Open Access
REVIEW
A Review of the Application of Artificial Intelligence in Orthopedic Diseases
Department of Electrical Engineering, Guizhou University, Guiyang, China
* Corresponding Author: Xiao Wang. Email:
(This article belongs to the Special Issue: Deep Learning in Computer-Aided Diagnosis Based on Medical Image)
Computers, Materials & Continua 2024, 78(2), 2617-2665. https://doi.org/10.32604/cmc.2024.047377
Received 03 November 2023; Accepted 03 January 2024; Issue published 27 February 2024
Abstract
In recent years, Artificial Intelligence (AI) has revolutionized people’s lives. AI has long made breakthrough progress in the field of surgery. However, the research on the application of AI in orthopedics is still in the exploratory stage. The paper first introduces the background of AI and orthopedic diseases, addresses the shortcomings of traditional methods in the detection of fractures and orthopedic diseases, draws out the advantages of deep learning and machine learning in image detection, and reviews the latest results of deep learning and machine learning applied to orthopedic image detection in recent years, describing the contributions, strengths and weaknesses, and the direction of the future improvements that can be made in each study. Next, the paper also introduces the difficulties of traditional orthopedic surgery and the roles played by AI in preoperative, intraoperative, and postoperative orthopedic surgery, scientifically discussing the advantages and prospects of AI in orthopedic surgery. Finally, the article discusses the limitations of current research and technology in clinical applications, proposes solutions to the problems, and summarizes and outlines possible future research directions. The main objective of this review is to inform future research and development of AI in orthopedics.Keywords
Artificial Intelligence (AI) has been closely connected with human life in recent years, and people are affected by AI everywhere. The concept of AI was first proposed by Prof. John McCarthy in 1956. It involves “the science and engineering of making intelligent machines, outstanding computer programs” [1]. AI is a technology that enables computers, computer-controlled robots, or software to think like humans. AI utilizes research results to develop intelligent software and systems by studying how the human brain thinks and how humans solve problems, learn, make decisions, and work. AI has many branches, with this study focusing specifically on Machine Learning (ML). While other branches of AI have significantly impacted science and technology, machine learning is undoubtedly the most researched direction in medical research applications today. ML allows machines to automatically learn and improve from experience without requiring human programming to specify rules and logic. Fig. 1 shows the components and primary models of machine learning. ML usually requires manual extraction of features, a process known as feature engineering.
Figure 1: Components and main models of machine learning
In most cases, feature engineering can be done efficiently; however, it is very challenging to be helpful in image detection. We want machines to automatically learn features from image data, thus reducing human workload. McCulloch et al. [2] were inspired by biology. They proposed a one-of-a-kind network similar to a biological neural network, miming its structure, implementation mechanism, and function. A network of artificial neurons typically consists of several layers containing an input layer, one or more hidden layers, and an output layer. In the hidden layers, researchers can refine the training by adjusting the neural network’s structure and the neuron nodes’ weights. Artificial Neural Networks (ANNs) allow computational models with multiple processing layers to learn multiple levels of abstract data representation. With the backpropagation algorithm, the model can indicate how to change the internal structure to find complex structures in large datasets. The principle of Artificial Neural Network (ANN) is shown in Fig. 2. Deep learning is a branch of machine learning that centers on ANNs. Deep Learning (DL) is far more effective than previous related techniques in speech and image recognition [3]. Unlike general ML, DL automatically learns high-dimensional abstractions of data through neural networks, reducing the composition of feature engineering and placing more emphasis on model structure, nonlinear processing, feature extraction, and feature transformation. The relationship between AI, ML, and DL is shown in Fig. 3.
Figure 2: Deep neural network
Figure 3: Relationship between artificial intelligence, machine learning and deep learning
At this stage, as medical data metrics continue to grow and the volume of data increases dramatically, there is an urgent need for robust data processing capabilities to support the medical field. Today, medical imaging is growing at an annual rate of up to 30%. While the demand for medical imaging is slowing down, it is still much higher than the 1.8% annual growth rate for radiologists. The growth in medical imaging demand far exceeds the physicians’ supply [4]. Today’s AI is integrated into people’s daily healthcare with investments of over $1.5 billion and growing [5]. The application of AI in medical imaging can help doctors make quick and accurate diagnoses and develop the proper medical treatment plan, which meets the current needs and has considerable potential in the coming years.
The field of orthopedics encompasses a wide range of conditions such as joint replacement, spinal correction, traumatic orthopedics, cartilage, and osteoarthritis, all of which require rigorous patient planning, including patient characterization, preoperative evaluation, intraoperative assistance, and postoperative rehabilitation. Personalized treatment can significantly improve diagnostic and surgical accuracy and medical efficiency. In medical fields such as radiology, dermatology, and cardiology, AI has already succeeded, surpassing senior experts in some areas. Cabitza et al. [6] report a 10-fold increase in machine-learning-related articles in the orthopedic literature since 2010. However, by deeply analyzing the relevant literature in this field in recent years, we found that applying relevant research in orthopedics to the clinic is still very challenging, and the application of AI in orthopedics is still in the developmental stage [7]. Although OsteoDetect, an AI based on deep learning, has been approved by the U.S. Food and Drug Administration (FDA) in 2018 for the detection and assisted diagnosis of wrist fractures in adults and has achieved good results, it is still deficient in penetration and clinical application. Therefore, applying AI to orthopedic diseases is still a pressing issue for current researchers. The purpose of this review is to provide an understanding of the concept of AI based on the analysis of a large number of studies in the field of orthopedics, to summarize the recent studies of AI in orthopedics in the last few years with the related improved methods, and to analyze the current limitations and the future development direction.
2 Advances in the Application of Artificial Intelligence to Orthopedic Image Detection
The diagnosis of many orthopedic diseases often relies on image judgment. However, there are many types of orthopedic diseases, and the similarity of different diseases is exceptionally high, which often causes young physicians to misdiagnose. Missed diagnosis in determining a patient’s illness will not only waste medical resources but also affect further patient treatment and aggravate the deterioration of the patient’s condition.
DL image processing techniques are categorized into image classification, target recognition, and semantic segmentation. Image classification techniques are mainly used to distinguish disease categories, while target recognition and semantic segmentation are used for lesion detection. Introducing deep learning into medical image detection, utilizing its powerful learning ability and excellent image segmentation ability, makes up for the shortcomings of traditional medical image recognition, such as slow speed and poor recognition effect, that makes the medical image obtain essential information and realizes more accurate classification, recognition and segmentation [8].
2.1 Deep Learning Orthopedic Image Detection Fundamentals
2.1.1 Deep Learning Orthopedic Image Detection Process
Orthopedic image detection based on deep learning consists of two main parts: training aims to use the training set for parameter learning of the detection network, and the process of the training phase mainly includes data preprocessing, detection network, and label matching and loss calculation, and finally generates data such as evaluation indexes. Testing aims to detect the model’s generalization ability, which determines whether the model can be used in the clinic. The testing phase mainly consists of input images, detection network, post-processing, and finally, the generated detection results. The deep learning image detection training process is shown in Fig. 4. The scoliosis image and detection results are shown in Fig. 5.
Figure 4: Deep learning image detection training process
Figure 5: Spinal curvature image testing process and results
2.1.2 Model Evaluation Metrics
Table 1 lists the commonly used evaluation metrics for image detection. The metrics can most intuitively reflect the model’s performance and the direction of future improvement.
The four indicators, True positives (TP), False positives (FP), False negatives (FN), and True negatives (TN), are presented together in the image as the corresponding confusion matrix. The confusion matrix structure is shown in Fig. 6. The model’s performance is better when the positions corresponding to the observations are more in quadrants two and four, and the model’s performance is worse when they are more in quadrants one and three.
Figure 6: Confusion matrix
2.1.3 Commonly Used Deep Learning Models
You Only Look Once-V5 (YOLOv5) is the most commonly used deep learning model for orthopedic image recognition. As a real-time detection model, its detection speed and accuracy have been validated on several validation sets with reasonable accuracy. Compared with the previous generation model YOLOv4, the new generation model YOLOv5 has improved in model resource consumption, detection speed and accuracy, and page design. Although YOLOv5 is not the latest achievement of the YOLO series, its balanced recognition speed and accuracy make it widely used. The frame and performance variations of the YOLO series are shown in Table 2. The structure of YOLOv5 is shown in Fig. 7.
Figure 7: YOLOv5 network architecture
The Focus and Cryptographic Service Provider (CSP) structures proposed by YOLOv5 can improve the receptive field and alleviate problems such as gradient disappearance. However, the model still has some defects, such as image transformation, occlusion, light change, and other indicators that need improvement in complex situations. Because YOLOv5 is a single-stage target detection model, it still faces challenges in the face of orthopedic detection and other small target detection tasks. The current applications of the improved YOLOv5 in medicine are shown in Table 3. At this stage, improvements to YOLOv5 can be made in the following ways. For example, (1) Introduce a multi-task learning mechanism to solve multiple related tasks simultaneously that improves the comprehensive performance of the model. (2) Effectively fuse multi-scale features to improve the detection accuracy of large and small targets. (3) Utilize only weakly supervised signals for detection to reduce the workload of data annotation, thus improving the model’s Robustness.
UNet was proposed by Olaf Ronneberger et al. in 2015 [18] and is now widely used in medical image segmentation due to its good segmentation accuracy. The network is U-shaped and adopts an entirely symmetric left-right structure. The left side consists of convolutional downsampling and MaxPooling, referred to as the contraction path in the original paper. The right side consists of four blocks, each feature-reduced by upsampling. This approach reduces the feature mapping of the output of the compression path and makes it consistent with the input image, referred to as the extension path in the original paper. The difference between UNet and the traditional segmentation model Fully Convolutional Networks (FCN) for Semantic Segmentation is that the deep and shallow information in FCN is achieved by adding the corresponding pixels. In contrast, UNet is achieved by splicing, which retains more positional information and allows deeper layers to choose freely between shallow UNet, which is more commonly used in the medical image field and requires high semantic segmentation accuracy. The structure of UNet is shown in Fig. 8.
Figure 8: UNet network architecture
In the deep information, the low-resolution information, after many times of downsampling, can provide the semantic information of the segmentation target in the context of the whole image. In the shallow information, the high-resolution information directly transferred from the encoder to the decoder after the concatenate operation can provide finer features for segmentation. However, because of the compacting path when superimposed on the convolution and pooling operation to gradually reduce the resolution of the feature map, this approach will introduce many parameters, reducing the model’s efficiency. Downsampling will continue to lose spatial information, affecting the final segmentation effect, and up-sampling involves spatial information restoration, which is only possible to achieve by considering the global information. The current stage of the improvement method on UNet is shown in Table 4. The following strategies can be used as future research directions: 1) Construct a global aggregation module to aggregate global information without deepening the structure of the contracting path, which can effectively solve the information loss problem in the up-sampling process. 2) Introducing an attention mechanism or a lightweight network can solve the problem of many UNet parameters and high computation and ensure the model is manageable.
Mask region-based convolutional neural network (R-CNN) was proposed by He et al. [34] in 2017; based on the previous generation of Faster-RCNN, a fully connected segmentation network was added on top of the leading feature network, and the original two tasks (classification regression) was changed to three tasks (classification, regression, and segmentation). Table 5 lists the AP performance of Faster R-CNN and Mask R-CNN with the MS COCO dataset. By comparing the various indexes, it is easy to find that Mask R-CNN is better than Faster R-CNN in all aspects, and the good semantic segmentation and target recognition accuracy of Mask R-CNN makes it favored by medical image researchers. The structure of Mask R-CNN is shown in Fig. 9.
Figure 9: Mask R-CNN network architecture
The structure of Mask R-CNN is rigorous, in which the Feature Pyramid Network (FPN) network adopts the hopping level connection similar to that in the UNet network, which enhances the ability of multiscale representation and uses the feature pyramid structure to integrate the multiscale information. Then, the high-quality proposal obtained by the prediction of the Region Proposal Network (RPN) network is transmitted to the Region of Interest (ROI) Head structure. Finally, the input feature map is predicted by the FCN layer to get the final mask result. In the year when Mask R-CNN was proposed, it not only won the best paper of ICCV2017 but also achieved better results than the existing model algorithms in the three challenges of the COCO dataset. However, facing orthopedic images, which are mainly segmented by small-sample target instances and lack pixel-level annotation, the performance index of Mask R-CNN still cannot meet the clinical use requirements. The current stage of the improvement method on Mask R-CNN is shown in Table 6. The following methods could be future improvements: 1) When orthopedic images lack pixel-level labeling, we can consider designing new loss functions or developing active learning methods to reduce the time of manual labeling. 2) When faced with small-sample instance segmentation, we can consider designing new data augmentation methods and introducing migration learning and generative adversarial networks to solve the small-sample segmentation problem.
Google proposed a Transformer in 2017 [45]. Transformer has been an emerging structure in recent years; the main difference with traditional Recurrent Neural Network (RNN) and Convolutional Neural Networks (CNN) models is that instead of recursive and convolutional structures, it is entirely based on the attention mechanism and residual connections. The attentional mechanisms contained in the structure have been used with great success in natural language processing (NLP) tasks. OpenAI proposed a series of powerful pre-trained language models, Generative Pre-trained Transformer (GPT), based on Transformer, which achieved excellent metrics in article generation and machine translation NLP tasks. Subsequently, researchers in the field of computer vision ported and applied them to many vision tasks with good results. Its primary applications and time are shown in Table 7. The structure of the Transformer is shown in Fig. 10.
Figure 10: Transformer network architecture
The structure of the transformer is as follows: firstly, position coding is added to the input variables; the encoder block performs a matrix transformation on the sequence data to obtain Q, K, and V and then calculates the Attention value; the add module contains the residual block, which serves to prevent degradation in the training of the deep neural network, and the Normalize which serves to speed up the training while improving the stability. The fully connected layer is then a two-layer neural network that first linearly transforms the features, then activates the function by Relu, and finally linearly transforms it so that its dimensionality is unchanged. After repeating the addition and normalization process, the data is fed into the decoder block, which has the same functionality as the encoder block. However, the multi-head attention has an additional masking structure that masks specific values so they do not affect when the parameters are updated. After the data is linearly transformed, Softmax obtains the probability distribution of the output, and finally, the predicted output is obtained.
Transformer has the following advantages over other models: 1. It achieves parallel computation, solves the problem that traditional models can only be computed serially, and realizes parallelization between training samples. 2. It solves the problem of long-term dependence, has a global feeling field, and pays attention to the global information. Self-attention requires only one step of matrix computation to complete the correlation between sequences and does not need to be passed through hidden layers like CNN. 3. It has strong multimodal fusion ability and better robustness. It needs to pass through the hidden layer as CNN does. 4. It has strong multimodal fusion ability and better robustness. It can achieve better results when dealing with images, audio, and this paper, and it also has specific adaptability in the face of noise or abnormal data. 5. It can deal with complex models and data better. Self-attention can globally model the connection between pixels in different locations, thus capturing the information in the image more effectively. However, Transformer still has the following problems when facing images like orthopedics, which are composed of a small number of datasets and are more concerned with fine-grained features: 1) High computational complexity. Dealing with multi-scale complex images will occupy too many resources, and the number of model parameters is large. 2) Insufficient ability to extract detailed features. As self-attention focuses more on global information, it reduces the ability to extract low-resolution images. 3) Loss of location information. Orthopedic images contain a large amount of spatial information at the pixel level, and the Transformer model cannot take advantage of the order in the sequence, which leads to the loss of positional information, and is not sensitive to fine-grained features, which may lead to weak positioning of the boundary capability.
The current improvements of Transformer in medical applications are shown in Table 8. Future research on Transformer can be carried out in the following areas: 1) Accelerated convergence. Use nested dense hopping connections in the backbone network and incorporate residual networks 2) Reduce the number of parameters to reduce computational complexity. It reduces computation effectively by encoding spatial and channel dimension information with shared weights. Also, consider adding a whole convolutional layer to enhance the linear projection. 3) Improve the extraction of detailed features by adding convolutional blocks to enhance the local spatial information capability.
2.1.4 Commonly Used Public Datasets in Orthopedics
Deep learning models usually require large amounts of data to avoid overfitting due to too many parameters. There are not many orthopedic datasets that can be made public due to reasons such as patient privacy and relevant laws. Therefore, in addition to using publicly available datasets to train the model, researchers should also use some internal datasets to test the model to ensure the accuracy of the results. Researchers can also extend the datasets by employing data preprocessing. Commonly used public datasets in orthopedics and their download addresses are shown in Table 9.
2.2 Artificial Intelligence-Assisted Fracture Detection
Traditional methods of fracture diagnosis include radiographs, computed tomography (CT), and magnetic resonance imaging (MRI). However, it is often difficult for radiologists to quickly and accurately determine the fracture site due to tiny fractures, different irradiation locations, and occult fractures. Using deep learning for fracture detection can effectively eliminate the interference of human subjective factors and shorten the diagnosis time while improving the accuracy rate [52].
Traditional rib fracture detection methods use CT images. However, due to the particular anatomical morphology of the rib cage, it takes much time for physicians to recognize traditional CT images. Zhou et al. [53] applied a CNN in rib fracture detection and classification, and the results showed that with the assistance of AI, the physician’s accuracy, sensitivity, and diagnosis time were all improved, which demonstrates the feasibility of CNN models for rib fracture diagnosis. However, the model used in this study needed to achieve satisfactory levels of accuracy and recall. Akifumi et al. [54] also developed a computer-aided diagnostic system utilizing deep learning, which was used for rib fracture detection. The model is based on a three-dimensional (3-D) object detection network in a two-stage object detection framework that utilizes 3-D convolution to maximize the extraction of image features. The results show that the algorithm is sensitive enough to quickly detect rib fractures in CT images. Liang et al. [55] developed a deep learning model called FracNet to detect and segment rib fractures using a 900-sheet dataset and showed a sensitivity of 92.9% and a Dice coefficient of 71.5%. The proposed model improves the sensitivity of rib fracture detection and significantly reduces clinical time consumption. However, these studies only realized the dichotomous classification of fracture detection and needed to provide a more detailed classification of the fracture degree, which still has limitations in practical applications. Yang et al. [56] used a convolutional neural network to diagnose rib fractures. They compared the diagnostic efficiency of a deep learning system with that of a radiologist and developed a discriminative model for fractures of different degrees. The system can accurately and quickly diagnose and categorize rib fractures, significantly saving medical resources. The specific indicators of the above study are shown in Table 10.
Traditionally, diagnosing thoracolumbar vertebral fractures (VF) in clinical testing is often applied together with imaging analysis, such as CT or MRI. However, this method is time-consuming and needs to improve detection accuracy. Kazuma et al. [57] applied deep convolutional neural networks (DCNN) to the diagnosis of VF for the first time, using images from 300 different patients to train the DCNN and evaluate the diagnostic accuracy, sensitivity, and specificity of the model at the same time with orthopedic residents, orthopedic surgeons, and spine surgeons. The results showed that DCNN outperformed orthopedic surgeons in all performance metrics. However, DCNN still needs to be improved to increase detection accuracy, and many experienced physicians often infer current symptoms by analyzing the patient’s medical history, which is yet to be possible with DCNN. The traditional approach is to train the DCNN directly using 3D images. However, this approach is too complex and computationally expensive and requires a lot of model training. Previously, Lindsey et al. [58] proposed deep-learning methods for fracture class detection on 2D images to address this problem. However, these methods are only based on 2D images and cannot be directly applied to 3D images. There is still room for further improvement in practical application and effectiveness. Kazutoshi et al. [59] proposed multiple 2D real-time detection YOLOv3 methods for pelvic fracture diagnosis. Each 2D-DCNN detects the image in different directions, and for each direction, three 2.5D plate images with different thicknesses are synthesized, and each YOLOv3 model simultaneously detects the fracture candidates in different directions. Finally, 3D crack regions were detected by integrating these crack candidates. The results noted a recall and precision of 0.805 and 0.907, respectively, confirming the feasibility of multiple 2D detection models for fracture diagnosis and significantly reducing the computational cost. However, the study only examined issues such as the presence of fracture without grading the degree of fracture. Li et al. [60] proposed a fracture grade identification method by applying the YOLOv5 network search optimization algorithm for graded diagnosis of osteoporotic vertebral fracture (OVF). By accurately detecting the vertebral position, the system realized the graded fracture diagnosis and achieved an accuracy rate of 96.85%, far more than the 54.11% independently detected by physicians. The specific indicators of the above study are shown in Table 11.
2.2.3 Intertrochanteric Fractures
Takaaki et al. [61] used an AI-assisted method to diagnose inter-rotor fractures. They used 3346 datasets to train and validate the Visual Geometry Group-16 (VGG_16) model and showed an accuracy of 95.5%, higher than the 92.2% of orthopedic surgeons. Liu et al. [62] proposed a better Faster-RCNN target detection algorithm for identifying fracture lines in intertrochanteric fractures and compared its performance to the physician level. The study’s results showed that the accuracy and specificity of Faster-RCNN were significantly better than previous methods and required only a quarter of the physician’s time. However, the study database contained only frontal and lateral hip radiographs and did not apply to diagnosing lateral radiographs, and the results were not validated.
Early detection of incomplete fractures and immediate treatment is one of the critical aspects of medical treatment of atypical femur fractures. However, general practitioners often miss the diagnosis of incomplete fractures. Taekyeong et al. [63] developed an integrated migration learning-based model for detecting and localizing fractures to address this issue. They selected six models (EfficientNet B5, B6, B7, DenseNet 121, MobileNet V1, and V2) for migration learning to avoid the problem of needing more features due to small datasets, which in turn may lead to situations such as overfitting. The study used 1050 datasets (which contained 100 images of incomplete fractures) to train the models, and the three highest accuracy and five highest accuracy models were combined and compared. The results show a high accuracy of 0.998 for the former in recognizing incomplete fracture images, which demonstrates the significant effect of migration learning in classifying and detecting fracture images. However, the inability to evaluate the fracture probability during detection limits its application. The specific indicators of the above study are shown in Table 12.
Responding to the problem of emergency room medical personnel in recognizing multiple fractures in conventional radiographs that are easily missed and misdiagnosed, Jakub et al. [64] applied deep learning to skeletal radiographs for the first time; they used five publicly available deep learning networks, which they improved, trained on 25,000 wrist, hand, and ankle photographs selected from hospitals to detect fractures, lateral deviations, body parts, and examination views. Comparisons with two senior orthopedic surgeons showed over 90% accuracy in identifying body parts and examination views, 90% accuracy in lateral deviation, and 83% accuracy in fracture detection. Although these metrics are similar to those of physicians, the system error rate increases when dealing with blurred images and a lack of data. Rebecca et al. [65] developed a deep learning system for detecting fractures throughout the musculoskeletal system. The system contained ten convolutional neural networks, each using a tiny variant of the extended residual network architecture23. The study used 314,886 datasets, divided into training and validation sets at a ratio of 9:1. Various metrics showed that the system accurately mimicked the expertise of orthopedic surgeons and radiologists in detecting fractures in adult musculoskeletal X-rays, demonstrating better robustness in the face of clinically variant cases and cases considered more challenging by physicians. Takaki et al. [66] applied automated localization and classification of Faster R-CNNs to pelvic, rib, and spinal fractures. It was found that with the help of this model, orthopedic surgeons, especially those with less experience, were significantly more sensitive to pelvic, rib, and spinal fractures. In addition, the model has good fracture detection sensitivity and accurately detects multiple fractures in humans. However, the study did not cover multiple fractures in the human body and could not fully assist healthcare professionals in detecting fractures throughout the body. The specific metrics of the study are shown in Table 13.
2.2.5 Diagnosis of Subtle Fractures
Liu et al. [67], for the first time, applied AI assistance to X-ray images to aid in the clinical diagnosis of tibial plateau fractures (TPFs). The study found that the AI algorithm recognized TPFs with an accuracy of 0.91, which is close to that of orthopedic surgeons (0.92 ± 0.03), but the recognition speed is 16 times faster than that of physicians. However, the study only focused on fracture line recognition and did not include fracture classification. Seok et al. [68] investigated the ability of deep learning algorithms to detect and classify proximal humerus fractures in anterior shoulder radiographs and compared them with a human group. The results showed that the CNN outperformed general practitioners and orthopedic surgeons, especially when classifying complex fractures, which is the most challenging area for human practitioners to differentiate; the CNN performed better. However, the reliability of the algorithm still needs to be improved. Gan et al. [69] used the Faster R-CNN model to detect distal radius fractures and found that the model had similar diagnostic capabilities to those of orthopedic surgeons. However, due to the small dataset used in the study, the results may not be satisfactory in real-world applications. Choi et al. [70] used a deep learning algorithm with a two-input convolutional neural network in combination with anteroposterior (AP) and lateral X-rays of the elbow to automatically detect pediatric supracondylar humerus fracture and compare it with the physician’s performance. The study results showed lower model specificity and PPV relative to human readers. This series of studies demonstrates the potential of deep learning to diagnose microfracture sites in humans. The specific indicators of the above study are shown in Table 14.
The above studies show that deep learning has achieved many advanced results in various types of fracture diagnosis. Tables 10 to 14 summarize the application methods of deep learning in fracture diagnosis in different parts of the human body. Among the various studies mentioned above, the image classification assistance models account for the most significant number, and all of them can automatically classify fractures with high accuracy. However, they are least used in practical clinical applications due to their inability to provide more detailed metric information, such as fracture location and fracture line shape. On the other hand, the research on target detection can obtain both the location and category information of the fracture, which benefits the doctors’ diagnosis in the actual clinic. However, it needs to consume more computational resources when dealing with large-scale data, and there is still much room for improvement in speed enhancement. Among the above studies, image segmentation is the least studied. Although image segmentation models can provide more detailed information about fracture contours and anatomical structures, which is more helpful for doctors to determine the scope and severity of fractures in the clinic, it is still challenging to deal with complex scenarios, such as overlap and occlusion between multiple fractures. Therefore, future research in deep learning applied to fracture diagnosis may focus more on improved aspects of target detection and image segmentation.
Large datasets are often required in AI-assisted fracture diagnostic studies; however, lack of interpretability and imaging ambiguity have been problems faced in past studies. Wu et al. [71], to cope with this problem, proposed the Functional Ambiguity Mitigating Operator (FAMO) model aimed at mitigating the impact of functional ambiguity on fracture detection, which applies to radiographs of a wide range of body parts. This study investigated whether good diagnostic results can be achieved with small datasets. In the study, ResNext 101 + FPN was used as the base network structure, processed with the addition of the FAMO model, and the other group was kept as ResNext 101 + FPN as a control. The study results showed that the FAMO model performed better in sensitivity, specificity, and AUC, with most AUCs exceeding 80% per case in different body parts. The new operator FAMO proposed in the study helps to mitigate feature ambiguity in X-ray fracture detection, thereby improving sensitivity and specificity at the level of per fracture, per image, and per case in all body sites. However, the unbalanced proportion of sites in the dataset and the single data source still need further improvement.
Different forms of fracture cause inaccurate results not characterized by categorization during imaging. To address this problem, Lee et al. [72] introduced radiological reports as additional information in a medical image classification task for the first time. They proposed a neural network with an encoder-decoder structure trained using radiology reports as auxiliary information and applied a meta-learning approach to generate adequate classification features. The network then learned classification representations from X-ray images and radiological reports. The results show a classification accuracy of 86.78% for the training dataset and an F1 score of 0.867 for fracture or standard classification, demonstrating the potential of deep learning to improve performance. However, the dataset’s imbalance and the system’s generalization ability still need to be improved.
Pawan et al. [73] proposed a data enhancement technique called BoostNet to improve the performance of deep neural networks in a four-step approach: 1. Several deep learning models are compared. Champnet is selected as the base model. 2. Datasets with different resolutions are evaluated to enhance the model performance. 3. The model is combined with image enhancement techniques (constrained self-adaptive histogram equalization, high-frequency filtering, and unsharp masking) to enhance the model performance further. 4. The model performance is verified by luminance order error to validate the BoostNet results. Their classification scheme achieved 95.88%, 94.99%, and 94.18% accuracy in musculoskeletal radiograph bone classification compared to ChampNet + CLAHE, ChampNet + HEF, and ChampNet + UM, respectively. This method demonstrates the feasibility of data enhancement in orthopedic images.
2.4 Assisting in the Diagnosis of Orthopedic Diseases
Early diagnosis and timely control of diseases are essential for treating patients and saving medical resources. Many diseases are difficult to treat in the later stages and are associated with many sequelae. However, the early diagnosis of many diseases is often complicated, resulting in delayed medical treatment. Applying deep learning in orthopedics frees patients from cumbersome examinations and even realizes remote system consultation for patients while saving medical resources for hospitals, which has significant application value.
The complex process of traditional scoliosis screening methods, the significant differences in anatomical structures of patients of different ages, and the low tissue contrast of X-ray spine images lead to inaccurate manual observation of the spinal curvature. At the same time, the patients are also at risk of radiation exposure. To address this problem, Yang et al. [74] developed a deep learning algorithm, a model that automatically screens for scoliosis on images of a patient’s back after removing clothing. The results show that the algorithm outperforms professional physicians in detecting scoliosis and grading severity. Patients can be screened remotely without being exposed to radiation risks. The study also showed promising results in initial diagnosis and prevention. However, the dataset used in the study needs to be more homogenous, containing only confirmed cases. In addition, the images used in the study were two-dimensional, and the image dimensionality enhanced to improve the model’s accuracy is an area that could be improved in the future. Ananthakrishna et al. [75] proposed an accurate computer-based automated measurement method that estimates spinal curvature based on identified anatomical landmarks and categorizes the scoliosis into four grades, which helps to minimize subjective errors and shows good accuracy during testing.
2.4.2 Detection of Lumbar Disc Degeneration
Traditional lumbar disc detection is too complicated and lacks quantitative standards. Zheng et al. [76] proposed a T2MRI-based lumbar disc degeneration (IVDD) segmentation network and quantification method, which consists of three modules and can realize high-precision segmentation of the IVDD region. The study demonstrated the relationship between lumbar disc degeneration and patient information that developed quantitative criteria for IVDD. This quantitative standard supports clinical trials and scientific research while also improving the efficiency of patient care. However, the study was limited to a trial in China, so the results may not be applicable globally.
In response to the high price of dual-energy X-ray bone densitometry for osteoporosis diagnosis and its limited availability in developing countries, Ryoungwoo et al. [77] applied deep learning model for the first time to the study of the acceptable diagnostic outcome of osteoporosis. They used a deep learning algorithm to predict osteoporosis by using simple hip radiography and built a deep neural network model. They randomly selected 1001 images and divided them into a training, validation, and test set. Modules such as external validation and attention mechanisms were also introduced. The study showed that the model achieved satisfactory results in all indicators and external validation, proving that the deep learning network model has potential value as a screening tool in osteoporosis diagnosis. However, due to the small dataset, it was impossible to classify the images into three categories (normal-osteopenia-osteoporosis). It only dichotomized the images, a significant limitation in practical applications. In addition, the subjects in the dataset were limited to postmenopausal middle-aged women, which is not generalizable in the scope of the group targeted.
Traditional methods for detecting developmental dysplasia of the hip (DDH) tend to have low accuracy when targeting children’s pelvic anatomy. Zhang et al. [78] applied deep learning to anterior pelvic radiographs to diagnose DDH in children. Compared with clinician-led diagnosis, the deep learning system was highly consistent, more convenient, and effective in DDH diagnosis. On top of that, Hiroki et al. [79] further improved the model by using YOLOv5 for DDH detection for the first time, developing a deep learning model in combination with a single-shot multi-box detector (SSD), and introducing migration learning. The dataset contains 205 standard images and 100 DDH images. The experimental results show that the sensitivity and specificity of the model are 94% and 96%, respectively, and the model also outperforms the standalone SSD model. However, there is still room for improvement with the limited number of datasets and the level of object detectors.
2.4.5 Cervical Spinal Cord Detection
Merali et al. [80] used a deep learning approach for the first time to detect degenerative cervical myelopathy (DCM) [81]. The model used a ResNet-50 neural network with class activation maps and used 6588 images to construct the dataset. It was found that the model performed well in detecting spinal cord compression in cervical MRI scans. Furthermore, in clinical trials, the model could automatically encode MRI scans for automatic feature extraction and generate data for secondary analysis, demonstrating the feasibility of training existing CNNs in new medical imaging classification tasks. However, the dataset contains only confirmed cases and lacks images of normal and mild patients. In addition, peripheral spinal cord compression was not differentiated during dataset labeling, which may trigger clinical symptoms in practical use. Therefore, improvement and categorization of the dataset are the following steps to be considered in this study.
Patients with spinal cervical myelopathy (CM) are often delayed in optimal treatment due to the lack of early symptoms. Eriku et al. [82] developed a machine-learning-based case screening method to address such problems. They proposed a technique for early detection of CM that encourages patients to consult a spine specialist to confirm the diagnosis and receive early treatment. The data was analyzed to screen out patients with CM by recording the differences in drawing time and drawing pressure between patients with CM and normal subjects when depicting spiral, square, and triangular waves on a tablet computer. The results showed that the model had a sensitivity and specificity of 76% in identifying whether a patient had CM, with an AUC of 0.80. However, other writing-motor disorders that could affect the results were not analyzed, and the method lacked sufficient sensitivity for further research to improve it for clinical use. A detailed description of the above essential studies is shown in Table 15.
Knee osteoarthritis (KOA), a common musculoskeletal disorder, is currently limited to medical treatment for symptomatic relief and total joint replacement surgery. Therefore, successful prediction of KOV progression would save millions of patients from surgery. However, conventional methods are usually subjective in their estimation of symptoms and radiographs, and therefore, an objective adjunct system is needed to help physicians make the diagnosis.
Aleksei et al. [83] proposed a multimodal machine learning-based KOA progression prediction model, which directly utilizes raw radiological data, physical examination information, patient history, anthropometric data, and optional radiologist statements (KL level) to predict the progression of structural KOA. By using 3,918 images for the test evaluation, they obtained an area under the ROC curve of 0.79 and an average accuracy of 68%, which gives the model a clear advantage in all metrics compared to reference methods such as logistic regression. However, the method is too dependent on the KL classification system and a single dataset, which needs improvement. Subsequently, Bany Mohammed et al. [84] proposed a CNN model and interpretable ensemble consisting of three modules. The ensemble included a scale-invariant and aspect-ratio-preserving model for localizing the knee joint and created multiple instances of a “hyperparameter-optimized” CNN model. In addition, they created an integrated scoring system based on the Kellgren-Lawrence grading (KL) scale to assess the severity of KOA and provide visual interpretation to predict disease progression. They tested the model using 37,996 datasets, and metrics showed that the method performs best in current KOV testing. However, the method has some limitations, such as low performance in classifying KL = 1, duplicate data in the dataset, and over-reliance on X-rays. Hegadi et al. [85] used ANN to differentiate between X-ray images of healthy and diseased knees, extracted the synovial cavity region from the images, and calculated the curvature values using noise cancellation and image enhancement techniques, which were shown to be 100% accurate. However, the experiment used only 42 datasets, making it possible for the results to be highly biased.
Knee joint effusion is a common trigger for knee osteoarthritis; however, traditional methods for effusion detection are costly. Sandhya et al. [86] developed a high-density neural network model. They trained and evaluated the network’s performance using low-resolution images to investigate the model’s applicability in low-cost MRIs. They validated the model with 163 validation sets and checked the stability of the model by adding zero-mean Gaussian noise to the test images. The results showed that the model’s accuracy only decreased by 1% in the presence of interference. Compared to the VGG16 network, the model demonstrated a significant advantage in distinguishing between normal and high fluid levels, and its overall performance outperformed that of radiologists. The feasibility of using neural network models for detection at low-resolution images was confirmed. However, the dataset used in this experiment is relatively tiny. Further model training and parameter optimization will be valuable directions for future work.
Bone tumors are classified as benign and malignant, and the treatments differ. Benign tumors can be cured entirely by small resection of the tumor and have a better prognosis. Malignant tumors, on the other hand, require extensive surgery with chemotherapy and other methods and have a poorer prognosis [87]. Therefore, it is crucial to accurately determine the degree of malignancy of the tumor at the initial stage of treating the patient. Nonetheless, traditional bone tumor diagnostic methods such as CT, X-rays, and MRI cannot identify complex and subtle bone tumors well. The application of AI in bone tumor detection and diagnosis has the advantages of fast speed, high accuracy, and identification of complex tumors. It has gradually begun to be applied in the clinic.
Kaito et al. [88] proposed a method to automatically assess bone tumors’ benign or malignant nature using deep learning and compared two networks, VGG 16 and ResNet 152. The experimental results showed that VGG 16 had better results in terms of recall and accuracy. In diagnosing six benign and four malignant patients, the model successfully classified them correctly. However, it requires physicians to manually segment the images, limiting its application in the clinic. Vlad et al. [89] used two pre-trained residual convolutional neural networks to classify images extracted from an MRI dataset for two classifiers, T1 and T2. It is worth noting that the amount of data used for the T1 classifier is twice that of the T2 classifier. Clinical trials have confirmed that the T1 classifier outperforms the T2 classifier, and both classifiers enable automatic segmentation of MRI images, thus significantly reducing the workload of physicians. However, these studies were limited to classifying bone tumors into benign and malignant categories, which could not solve practical clinical problems. Li et al. [90] developed a deep learning model called YOLO, which can detect and classify bone lesions on full-field radiographs with limited manual intervention. They trained the model using 1085 bone tumor radiographs and 345 normal bone radiographs. In the detection task, the model’s accuracy on the internal and external validation sets was 86.36% and 85.37%, higher than the two radiologists’ 81.87% and 79.27%, respectively. In addition, physicians in clinical practice favor the model’s ability to detect typical, benign, neutral, and malignant tumor types.
Biopsy necrosis rate is the traditional method of measuring bone tumor sensitivity and guiding postoperative chemotherapy. However, studies have shown that this invasive procedure carries risks for patients [91]. For this reason, Xu et al. [92] proposed a new method for detecting necrosis rates using time-series X-ray images. This method utilizes a generative adversarial network with long and short-term memory to generate time-series X-ray images, and an image-to-image transformation network produces an initial image. This method enlarges rare bone tumor X-ray images by a factor of 10, resulting in necrosis rate grading results similar to biopsy. It is the latest technique for detecting rare bone tumors’ necrosis rate, overcoming conventional methods’ time-consuming and invasive problems.
2.4.8 Orthopedic Rare Class Disease Detection
Zafer et al. [93] proposed a new method for training deep neural networks with limited data to address the problem of a limited number of rare disease datasets. They trained CNN models by modifying data created from normal hip radiographs. Patient images were not used in the training phase, and Legg-Calve-Perthes disease (LCPD) images were tested in the testing phase. The results showed the method was more accurate than the experimental specialized physicians. However, due to the scarcity of datasets, test and training data were mixed in the experiment. In addition, the accuracy of the model results was somewhat affected because only the height of the femoral head was changed, especially the lateral column. Nonetheless, this experiment provides valuable lessons for researching rare diseases in orthopedics.
A detailed description of the above important studies is shown in Table 16.
The above studies show that machine and deep learning have achieved good results in diagnosing orthopedic diseases. Tables 15 and 16 summarize the methods and metrics for applying deep learning to diagnose diseases. The 2D images in the above studies are the most extensive and perform well in accuracy. However, 2D images cannot provide more detailed information about the lesions of the disease in practical applications and, therefore, perform poorly in actual clinical results. More importantly, most of the above studies have generally low-quality datasets and severely limited study subjects, which still have significant limitations in clinical applications. Therefore, future studies applying deep learning and machine learning to diagnose orthopedic diseases may focus more on image upgrading and dataset quality improvement.
3 Progress in the Application of Artificial Intelligence in Orthopedic Surgery
In recent years, with the development of orthopedic surgery concepts toward refinement, minimal invasiveness, and low risk, the application of AI to orthopedic surgery has become an area of great interest. Applying deep learning, machine learning, and robotics to surgery improves surgical safety and reduces radiation exposure to doctors and patients, which plays a crucial role in the rapid recovery of patients after surgery and reduces surgical stress for doctors.
3.1 Deep Learning to Reduce Orthopedic Surgery Risks
In orthopedic surgery, preoperative prediction of surgical risk, clarification of lesion location, analysis of implant parameters, and constant monitoring of intraoperative patient physiology and postoperative recovery are all critical steps. The general flow of spinal surgery is shown in Fig. 11. However, the preoperative, intraoperative, and postoperative stages of traditional orthopedic surgery are the sole responsibility of the physician, which may lead to miscalculations when the physician is inexperienced or overburdened with work. Once the link error occurs, it will seriously affect the patient’s surgical outcome. Applying deep learning to orthopedic surgery can alleviate the above problems and reduce medical errors.
Figure 11: Flow chart of spine surgery
3.1.1 Preoperative Prediction of Risk
Preoperative prediction of surgical outcomes and risk assessment are integral to the surgery. Incorrect risk assessment can result in additional care or readmission of patients after surgery, which imposes a significant economic burden on the healthcare system [94]. PremN and other researchers [95] developed machine learning algorithms based on preoperative big data for predicting hospitalization time and cost of postoperative patients and proposed a model that takes into account the patient’s risk of comorbidities, who trained their model using data from 122,334 postoperative patients, and found that the machine learning algorithm demonstrated excellent validity, reliability, and responsiveness concerning age, race, gender, and comorbidity scores (“risk of disease” and “risk of morbidity”). These tools are expected to support physicians as they develop surgical plans for their patients, helping to identify potential risks, avoid them, and predict possible complications.
3.1.2 Intraoperative Assisted Surgery
Spinal deformities are usually accompanied by various problems such as scoliosis, kyphosis, vertebral rotation, and wedge degeneration, which will make the corrective plan for the patient exceptionally difficult if the location of the lesion cannot be accurately known before the operation. Still, it is often difficult to comprehensively observe the specific information about the location of the lesion by traditional detection methods [96]. Chen et al. [97] proposed a vertebral body that can automatically locate and identify the vertebral body in the three-dimensional CT volume of the Joint CNN learning model (J-CNN), which is based on an initial set of vertebral centroids generated by a random forest classifier. J-CNN can identify vertebral body types and exclude false detections effectively. It was also validated on the MICCAI 2014 Vertebral Bone Localization and Recognition Computational Challenge dataset, which showed an improvement in recognition rate of 10.12% compared to the state-of-the-art method at that time. This method helps physicians to locate vertebral positions quickly and accurately in the clinic.
With the wide variety of joint replacement prostheses available, traditional methods could be slow to match the patient’s prosthesis and have a large margin of error, making it challenging to achieve optimal results with joint surgery. Myriam et al. [98] analyzed the geometric parameters of the implants and the stress shielding effect by combining machine learning with parametric finite element analysis to achieve the optimal performance of the short-shanked prostheses. The study’s results showed that the total length of the implant shank is not the only parameter that affects stress shielding; the radius and width of the implant stem also influence the results. This method is faster and more accurate than conventional techniques and contributes to the implant's long-term efficacy.
3.1.3 Postoperative Testing Indicators
Inaccurate placement or inadequate fixation of the implant may lead to dislodgement of the prosthesis and infection of the surrounding tissue. Therefore, imaging analysis of postoperative implants is critical. Traditional methods require X-rays to diagnose postoperative mechanical loosening, but recognizing X-rays may be difficult for inexperienced physicians [99]. Borjali et al. [100] developed an automated tool to detect postoperative mechanical loosening by training deep convolutional neural networks using X-rays. They elaborated on the CNNs learning process that explains how the system works. This research has increased the confidence of healthcare professionals in applying AI in clinical practice.
Álvaro et al. [101] first proposed a multivariate algorithm called AI-HPRO, which combines Natural Language Processing (NLP) and Extreme Gradient Boosting. They applied it to the detection of surgical site infections in patients undergoing hip replacement surgery to solve the problem of time-consuming and resource-consuming detection of traditional surgical site infections (SSIs). The method screens for SSIs in hip replacement surgery patients by combining NLP and extreme gradient boosting. The results of the study show that the clinical indicators are even better than manual testing, where the monitoring time is reduced from 975/h to 63.5/h, and the total number of clinical records manually reviewed is reduced by 88.95%, which is a significant saving of healthcare resources. The study demonstrated that an algorithm combining NLP and extreme gradient boosting techniques can achieve accurate real-time monitoring of orthopedic SSIs.
The above studies show that machine learning and deep learning have achieved satisfactory results in assisting orthopedic surgery. However, despite the excellent performance of the above models in terms of metrics, only a few studies have been carried out in clinical applications. Most studies still suffer from the black box problem, where doctors are not aware of the model and may be reluctant to follow the recommendations of the AI model because the model does not have a human-computer interface, resulting in unintuitive results and the inability to ask questions. Moreover, the EU addressed the liability issue by including a provision in the General Data Protection Regulation that AI algorithmic decisions about humans must be interpretable [102]. Therefore, future research on machine learning and deep learning in assisting orthopedic surgery should focus on the interpretability of the model and develop an assisted diagnostic system; the development of an assisted diagnostic system can help doctors understand the principles of the model more intuitively and the inclusion of an interpretable module in the model can solve the corresponding legal issues.
3.2 Robotics in Orthopedic Surgery
Most orthopedic surgeries require the implantation of prostheses due to the complex structure of the human skeleton and its proximity to vital blood vessels. However, inaccurate implant placement due to instrument tremors is inevitable when surgeons are exhausted [103], thus increasing the difficulty of surgery. Therefore, AI-assisted analysis and pathologic judgment are critical to assist surgeons in determining the optimal surgical path and reducing surgical errors [104]. In addition, the rigidity of the human skeleton allows the robot to fixate implants quickly, and the robot’s navigation system enables it to handle implants more accurately than the surgeon. Moreover, the camera-manipulated robot provides a stabilized image of the surgical area, which can improve the stability and accuracy of the surgeon during surgical manipulation, thus improving the safety and reliability of the surgery, surgical success, and prognostic outcomes. Considering the general exposure risk to the surgeon in modern surgical procedures, using robots in the necessary part of the surgery can reduce the risk to the healthcare provider. The metrics that should be considered for orthopedic surgery are shown in Table 17; the higher the total score, the better the surgical outcome. As early as 1985, Kwoh et al. [105] began using robots in surgery; however, surgical robots were not introduced into orthopedics until 1992, which stemmed from a collaboration with the U.S. Food and Drug Administration. In recent years, the results achieved by robots in orthopedic surgery have been quite remarkable. Table 18 lists the companies represented by today’s orthopedic surgical robots and their products. Pictures of the da Vinci V and Tiangui II Orthopedic surgical robots are shown in Figs. 12 and 13.
Figure 12: Da Vinci V
Figure 13: Tiangui II orthopedic surgical robot
3.2.1 Robot-Assisted Navicular Fracture
Fractures of the navicular bone of the hand can affect the movement and stability of the wrist joint of the organism. If the patient does not use the correct treatment in time, it can lead to osteonecrosis, chronic pain, traumatic arthritis, and other serious consequences. Traditional medical methods can lead to sequelae such as postoperative dysfunction [106]. To address these issues, Wang et al. [107] designed an automated multi-degree-of-freedom (DOF) surgical robot with a computer-assisted navigation system for verifying the accuracy of percutaneous navicular bone guides and performed experimental studies on cadavers. The results showed that the method achieved the expected results. Xiao et al. [108] compared the effectiveness of unarmed vs. robotic assistance in the medical treatment of navicular fractures by recording metrics from different procedures and showed that the robotic-assisted group had significantly better accuracy, shorter time, and less radiation exposure. Wang et al. [109] investigated whether robot-assisted percutaneous screw internal fixation could be used to treat navicular fractures. Patients undergoing robotic-assisted surgery were compared to those undergoing conventional incisional reduction and internal fixation (ORIF) of the navicular fracture. At subsequent postoperative follow-ups, implant accuracy VAS scores were superior to those of the conventional procedure in both the robotic-assisted group.
3.2.2 Assisting Femoral Surgery
Surgery has been a challenge for physicians in patients with intertrochanteric fractures who often suffer from post-traumatic stress and various underlying conditions caused by surgical intolerance [110]. Reducing operative time, bleeding, and implant accuracy has been the research direction in this procedure. Maeda et al. [111] developed a femoral fracture reduction robotic system called “FRAC-Robo” to assist in dissection and maintenance of reduction during orthopedic surgery. Clinical trials have shown that the robot can generate sufficient force and torque to reduce intertrochanteric fractures, thus confirming the device’s effectiveness in reducing intertrochanteric fractures. Ye et al. [112] proposed a system for robot-assisted femoral fracture repositioning, designing actuators whose trajectories are all linear and whose low-speed and smooth characteristics make them preferable for femoral fracture-type surgeries. A robot-assisted surgery group and a freehand control group were also set up. The study results showed that the number of fluoroscopies, operation time, intraoperative bleeding, and postoperative recovery were better in the robotic group than in the freehand group. A detailed description of the above essential studies is shown in Table 19.
3.2.3 Assisted Total Knee Arthroplasty
In 1972, Insall [113] proposed total knee arthroplasty to reconstruct the patient’s joint, correcting the problem of joint deformity and enhancing knee mobility. However, conventional joint replacement surgery is associated with a high risk of postoperative pain, limited postoperative mobility, and poor postoperative rehabilitation. Therefore, the current technology’s problem is how to minimize the incision and enhance surgical precision [114]. Robot-assisted total knee replacement, on the other hand, breaks through the limitations of traditional surgery and outperforms it in terms of precision and incision.
Matjaz et al. [115] investigated a robotic system for total knee replacement (TKR) surgery. Preoperative planning software based on computed tomography enabled accurate surgical procedure planning. During the procedure, the surgeon guides a small specialized robot called Acrobot. The robot limits the motion to a predefined area by active constraint control, which allows the surgeon to guide the robot to make ankle cuts for high-precision TKR installation. The system has been successfully applied in seven clinical trials with satisfactory results.
Werner et al. [116] introduced the robot into total knee arthroplasty, and their study found that the mean difference between preoperatively planned and performed postoperative tibio-femoral alignment in the robot group was 0.88, which was significantly lower than that in the conventional control group, which was 2.68. This result suggests that the robot has a promising future for use in total knee arthroplasty. However, the problems of long operation times and excessive costs still need to be solved.
Cobb et al. [117] compared the results of the Acrobot system with conventional surgery in unicompartmental knee replacement. Their study found that in all patients in the Acrobot group, the tibia and femur were aligned within 2° of the planned position in the coronal plane. In contrast, only 40% of the patients in the conventional group achieved this accuracy. Although the scoring system was subject to a large margin of error and did not allow for extended observation of the patient’s postoperative recovery, it still demonstrated that the procedure was less risky and invasive with robotic assistance.
The complex anatomy of the spine, surrounded by the immediate vicinity of vital blood vessels and nerves, makes spinal surgery difficult. Pedicle screw instrumentation has been the gold standard technique for spinal screw fixation since its introduction by Roy Camille in 1970 [118]. Although pedicle screw instrumentation has been clinically effective, misplaced screws continue to cause severe neurovascular injury. In conventional clinical spine surgery, screw misplacement rates are as high as 15.7% [119]. In recent years, with the development of spinal surgical techniques and concepts, spinal surgery has made significant progress in precision, minimally invasiveness, and postoperative recovery. However, current techniques still need help with radiation exposure and nail placement accuracy.
Ponnusamy et al. [120] introduced the da Vinci robot into posterior spine surgery for the first time and tested it on a porcine model. The study results show that the robot can perform major non-instrumented maneuvers in the posterior part of the spine, enhancing the procedure’s safety while reducing the surgeon’s stress during the operation.
Addressing the relatively low accuracy of pedicle screws and the need to improve surgical safety, Onen et al. [121] studied the clinical and imaging outcomes of spine surgery in 27 patients by using Spine-Assist and setting up experimental and control groups. The results of the study showed that robotic spine surgery provided high accuracy in pedicle screw placement and significantly reduced radiation exposure. However, robotic-assisted surgery is expensive and may still need help in practical rollout. Lin et al. [122] addressed the problem of the high cost of surgery. They cooperated with a medical device company to develop a unique surgical robot for spinal surgery, Orthobot, and experimented on porcine lumbar cones. The results of the study showed that the excellent rate of both radiation exposure time and pedicle screw implantation was higher than that of the traditional method and even superior to minimally invasive surgery in terms of incision. However, the experimental sample size was small, and it is not possible to determine for the time being whether other orthopedic conditions (e.g., osteoporosis) may affect the clinical results. Therefore, the generalizability of these results still requires further in-depth studies.
Li et al. [123] proposed a collaborative spinal robotic system to assist laminectomy in response to the lack of precision in a conventional decompressive laminectomy. The system’s reliability was verified through experiments on porcine lumbar vertebrae and compared with the unarmed group. A comparison of the studies revealed that the time required, whether the vertebral plate was penetrated or not, and the remaining thickness of the vertebral plate was better than those of the freehand group in terms of lateral laminectomy in the machine group. The findings validate the safety of robot-assisted laminectomy. However, the efficiency and time of the system still need to be improved.
Derek et al. [124] used spinal robotics for the first time for pedicle screw placement in children, assessed the effectiveness of the procedure by recording intraoperative radiation vs. postoperative screw position, and analyzed common causes of misalignment such as lateral deviation. The results show that even in pediatric patients, the technique can achieve results far exceeding freehand surgery. A detailed description of the above essential studies is shown in Table 20.
3.2.5 Assisting Minimally Invasive Surgery
Numerous studies have shown that minimally invasive surgery is superior to open surgery in terms of safety and price [125]. Bowen et al. [126] applied a novel real-time image-guided robotic system for the first time to short-segment lumbar fusion surgery in patients with diagnostic degenerative diseases. By comparing the performance of the robot-assisted group and the unarmed group in terms of screw accuracy, discharge time, and bleeding found that the robotic group outperformed the unarmed group in all the metrics, and the study proved that the mechanical group had a lower economic cost. However, the data is too homogeneous and has yet to be applied in the clinic. Zhu et al. [127] used remote-controlled robot-assisted minimally invasive treatment of displaced femoral fractures, where the fracture reduction procedure was performed manually under teleoperation. The results proved that this robot-assisted system has high reduction accuracy, high safety, and low radiation exposure by setting up two trials as a control. With this application, precise fracture reduction can be achieved under visual control, opening the way for teleoperated robot-assisted minimally invasive surgery.
A detailed description of the above essential studies is shown in Table 21.
Tables 19–21 summarize the use of different robotic technologies in various orthopedic surgeries. AI-based robots help to optimize the positioning of devices and tools, avoid the risk of exposure to healthcare workers, and overcome the difficulties of traditional techniques. With the help of these systems, implants are inserted into the patient at the correct angle and depth, improving the safety and accuracy of the procedure. Thus, using robotics in orthopedic surgeries will reduce the need for assistants, thus saving healthcare resources.
Federer et al. [128] provided a scoping review of the use of AI in orthopedics and its changing trends but needed to provide an in-depth study of its nature. Poduval et al. [129] provided a scientific discussion of using AI and machine learning in orthopedics but needed more specific classification studies. Lee et al. [130] classified orthopedic disorders but lacked explanations of the models. Lalehzarian et al. [131] did a scientific discussion on the application of machine learning in orthopedic surgery, but it still needs to include the detection of images. Wang et al. [132] explained the advantages and disadvantages of AI in orthopedic clinical applications but did not include a scientific discussion of the specific applications. Cabitza et al. [6] classified the machine and deep learning models but lacked a discussion of the diseases. Kalmet et al. [133] did a scientific discussion of deep learning in fracture detection but needed a metrics analysis of the models. Therefore, the main contributions of this paper are as follows:
(1) This paper provides an in-depth metric analysis of deep learning and machine learning models. It systematically summarizes the relevant applications and their metrics, based on which scientific opinions on the future direction of improvement are provided.
(2) The classification of fractures and orthopedic diseases is discussed more comprehensively, and the advantages and shortcomings of AI in orthopedic image detection are summarised scientifically.
(3) The application of robotics in orthopedic surgery is explored, and the importance of robotics and the future direction of improvement are discussed rationally.
The core problem in the current healthcare field is that physician-centered healthcare resources cannot meet patients’ needs, creating an urgent need for medical AI [134]. The above studies on fracture detection and disease diagnosis showed that the accuracy, specificity, and sensitivity of AI in image recognition are better than the independent judgment of physicians. In practical application, the collaborative diagnosis between AI and doctors can further shorten the manual diagnosis time, reduce the rate of leakage and misdiagnosis [135], accelerate the patients into the next step of treatment, and improve patient satisfaction. The application of AI robots in orthopedic surgery has significantly improved surgical safety, accuracy, and postoperative outcomes. Studies have shown that patients who underwent robotic-assisted surgery recovered faster after surgery and had superior physical indicators than patients who underwent unassisted surgery throughout [136–137]. This study confirms that robotic-assisted surgery saves medical resources and provides patients with better medical services. It shows that the application of AI in orthopedics has a good development prospect.
However, although most models studied perform well in metrics, AI is currently only used in a few clinical settings for assisted diagnosis. The main reason for this status quo is that more than just improving the indicators of the model is needed to promote the clinical use of AI. Some core potential and clinically useful indicators are often easily ignored by researchers, such as the model’s ability to generalize, interpretability, etc., and the specific analysis of the current problems are as follows:
(1) It is difficult for researchers to obtain high-quality datasets. The quality and quantity of training datasets directly affect the model’s performance. Still, getting large-scale patient data in the medical field is difficult due to relevant laws and regulations. In addition, the datasets usually need to be labeled with images by professional doctors, which is time-consuming, so the number of labeled datasets is limited. A decrease in the number of datasets can lead to a significant reduction in model accuracy. Therefore, the biggest challenge facing the current research is finding high-quality datasets and ways to overcome legal issues.
(2) Most models need better generalization ability in clinical practice. The main reasons for this problem are that only some models are externally validated, excellent metrics do not translate into good clinical performance, and most disease diagnostic studies usually focus on a single disease, which may be severely limited in practical applications.
(3) Although many scholars have contributed to promoting the application of AI in orthopedics, it is difficult to cover all orthopedic disease studies due to the wide variety of orthopedic diseases. As a result, AI cannot assist doctors in the clinic regarding rare orthopedic disorders.
(4) Models are not interpretable. Although the deep learning model performs well in accuracy, only some scholars have interpreted it. The algorithm’s decision-making process uses opaque hidden layers, and the connections between inputs and outputs are unknown, resulting in the model producing predictions that are not scientifically interpretable. How the model learns from the training and test sets remains to be determined, which limits its use in clinical applications.
(5) Most studies have only dichotomized the disease, whereas a physician often needs to base his interpretation on multiple factors when diagnosing a patient’s condition. Therefore, most studies have limitations in assisting physicians in clinical diagnosis.
(6) Surgical robots are expensive and have only a few auxiliary operating functions, so they do not realize economic benefits.
(7) Some studies have shown some contradictions between the robustness and accuracy of the models [138–139]. Most of the models proposed by researchers need to fully consider the relationship between robustness and precision, which leads to models that cannot be used stably in the clinic.
(8) The robotic system does not consider muscle tremors that may occur in patients during surgery, which may cause additional harm to the patient. In addition, it remains a controversial issue as to who will be held responsible if the model makes an error and causes damage to the patient.
4.3 Future Directions for Improvement
Given the current clinical and technological problems, combined with the existing clinical experience and improvement methods, future research directions for the application of AI in orthopedics can be studied in the following aspects:
(1) For problems such as poor clinical effect of the model, the model’s generalization ability can be improved by introducing a cross-validation step of validation set and test set in the model. At the same time, the attention mechanism and residual structure can be added to enhance the extraction and fusion of deep and shallow features by the model to maximize the preservation of the image’s original information.
(2) For the problem of lack of dataset, researchers can adopt the migration learning approach to improve the convergence speed of the model. At the same time, it should actively cooperate with physicians to rationally communicate with patients and achieve accurate annotation with the assistance of physicians.
(3) To address the problem of model interpretability, the module responsible for interpretability can be considered trained together with the model, or the interpretability module can be added after the training is completed. Both methods can explain the judgment basis of the model to a certain extent.
(4) For situations like the robot’s weak risk resistance during surgery, researchers should actively improve the algorithm and comprehensively consider possible intraoperative conditions to avoid accidents during surgery.
(5) For the function of the model, it is necessary to find a balance between considering robustness and accuracy so that the model is in pursuit of robustness and accuracy at the same time.
(6) Disease progression is a slow process, so when classifying the severity of the disease, it should be segmented as much as possible. Classifying the images used for training into multiple levels will help physicians in clinical practice.
In conclusion, this paper analyzes the studies related to AI in orthopedics, highlighting each study’s contributions, strengths and weaknesses, and the directions that can be improved in the future. The rapid development of AI has enabled it to play an essential role in detecting orthopedic diseases and improving surgical indexes, effectively reducing the burden on doctors, optimizing the use of medical resources, and improving patient satisfaction. It is worth noting that the application of AI in orthopedics is not to replace professional physicians but to assist them in improving efficiency and diagnostic accuracy. It is foreseeable that in the future, the application of AI in orthopedics is expected to turn into a comprehensive diagnostic tool, with more machine learning and deep learning technologies being further developed and applied, based on which patients will enjoy more personalized, precise, minimally invasive, and remote services.
Acknowledgement: The authors would like to express their gratitude for the valuable feedback and suggestions provided by all the anonymous reviewers and the editorial team.
Funding Statement: This work was supported in part by the National Natural Science Foundation of China under Grants 61861007 and 61640014; in part by the Guizhou Province Science and Technology Planning Project ZK [2021]303; in part by the Guizhou Province Science Technology Support Plan under Grants [2022]017, [2023]096 and [2022]264; in part by the Guizhou Education Department Innovation Group Project under Grant KY [2021]012; in part by the Talent Introduction Project of Guizhou University (2014)-08.
Author Contributions: The authors confirm contribution to the paper as follows: Study conception and design: Xinlong Diao, Xiao Wang, Qinmu Wu; data collection: Xinlong Diao, Xiao Wang, Qinmu Wu, Zhiqin He; analysis and interpretation of results: Xinlong Diao, Junkang Qin, Zhiqin He; draft manuscript preparation: Xinlong Diao, Xinghong Fan, Xiao Wang, Junkang Qin. All authors reviewed the results and approved the final version of the manuscript.
Availability of Data and Materials: All data in this paper can be found in Google Scholar.
Conflicts of Interest: The authors declare that they have no conflicts of interest to report regarding the present study.
References
1. S. L. Andresen, “John McCarthy: Father of AI,” IEEE Intell. Syst., vol. 17, no. 5, pp. 84–85, 2002. doi: 10.1109/mis.2002.1039837. [Google Scholar] [CrossRef]
2. W. S. McCulloch and W. Pitts, “A logical calculus of the ideas immanent in nervous activity,” Bull. Math. Biol., vol. 5, pp. 115–133, 1943. doi: 10.1007/bf02478259. [Google Scholar] [CrossRef]
3. Y. LeCuN, Y. Bengio, and G. Hinton, “Deep learning,” Nature, vol. 521, no. 7553, pp. 436–444, 2015. doi: 10.1038/nature14539. [Google Scholar] [PubMed] [CrossRef]
4. D. C. Levin, V. M. Rao, L. Parker, A. J. Frangos, and J. H. Sunshine, “Bending the curve: The recent marked slowdown in growth of noninvasive diagnostic imaging,” Am. J. Roentgenol., vol. 196, no. 1, pp. W25–W29, 2011. doi: 10.2214/ajr.10.4835. [Google Scholar] [PubMed] [CrossRef]
5. J. H. F. Oosterhoff and J. N. Doornberg, “Artificial intelligence in orthopedics: False hope or not? A narrative review along the line of Gartner’s hype cycle,” EFORT Open Rev., vol. 5, no. 10, pp. 593–603, 2020. doi: 10.1302/2058-5241.5.190092. [Google Scholar] [PubMed] [CrossRef]
6. F. Cabitza, A. Locoro, and G. Banfi, “Machine learning in orthopedics: A literature review,” Front. Bioeng. Biotechnol., vol. 6, pp. 75, 2018. doi: 10.3389/fbioe.2018.00075. [Google Scholar] [PubMed] [CrossRef]
7. N. Maffulli et al., “Artificial intelligence and machine learning in orthopedic surgery: A systematic review protocol,” J. Orthop. Surg. Res., vol. 15, pp. 1–5, 2020. doi: 10.1186/s13018-020-02002-z. [Google Scholar] [PubMed] [CrossRef]
8. X. Liu, L. Song, S. Liu, and Y. Zhang, “A review of deep-learning-based medical image segmentation methods,” Sustainability, vol. 13, no. 3, pp. 1224, 2021. doi: 10.3390/su13031224. [Google Scholar] [CrossRef]
9. Z. Ji et al., “Lung nodule detection in medical images based on improved YOLOv5s,” IEEE Access, vol. 11, pp. 76371–76387, 2023. doi: 10.1109/access.2023.3296530. [Google Scholar] [CrossRef]
10. S. Guo, L. Li, T. Guo, Y. Cao, and Y. Li, “Research on mask-wearing detection algorithm based on improved YOLOv5,” Sensors, vol. 22, no. 13, pp. 4933, 2022. doi: 10.3390/s22134933. [Google Scholar] [PubMed] [CrossRef]
11. W. Sheng et al., “Symmetry-based fusion algorithm for bone age detection with YOLOv5 and ResNet34,” Symmetry, vol. 15, no. 7, pp. 1377, 2023. doi: 10.3390/sym15071377. [Google Scholar] [CrossRef]
12. J. Ren, Z. Wang, Y. Zhang, and L. Liao, “YOLOv5-R: Lightweight real-time detection based on improved YOLOv5,” J. Electron. Imaging, vol. 31, no. 3, pp. 033033, 2022. doi: 10.1117/1.jei.31.3.033033. [Google Scholar] [CrossRef]
13. S. Yuan, Y. Wang, S. Lin, T. Liang, W. Jiang and Z. Zhao, “Real-time recognition and warning of mask wearing based on improved YOLOv5 R6.1,” Int. J. Intell. Syst., vol. 37, no. 11, pp. 9309–9338, 2022. doi: 10.1002/int.22994. [Google Scholar] [CrossRef]
14. S. Arunachalam and G. Sethumathavan, “An effective tumor detection in MR brain images based on deep CNN approach: i-YOLOV5,” Appl. Artif. Intell., vol. 36, no. 1, pp. 2151180, 2022. doi: 10.1080/08839514.2022.2151180. [Google Scholar] [CrossRef]
15. Z. Yücel, F. Akal, and P. Oltulu, “Mitotic cell detection in histopathological images of neuroendocrine tumors using improved YOLOv5 by transformer mechanism,” Signal Image Video Process., vol. 36, pp. 4107–4114, 2023. doi: 10.1007/s11760-023-02642-8. [Google Scholar] [CrossRef]
16. H. Zhang, Y. Xu, and J. Liu, “Research on medical image object detection based on swin-transformer and YOLOv5,” in 5th IEEE Int. Conf. on Power, Intell. Comput. Syst. (ICPICS), 2023, pp. 1069–1074. [Google Scholar]
17. R. Yang, W. Li, X. Shang, D. Zhu, and X. Man, “KPE-YOLOv5: An improved small target detection algorithm based on YOLOv5,” Electronics, vol. 12, no. 4, pp. 817, 2023. doi: 10.3390/electronics12040817. [Google Scholar] [CrossRef]
18. O. Ronneberger, P. Fischer, and T. Brox, “U-Net: Convolutional networks for biomedical image segmentation,” in 18th Int. Conf. Med. Image Comput. Comput. Assist. Interv.–MICCAI 2015, Munich, Germany, Springer International Publishing, Oct. 5–9, 2015, pp. 234–241. [Google Scholar]
19. F. Milletari, N. Navab, and S. A. Ahmadi, “V-Net: Fully convolutional neural networks for volumetric medical image segmentation,” in 4th Int. Conf. on 3DV, IEEE, 2016, pp. 565–571. [Google Scholar]
20. M. Z. Alom, M. Hasan, C. Yakopcic, T. Taha, and V. Asari, “Recurrent residual convolutional neural network based on U-Net (R2U-Net) for medical image segmentation,” 2018. doi: 10.48550/arXiv.1802.06955. [Google Scholar] [CrossRef]
21. N. Ibtehaz and M. S. Rahman, “MultiResUNet: Rethinking the U-Net architecture for multimodal biomedical image segmentation,” Neural Netw., vol. 121, pp. 74–87, 2020. doi: 10.1016/j.neunet.2019.08.025. [Google Scholar] [PubMed] [CrossRef]
22. S. Guan, A. A. Khan, S. Sikdar, and P. Chitnis, “Fully dense UNet for 2-D sparse photoacoustic tomography artifact removal,” IEEE J. Biomed. Health Inform., vol. 24, no. 2, pp. 568–576, 2019. doi: 10.1109/jbhi.2019.2912935. [Google Scholar] [PubMed] [CrossRef]
23. J. Zhan, Y. Zhang, Y. Jin, J. Xu, and X. Xu, “MDU-Net: Multi-scale densely connected U-Net for biomedical image segmentation,” Health Inf. Sci. Syst., vol. 11, no. 1, pp. 13, 2023. doi: 10.1007/s13755-022-00204-9. [Google Scholar] [PubMed] [CrossRef]
24. X. Li, H. Chen, X. Qi, Q. Dou, C. Fu and P. A. Heng, “H-DenseUNet: Hybrid densely connected UNet for liver and tumor segmentation from CT volumes,” IEEE Trans. Med. Imaging, vol. 37, no. 12, pp. 2663–2674, 2018. doi: 10.1109/tmi.2018.2845918. [Google Scholar] [PubMed] [CrossRef]
25. Z. Gu et al., “CE-Net: Context encoder network for 2D medical image segmentation,” IEEE Trans. Med. Imaging, vol. 38, no. 10, pp. 2281–2292, 2019. doi: 10.1109/tmi.2019.2903562. [Google Scholar] [PubMed] [CrossRef]
26. S. Moradi et al., “MFP-Unet: A novel deep learning based approach for left ventricle segmentation in echocardiography,” Phys. Med., vol. 67, pp. 58–69, 2019. doi: 10.1016/j.ejmp.2019.10.001. [Google Scholar] [PubMed] [CrossRef]
27. N. V. Keetha and C. S. R. Annavarapu, “U-Det: A modified U-Net architecture with bidirectional feature network for lung nodule segmentation,” 2020. doi: 10.48550/arxiv.2003.09293. [Google Scholar] [CrossRef]
28. W. Chen et al., “Prostate segmentation using 2D bridged U-net,” in 2019 Int. Conf. on Neural Netw. (IJCNN), IEEE, 2019, pp. 1–7. doi: 10.1109/ijcnn.2019.8851908. [Google Scholar] [CrossRef]
29. Z. L. Ni et al., “RAUNet: Residual attention U-Net for semantic segmentation of cataract surgical instruments,” in Int. Conf. Neural Inf. Process., Cham, Springer International Publishing, 2019, pp. 139–149. doi: 10.1007/978-3-030-36711-4_13. [Google Scholar] [CrossRef]
30. W. Zhu et al., “AnatomyNet: Deep learning for fast and fully automated whole-volume segmentation of head and neck anatomy,” Med. Phys, vol. 46, no. 2, pp. 576–589, 2019. doi: 10.1002/mp.13300. [Google Scholar] [PubMed] [CrossRef]
31. Z. Zhou, M. R. Siddiquee, N. Tajbakhsh, and J. Liang, “UNet++: A nested u-Net architecture for medical image segmentation,” in 4th Int. Conf. on MICCAI 2018, Granada, Spain, Springer International Publishing, Sep. 20, 2018, pp. 3–11. [Google Scholar]
32. Q. Jun, Z. Meng, C. Sun, L. Wei, and R. Su, “RA-UNet: A hybrid deep attention-aware network to extract liver and tumor in CT scans,” Front. Bioeng. Biotechnol., vol. 8, pp. 605132, 2020. doi: 10.3389/fbioe.2020.605132. [Google Scholar] [PubMed] [CrossRef]
33. C. Li et al., “ANU-Net: Attention-based nested U-Net to exploit full resolution features for medical image segmentation,” Comput. Graph., vol. 90, pp. 11–20, 2020. doi: 10.1016/j.cag.2020.05.003. [Google Scholar] [CrossRef]
34. K. He, G. Gkioxari, P. Dollár, and R. Girshick, “Mask R-CNN,” in 2017 IEEE Conf. on Int. Conf. Comput. Vis., Venice, Italy, 2017, pp. 2980–2988. [Google Scholar]
35. M. Vania and D. Lee, “Intervertebral disc instance segmentation using a multistage optimization mask-RCNN (MOM-RCNN),” J. Comput. Des. Eng., vol. 8, no. 4, pp. 1023–1036, 2021. doi: 10.1093/jcde/qwab030. [Google Scholar] [CrossRef]
36. B. Felfeliyan, A. Hareendranathan, G. Kuntze, J. Jaremko, and J. L. Ronsky, “Improved-Mask R-CNN: Towards an accurate generic MSK MRI instance segmentation platform (data from the Osteoarthritis Initiative),” Comput. Med. Imaging Graph., vol. 97, pp. 102056, 2022. doi: 10.1016/j.compmedimag.2022.102056. [Google Scholar] [PubMed] [CrossRef]
37. K. Long et al., “Probability-based Mask R-CNN for pulmonary embolism detection,” Neurocomputing, vol. 422, pp. 345–353, 2021. doi: 10.1016/j.neucom.2020.10.022. [Google Scholar] [CrossRef]
38. X. Cao et al., “Application of generated mask method based on Mask R-CNN in classification and detection of melanoma,” Comput. Methods Programs Biomed., vol. 207, pp. 106174, 2021. doi: 10.1016/j.cmpb.2021.106174. [Google Scholar] [PubMed] [CrossRef]
39. G. Lv, K. Wen, Z. Wu, X. Jin, H. An and J. He, “Nuclei R-CNN: Improve mask R-CNN for nuclei segmentation,” in 2nd Int. Conf. Inf. Commun. Signal Process. (ICICSP), IEEE, 2019, pp. 357–362. [Google Scholar]
40. H. Yan, H. Lu, M. Ye, K. Yan, Y. Xu and Q. Jin, “Improved mask R-CNN for lung nodule segmentation,” in 2019 10th Int. Conf. Inf. Technol. Med. Edu. (ITME), IEEE, 2019, pp. 137–141. [Google Scholar]
41. H. M. A. Bhatti, J. Li, S. Siddeeq, A. Rehman, and A. Manzoor, “Multi-detection and segmentation of breast lesions based on mask RCNN-FPN,” in 2020 Int. Conf. Bioinform. Biomed. (BIBM), IEEE, 2020, pp. 2698–2704. [Google Scholar]
42. Z. H. Cui et al., “Application of improved mask R-CNN algorithm based on gastroscopic image in detection of early gastric cancer,” in 2022 46th COMPSAC Conf., IEEE, 2022, pp. 1396–1401. [Google Scholar]
43. Y. Zhang, J. Chu, L. Leng, and J. Miao, “Mask-refined R-CNN: A network for refining object details in instance segmentation,” Sensors, vol. 20, no. 4, pp. 1010, 2020. doi: 10.3390/s20041010. [Google Scholar] [PubMed] [CrossRef]
44. S. Mulay, G. Deepika, S. Jeevakala, K. Ram, and M. Sivaprakasam, “Liver segmentation from multimodal images using HED-mask R-CNN,” in 1st MMMI Conf. on MICCAI 2019, Shenzhen, China, Springer International Publishing, Oct. 13, 2019, pp. 68–75. [Google Scholar]
45. A. Vaswani et al., “Attention is all you need,” Adv. Neural Inf. Process., vol. 30, pp. 5998–6008, 2017. [Google Scholar]
46. J. Chen et al., “TransUNet: Transformers make strong encoders for medical image segmentation,” arXiv:2102.04306, 2021. doi: 10.48550/arxiv.2102.04306. [Google Scholar] [CrossRef]
47. A. Hatamizadeh et al., “UNETR: Transformers for 3D medical image segmentation,” in 2022 IEEE/CVF Conf. Appl. Comput. Vis., 2022, pp. 574–584. [Google Scholar]
48. Y. Zhang, H. Liu, and Q. Hu, “Transfuse: Fusing transformers and cnns for medical image segmentation,” in 24th Int. Conf. on MICCAI, Strasbourg, France, Springer International Publishing, Sep. 27–Oct. 1, 2021, pp. 14–24. [Google Scholar]
49. J. M. J. Valanarasu, P. Oza, I. Hacihaliloglu, and V. M. Patel, “Medical transformer: Gated axial-attention for medical image segmentation,” in 24th Int Conf. on MICCAI, Strasbourg, France, Springer International Publishing, Sep. 27–Oct. 1, 2021, pp. 36–46. [Google Scholar]
50. H. Cao et al., “Swin-Unet: Unet-like pure transformer for medical image segmentation,” in European Conf. on Comput. Vis., Cham, Springer Nature Switzerland, 2022, pp. 205–218. doi: 10.1007/978-3-031-25066-8_9. [Google Scholar] [CrossRef]
51. A. Lin, B. Chen, J. Xu, Z. Zhang, G. Lu and D. Zhang, “DS-TransUNet: Dual swin transformer U-Net for medical image segmentation,” IEEE Trans. Instrum. Meas., vol. 71, pp. 1–15, 2022. doi: 10.1109/tim.2022.3178991. [Google Scholar] [CrossRef]
52. R. Y. L. Kuo, “Artificial intelligence in fracture detection: A systematic review and meta-analysis,” Radiology, vol. 304, no. 1, pp. 50–62, 2022. doi: 10.1148/radiol.211785. [Google Scholar] [PubMed] [CrossRef]
53. Q. Q. Zhou et al., “Automatic detection and classification of rib fractures on thoracic CT using convolutional neural network: Accuracy and feasibility,” Korean J. Radiol., vol. 21, no. 7, pp. 869, 2020. doi: 10.3348/kjr.2019.0651. [Google Scholar] [PubMed] [CrossRef]
54. A. Niiya et al., “Development of an artificial intelligence-assisted computed tomography diagnosis technology for rib fracture and evaluation of its clinical usefulness,” Sci. Rep., vol. 12, no. 1, pp. 8363, 2022. doi: 10.1038/s41598-022-12453-5. [Google Scholar] [PubMed] [CrossRef]
55. L. Jin et al., “Deep-learning-assisted detection and segmentation of rib fractures from CT scans: Development and validation of FracNet,” eBioMedicine, vol. 62, pp. 103106, 2020. doi: 10.1016/j.ebiom.2020.103106. [Google Scholar] [PubMed] [CrossRef]
56. C. Yang et al., “Development and assessment of deep learning system for the location and classification of rib fractures via computed tomography,” Eur. J. Radiol., vol. 154, pp. 110434, 2022. doi: 10.1016/j.ejrad.2022.110434. [Google Scholar] [PubMed] [CrossRef]
57. K. Murata et al., “Artificial intelligence for the detection of vertebral fractures on plain spinal radiography,” Sci. Rep., vol. 10, no. 1, pp. 20031, 2020. doi: 10.1038/s41598-020-76866-w. [Google Scholar] [PubMed] [CrossRef]
58. R. Lindsey et al., “Deep neural network improves fracture detection by clinicians,” Proc. Natl. Acad. Sci., vol. 115, no. 45, pp. 11591–11596, 2018. doi: 10.1073/pnas.1806905115. [Google Scholar] [PubMed] [CrossRef]
59. K. Ukai et al., “Detecting pelvic fracture on 3D-CT using deep convolutional neural networks with multi-orientated slab images,” Sci. Rep., vol. 11, no. 1, pp. 11716, 2021. doi: 10.1038/s41598-021-91144-z. [Google Scholar] [PubMed] [CrossRef]
60. L. Shen et al., “Using artificial intelligence to diagnose osteoporotic vertebral fractures on plain radiographs,” J. Bone Miner. Res., vol. 38, no. 9, pp. 1278–1287, 2023. doi: 10.1002/jbmr.4879. [Google Scholar] [PubMed] [CrossRef]
61. T. Urakawa, Y. Tanaka, S. Goto, H. Matsuzawa, K. Watanabe and N. Endo, “Detecting intertrochanteric hip fractures with orthopedist-level accuracy using a deep convolutional neural network,” Skelet. Radiol., vol. 48, pp. 239–244, 2019. doi: 10.1007/s00256-018-3016-3. [Google Scholar] [PubMed] [CrossRef]
62. P. Liu et al., “Artificial intelligence to detect the femoral intertrochanteric fracture: The arrival of the intelligent-medicine era,” Front. Bioeng. Biotechnol., vol. 10, pp. 927926, 2022. doi: 10.3389/fbioe.2022.927926. [Google Scholar] [PubMed] [CrossRef]
63. T. Kim, N. H. Moon, T. S. Goh, and I. D. Jung, “Detection of incomplete atypical femoral fracture on anteroposterior radiographs via explainable artificial intelligence,” Sci. Rep., vol. 13, no. 1, pp. 10415, 2023. doi: 10.1038/s41598-023-37560-9. [Google Scholar] [PubMed] [CrossRef]
64. J. Olczak et al., “Artificial intelligence for analyzing orthopedic trauma radiographs: Deep learning algorithms—Are they on par with humans for diagnosing fractures?,” Acta Orthop., vol. 88, no. 6, pp. 581–586, 2017. doi: 10.1080/17453674.2017.1344459. [Google Scholar] [PubMed] [CrossRef]
65. R. M. Jones et al., “Assessment of a deep-learning system for fracture detection in musculoskeletal radiographs,” npj Digit. Med., vol. 3, no. 1, pp. 144, 2020. doi: 10.1038/s41746-020-00352-w. [Google Scholar] [PubMed] [CrossRef]
66. T. Inoue et al., “Automated fracture screening using an object detection algorithm on whole-body trauma computed tomography,” Sci. Rep., vol. 12, no. 1, pp. 16549, 2022. doi: 10.1038/s41598-022-20996-w. [Google Scholar] [PubMed] [CrossRef]
67. P. Liu et al., “Artificial intelligence to diagnose tibial plateau fractures: An intelligent assistant for orthopedic physicians,” Curr. Med. Sci., vol. 41, no. 6, pp. 1158–1164, 2021. doi: 10.1007/s11596-021-2501-4. [Google Scholar] [PubMed] [CrossRef]
68. S. W. Chung et al., “Automated detection and classification of the proximal humerus fracture by using deep learning algorithm,” Acta Orthop., vol. 89, no. 4, pp. 468–473, 2018. doi: 10.1080/17453674.2018.1453714. [Google Scholar] [PubMed] [CrossRef]
69. K. Gan et al., “Artificial intelligence detection of distal radius fractures: A comparison between the convolutional neural network and professional assessments,” Acta Orthop., vol. 90, no. 4, pp. 394–400, 2019. doi: 10.1080/17453674.2019.1600125. [Google Scholar] [PubMed] [CrossRef]
70. J. W. Choi et al., “Using a dual-input convolutional neural network for automated detection of pediatric supracondylar fracture on conventional radiography,” Investig. Radiol., vol. 55, no. 2, pp. 101–110, 2020. doi: 10.1097/rli.0000000000000615. [Google Scholar] [PubMed] [CrossRef]
71. H. Z. Wu et al., “The feature ambiguity mitigate operator model helps improve bone fracture detection on X-ray radiograph,” Sci. Rep., vol. 11, no. 1, pp. 1589, 2021. doi: 10.1038/s41598-021-81236-1. [Google Scholar] [PubMed] [CrossRef]
72. C. Lee, J. Jang, S. Lee, Y. S. Kim, H. J. Jo and Y. Kim, “Classification of femur fracture in pelvic X-ray images using meta-learned deep neural network,” Sci. Rep., vol. 10, no. 1, pp. 13694, 2020. doi: 10.1038/s41598-020-70660-4. [Google Scholar] [PubMed] [CrossRef]
73. P. K. Mall and P. K. Singh, “BoostNet: A method to enhance the performance of deep learning model on musculoskeletal radiographs X-ray images,” Int. J. Syst. Assur. Eng. Manag., vol. 13, no. 1, pp. 658–672, 2022. doi: 10.1007/s13198-021-01580-3. [Google Scholar] [CrossRef]
74. J. Yang et al., “Development and validation of deep learning algorithms for scoliosis screening using back images,” Commun. Biol., vol. 2, no. 1, pp. 390, 2019. doi: 10.1038/s42003-019-0635-8. [Google Scholar] [PubMed] [CrossRef]
75. A. Thalengala, S. N. Bhat, and H. Anitha, “Computerized image understanding system for reliable estimation of spinal curvature in idiopathic scoliosis,” Sci. Rep., vol. 11, no. 1, pp. 7144, 2021. doi: 10.1038/s41598-021-86436-3. [Google Scholar] [PubMed] [CrossRef]
76. H. D. Zheng et al., “Deep learning-based high-accuracy quantitation for lumbar intervertebral disc degeneration from MRI,” Nat. Commun., vol. 13, no. 1, pp. 841, 2022. doi: 10.1038/s41467-022-28387-5. [Google Scholar] [PubMed] [CrossRef]
77. R. Jang, J. H. Choi, N. Kim, J. S. Chang, P. W. Yoon and C. H. Kim, “Prediction of osteoporosis from simple hip radiography using deep learning algorithm,” Sci. Rep., vol. 11, no. 1, pp. 19997, 2021. doi: 10.1038/s41598-021-99549-6. [Google Scholar] [PubMed] [CrossRef]
78. S. Zhang, J. Sun, C. Liu, J. H. Fang, H. T. Xie and B. Ning, “Clinical application of artificial intelligence-assisted diagnosis using anteroposterior pelvic radiographs in children with developmental dysplasia of the hip,” Bone Jt. J., vol. 102, no. 11, pp. 1574–1581, 2020. doi: 10.1302/0301-620x.102b11.bjj-2020-0712.r2. [Google Scholar] [PubMed] [CrossRef]
79. H. Den, J. Ito, and A. Kokaze, “Diagnostic accuracy of a deep learning model using YOLOv5 for detecting developmental dysplasia of the hip on radiography images,” Sci. Rep., vol. 13, no. 1, pp. 6693, 2023. doi: 10.1038/s41598-023-33860-2. [Google Scholar] [PubMed] [CrossRef]
80. Z. Merali, J. Z. Wang, J. H. Badhiwala, C. D. Witiw, J. R. Wilson and M. G. Fehlings, “A deep learning model for detection of cervical spinal cord compression in MRI scans,” Sci. Rep., vol. 11, no. 1, pp. 10473, 2021. doi: 10.1038/s41598-021-89848-3. [Google Scholar] [PubMed] [CrossRef]
81. A. Nouri, L. Tetreault, A. Singh, S. K. Karadimas, and M. G. Fehlings, “Degenerative cervical myelopathy: Epidemiology, genetics, and pathogenesis,” Spine, vol. 40, no. 12, pp. E675–E693, 2015. doi: 10.1097/brs.0000000000000913. [Google Scholar] [PubMed] [CrossRef]
82. E. Yamada et al., “A screening method for cervical myelopathy using machine learning to analyze a drawing behavior,” Sci. Rep., vol. 13, no. 1, pp. 10015, 2023. doi: 10.21203/rs.3.rs-2527781/v1. [Google Scholar] [CrossRef]
83. A. Tiulpin et al., “Multimodal machine learning-based knee osteoarthritis progression prediction from plain radiographs and clinical data,” Sci. Rep., vol. 9, no. 1, pp. 20038, 2019. doi: 10.1038/s41598-019-56527-3. [Google Scholar] [PubMed] [CrossRef]
84. M. Bany Muhammad and M. Yeasin, “Interpretable and parameter optimized ensemble model for knee osteoarthritis assessment using radiographs,” Sci. Rep., vol. 11, no. 1, pp. 14348, 2021. doi: 10.1038/s41598-021-93851-z. [Google Scholar] [PubMed] [CrossRef]
85. R. S. Hegadi, D. I. Navale, T. D. Pawar, and D. D. Ruikar, “Osteoarthritis detection and classification from knee X-ray images based on artificial neural network,” in RTIP2R 2018: Recent Trends in Image Processing and Pattern Recognition, Solapur, India, Dec. 21–22, 2018, 2019, pp. 97–105. [Google Scholar]
86. S. Raman, G. E. Gold, M. S. Rosen, and B. Sveinsson, “Automatic estimation of knee effusion from limited MRI data,” Sci. Rep., vol. 12, no. 1, pp. 3155, 2022. doi: 10.1038/s41598-022-07092-9. [Google Scholar] [PubMed] [CrossRef]
87. A. Hillmann and T. Gösling, “Gutartige Knochentumoren,” Der Unfallchirurg, vol. 117, pp. 873–882, 2014. doi: 10.1007/s00113-014-2577-4. [Google Scholar] [CrossRef]
88. K. Furuo, K. Morita, T. Hagi, T. Nakamura, and T. Wakabayashi, “Automatic benign and malignant estimation of bone tumors using deep learning,” in 2021 5th IEEE Int. Conf. on CYBCONF, 2021, pp. 030–033. [Google Scholar]
89. V. A. Georgeanu, M. Mămuleanu, S. Ghiea, and D. Selisteanu, “Malignant bone tumors diagnosis using magnetic resonance imaging based on deep learning algorithms,” Medicina, vol. 58, no. 5, pp. 636, 2022. doi: 10.3390/medicina58050636. [Google Scholar] [PubMed] [CrossRef]
90. J. Li et al., “Primary bone tumor detection and classification in full-field bone radiographs via YOLO deep learning model,” Eur. Radiol., vol. 33, no. 6, pp. 4237–4248, 2023. doi: 10.1007/s00330-022-09289-y. [Google Scholar] [PubMed] [CrossRef]
91. R. B. Interiano et al., “Initial diagnostic management of pediatric bone tumors,” J. Pediatr. Surg., vol. 51, no. 6, pp. 981–985, 2016. doi: 10.1016/j.jpedsurg.2016.02.068. [Google Scholar] [PubMed] [CrossRef]
92. Z. Xu et al., “Bone tumor necrosis rate detection in few-shot X-rays based on deep learning,” Comput. Med. Imaging Graph., vol. 102, pp. 102141, 2022. doi: 10.1016/j.compmedimag.2022.102141. [Google Scholar] [PubMed] [CrossRef]
93. Z. Soydan et al., “An AI based classifier model for lateral pillar classification of Legg-Calve–Perthes,” Sci. Rep., vol. 13, no. 1, pp. 6870, 2023. doi: 10.1038/s41598-023-34176-x. [Google Scholar] [PubMed] [CrossRef]
94. K. N. Kunze, E. M. Polce, A. Sadauskas, and B. R. Levine, “Development of machine learning algorithms to predict patient dissatisfaction after primary total knee arthroplasty,” J. Arthroplasty, vol. 35, no. 11, pp. 3117–3122, 2020. doi: 10.1016/j.arth.2020.05.061. [Google Scholar] [PubMed] [CrossRef]
95. P. N. Ramkumar et al., “Development and validation of a machine learning algorithm after primary total hip arthroplasty: Applications to length of stay and payment models,” J. Arthroplasty, vol. 34, no. 4, pp. 632–637, 2019. doi: 10.1016/j.arth.2018.12.030. [Google Scholar] [PubMed] [CrossRef]
96. M. Shah, D. R. Halalmeh, A. Sandio, R. S. Tubbs, and M. D. Moisi, “Anatomical variations that can lead to spine surgery at the wrong level: Part II thoracic spine,” Cureus, vol. 12, no. 6, pp. e8667, 2020. doi: 10.7759/cureus.8684. [Google Scholar] [PubMed] [CrossRef]
97. H. Chen et al., “Automatic localization and identification of vertebrae in spine CT via a joint learning model with deep neural networks,” in 18th Int. Conf. on MICCAI 2015, Munich, Germany, Springer International Publishing, Oct. 5–9, 2015, pp. 515–522. [Google Scholar]
98. M. Cilla, E. Borgiani, J. Martínez, G. N. Duda, and S. Checa, “Machine learning techniques for the optimization of joint replacements: Application to a short-stem hip implant,” PLoS One, vol. 12, no. 9, pp. e0183755, 2017. doi: 10.1371/journal.pone.0183755. [Google Scholar] [PubMed] [CrossRef]
99. E. Schiffner et al., “Loosening of total knee arthroplasty–Always aseptic?,” J. Clin. Orthop. Trauma., vol. 11, pp. S234–S238, 2020. doi: 10.1016/j.jcot.2019.05.001. [Google Scholar] [PubMed] [CrossRef]
100. A. Borjali, A. Chen, O. Muratoglu, M. Morid, and K. Varadarajan, “Detecting mechanical loosening of total hip arthroplasty using deep convolutional neural network,” Orthop. Proc. Bone & Joint, vol. 102, no. 1, pp. 133–133, 2020. [Google Scholar]
101. Á. Flores-Balado et al., “Using artificial intelligence to reduce orthopedic surgical site infection surveillance workload: Algorithm design, validation, and implementation in 4 Spanish hospitals,” Am. J. Infect. Control, vol. 51, no. 11, pp. 1225–1229, 2023. doi: 10.1016/j.ajic.2023.04.165. [Google Scholar] [PubMed] [CrossRef]
102. B. Goodman and S. Flaxman, “European Union regulations on algorithmic decision-making and a right to explanation,” AI Mag., vol. 38, no. 3, pp. 50–57, 2017. doi: 10.1609/aimag.v38i3.2741. [Google Scholar] [CrossRef]
103. P. E. Dupont et al., “A decade retrospective of medical robotics research from 2010 to 2020,” Sci. Robot., vol. 6, no. 60, pp. eabi8017, 2021. doi: 10.1126/scirobotics.abi8017. [Google Scholar] [PubMed] [CrossRef]
104. P. T. Nallamothu and J. P. Bharadiya, “Artificial intelligence in orthopedics: A concise review,” Asian J. of Orthop. Research, vol. 9, no. 1, pp. 17–27, 2023. [Google Scholar]
105. Y. S. Kwoh, J. Hou, E. A. Jonckheere, and S. Hayati, “A robot with improved absolute positioning accuracy for CT guided stereotactic brain surgery,” IEEE. Trans. Biomed. Eng., vol. 35, no. 2, pp. 153–160, 1988. doi: 10.1109/10.1354. [Google Scholar] [PubMed] [CrossRef]
106. D. Mirić, B. Karović, and K. Senohradski, “Role of wrist instability in the onset of pseudoarthrosis of the scaphoid bone,” Srp. Ark. Celok. Lek., vol. 128, no. 11–12, pp. 384–388, 2000. [Google Scholar]
107. Q. Wang, M. Shuai, G. Xiong, and H. Jia, “A cadaveric feasibility study of an automatic multi-degrees of freedom surgical robot for percutaneous scaphoid guide pin insertion,” J. Hand. Surg. (Asian-Pacific Volume), vol. 28, no. 4, pp. 461–466, 2023. doi: 10.1142/s2424835523500479. [Google Scholar] [PubMed] [CrossRef]
108. C. Xiao et al., “Robot-assisted vs traditional percutaneous freehand for the scaphoid fracture treatment: A retrospective study,” Int. Orthop., vol. 47, no. 3, pp. 839–845, 2023. doi: 10.1007/s00264-022-05532-9. [Google Scholar] [PubMed] [CrossRef]
109. C. Wang et al., “Robot-assisted percutaneous screw fixation in the treatment of navicular fracture,” Front. Surg., vol. 9, pp. 1049455, 2023. doi: 10.3389/fsurg.2022.1049455. [Google Scholar] [PubMed] [CrossRef]
110. N. Hawi et al., “Navigated femoral shaft fracture treatment: Current status,” Comput. Aided Surg., vol. 13, no. 3, pp. 148–156, 2008. doi: 10.3233/thc-2011-0652. [Google Scholar] [PubMed] [CrossRef]
111. Y. Maeda et al., “Robot-assisted femoral fracture reduction: Preliminary study in patients and healthy volunteers,” Comput. Aided Surg., vol. 13, no. 3, pp. 148–156, 2008. doi: 10.3109/10929080802031038. [Google Scholar] [PubMed] [CrossRef]
112. R. Ye, Y. Chen, and W. Yau, “A simple and novel hybrid robotic system for robot-assisted femur fracture reduction,” Adv Robotics, vol. 26, no. 1–2, pp. 83–104, 2012. doi: 10.1163/016918611x607383. [Google Scholar] [CrossRef]
113. J. N. Insall et al., “Total knee arthroplasty,” Clin. Orthop. Relat. Res., vol. 192, pp. 13–22, 1985. doi: 10.1097/00003086-198501000-00003. [Google Scholar] [CrossRef]
114. F. S. Hossain, S. Konan, S. Patel, S. Rodriguez-Merchan, and F. Haddad, “The assessment of outcome after total knee arthroplasty: Are we there yet?,” Bone Jt. J., vol. 97, no. 1, pp. 3–9, 2015. doi: 10.1302/0301-620X.97B1.34434. [Google Scholar] [PubMed] [CrossRef]
115. M. Jakopec, F. R. Y. Baena, S. J. Harris, P. Gomes, J. Cobb and B. L. Davies, “The hands-on orthopedic robot “Acrobot”: Early clinical trials of total knee replacement surgery,” IEEE Trans. Robot. Autom., vol. 19, no. 5, pp. 902–911, 2003. doi: 10.1109/TRA.2003.817510. [Google Scholar] [CrossRef]
116. W. Siebert, S. Mai, R. Kober, and P. F. Heeckt, “Technique and first clinical results of robot-assisted total knee replacement,” The Knee, vol. 9, no. 3, pp. 173–180, 2002. doi: 10.1016/s0968-0160(02)00015-7. [Google Scholar] [PubMed] [CrossRef]
117. J. Cobb et al., “Hands-on robotic unicompartmental knee replacement: A prospective, randomised controlled study of the acrobot system,” J. Bone Joint Surg. Br., vol. 88, no. 2, pp. 188–197, 2006. doi: 10.1302/0301-620x.88b2.17220. [Google Scholar] [PubMed] [CrossRef]
118. R. Roy-Camille, G. Saillant, and C. Mazel, “Internal fixation of the lumbar spine with pedicle screw plating,” Clin. Orthop. Relat. Res., vol. 203, pp. 7–17, 1986. doi: 10.1097/00003086-198602000-00003. [Google Scholar] [CrossRef]
119. J. M. Hicks, A. Singla, F. H. Shen, and V. Arlet, “Complications of pedicle screw fixation in scoliosis surgery: A systematic review,” Spine, vol. 35, no. 11, pp. E465–E470, 2010. doi: 10.1097/brs.0b013e3181d1021a. [Google Scholar] [PubMed] [CrossRef]
120. K. Ponnusamy, S. Chewning, and C. Mohr, “Robotic approaches to the posterior spine,” Spine, vol. 34, no. 19, pp. 2104–2109, 2009. doi: 10.1097/brs.0b013e3181b20212. [Google Scholar] [PubMed] [CrossRef]
121. M. R. Onen, M. Simsek, and S. Naderi, “Robotic spine surgery: A preliminary report,” Turk. Neurosurg., vol. 24, no. 4, pp. 512–518, 2014. doi: 10.5137/1019-5149.jtn.8951-13.1. [Google Scholar] [PubMed] [CrossRef]
122. Y. Lin, “Application of semi-automatic spinal surgery robot system in spinal surgery,” Chinese J. Tissue Eng. Res., vol. 24, no. 24, pp. 3792, 2020. doi: 10.3969/j.issn.2095-4344.2745. [Google Scholar] [CrossRef]
123. Z. Li et al., “Collaborative spinal robot system for laminectomy: A preliminary study,” Neurosurg. Focus, vol. 52, no. 1, pp. E11, 2022. doi: 10.3171/2021.10.focus21499. [Google Scholar] [PubMed] [CrossRef]
124. D. Gonzalez, S. Ghessese, D. Cook, and D. Hedequist, “Initial intraoperative experience with robotic-assisted pedicle screw placement with stealth navigation in pediatric spine deformity: An evaluation of the first 40 cases,” J. Robot. Surg., vol. 15, pp. 687–693, 2021. doi: 10.1007/s11701-020-01159-3. [Google Scholar] [PubMed] [CrossRef]
125. A. S. Narain et al., “Patient perceptions of minimally invasive versus open spine surgery,” Clin. Spine Surg., vol. 31, no. 3, pp. E184–E192, 2018. doi: 10.1097/bsd.0000000000000618. [Google Scholar] [PubMed] [CrossRef]
126. B. Jiang et al., “Robot-assisted versus freehand instrumentation in short-segment lumbar fusion: Experience with real-time image-guided spinal robot,” World Neurosurg., vol. 136, pp. e635–e645, 2020. doi: 10.1016/j.wneu.2020.01.119. [Google Scholar] [PubMed] [CrossRef]
127. Q. Zhu, B. Liang, X. Wang, X. Sun, and L. Wang, “Minimally invasive treatment of displaced femoral shaft fractures with a teleoperated robot-assisted surgical system,” Injury, vol. 48, no. 10, pp. 2253–2259, 2017. doi: 10.1016/j.injury.2017.07.014. [Google Scholar] [PubMed] [CrossRef]
128. S. J. Federer and G. G. Jones, “Artificial intelligence in orthopedics: A scoping review,” PLoS One, vol. 16, no. 11, pp. e0260471, 2021. doi: 10.1371/journal.pone.0260471. [Google Scholar] [PubMed] [CrossRef]
129. M. Poduval, A. Ghose, S. Manchanda, V. Bagaria, and A. Sinha, “Artificial intelligence and machine learning: A new disruptive force in orthopedics,” Indian J. Orthop., vol. 54, pp. 109–122, 2020. doi: 10.1007/s43465-019-00023-3. [Google Scholar] [PubMed] [CrossRef]
130. J. H. Lee and S. W. Chung, “Deep learning for orthopedic disease based on medical image analysis: Present and future,” Appl. Sci., vol. 12, no. 2, pp. 681, 2022. doi: 10.3390/app12020681. [Google Scholar] [CrossRef]
131. S. P. Lalehzarian, A. K. Gowd, and J. N. Liu, “Machine learning in orthopedic surgery,” World J. Orthop., vol. 12, no. 9, pp. 685, 2021. doi: 10.5312/wjo.v12.i9.685. [Google Scholar] [PubMed] [CrossRef]
132. Y. Wang, R. Li, and P. Zheng, “Progress in clinical application of artificial intelligence in orthopedics,” Digit. Med., vol. 8, no. 1, pp. 4, 2022. doi: 10.4103/digm.digm_10_21. [Google Scholar] [CrossRef]
133. H. S. Kalmet et al., “Deep learning in fracture detection: A narrative review,” Acta Orthop., vol. 91, no. 2, pp. 215–220, 2020. doi: 10.1080/17453674.2019.1711323. [Google Scholar] [PubMed] [CrossRef]
134. E. Justaniah, G. Aldabbagh, A. Alhothali, and N. Abourokbah, “Classifying breast density from mammogram with pretrained CNNs and weighted average ensembles,” Appl. Sci., vol. 12, no. 11, pp. 5599, 2022. doi: 10.3390/app12115599. [Google Scholar] [CrossRef]
135. N. Farzaneh, S. Ansari, E. Lee, K. R. Ward, and M. W. Sjoding, “Collaborative strategies for deploying artificial intelligence to complement physician diagnoses of acute respiratory distress syndrome,” npj Digit. Med., vol. 6, no. 1, pp. 62, 2023. doi: 10.1038/s41746-023-00797-9. [Google Scholar] [PubMed] [CrossRef]
136. R. C. Marchand et al., “Patient satisfaction outcomes after robotic arm-assisted total knee arthroplasty: A short-term evaluation,” J. Knee Surg., vol. 30, no. 9, pp. 849–853, 2017. doi: 10.1055/s-0037-1607450. [Google Scholar] [PubMed] [CrossRef]
137. A. F. Smith et al., “Improved patient satisfaction following robotic-assisted total knee arthroplasty,” J. Knee Surg., vol. 34, no. 7, pp. 730–738, 2019. doi: 10.1055/s-0039-1700837. [Google Scholar] [PubMed] [CrossRef]
138. H. Zhang, Y. Yu, J. Jiao, E. P. Xing, L. E. Ghaoui and M. I. Jordan, “Theoretically principled trade-off between robustness and accuracy,” in Int. Conf. Mach. Learn., 2019, pp. 7472–7482. [Google Scholar]
139. T. Pang, M. Lin, X. Yang, J. Zhu, and S. Yan, “Robustness and accuracy could be reconcilable by (proper) definition,” in Int. Conf. Mach. Learn., 2022, pp. 17258–17277. doi: 10.48550/arxiv.2202.10103. [Google Scholar] [CrossRef]
Cite This Article
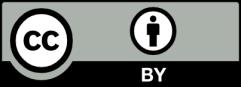
This work is licensed under a Creative Commons Attribution 4.0 International License , which permits unrestricted use, distribution, and reproduction in any medium, provided the original work is properly cited.