Intrusion Detection Evaluation Dataset (CICIDS2017) dataset. Particularly, the accuracy is also very high, reaching 99.7%, and the precision goes up to 99.9% for unknown DDoS attacks on the DDoS Evaluation Dataset (CICDDoS2019) dataset. The success of the proposed method is due to the combination of SLCPL, an advanced Open-Set Recognition (OSR) technique, and FCM, a traditional yet highly applicable clustering technique. This has yielded a novel method in the field of unknown attack detection. This further expands the trend of applying DL and ML techniques in the development of intrusion detection systems and cybersecurity. Finally, implementing the proposed method in real-world systems can enhance the security capabilities against increasingly complex threats on computer networks.Keywords
Cite This Article
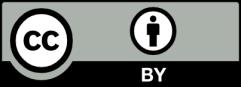