Open Access
ARTICLE
CVTD: A Robust Car-Mounted Video Text Detector
1 Department of Computer Science and Engineering, Chongqing University of Technology, Chongqing, China
2 College of Information and Engineering, Jingdezhen Ceramic University, Jingdezhen, China
* Corresponding Author: Jianxun Zhang. Email:
Computers, Materials & Continua 2024, 78(2), 1821-1842. https://doi.org/10.32604/cmc.2023.047236
Received 30 October 2023; Accepted 11 December 2023; Issue published 27 February 2024
Abstract
Text perception is crucial for understanding the semantics of outdoor scenes, making it a key requirement for building intelligent systems for driver assistance or autonomous driving. Text information in car-mounted videos can assist drivers in making decisions. However, Car-mounted video text images pose challenges such as complex backgrounds, small fonts, and the need for real-time detection. We proposed a robust Car-mounted Video Text Detector (CVTD). It is a lightweight text detection model based on ResNet18 for feature extraction, capable of detecting text in arbitrary shapes. Our model efficiently extracted global text positions through the Coordinate Attention Threshold Activation (CATA) and enhanced the representation capability through stacking two Feature Pyramid Enhancement Fusion Modules (FPEFM), strengthening feature representation, and integrating text local features and global position information, reinforcing the representation capability of the CVTD model. The enhanced feature maps, when acted upon by Text Activation Maps (TAM), effectively distinguished text foreground from non-text regions. Additionally, we collected and annotated a dataset containing 2200 images of Car-mounted Video Text (CVT) under various road conditions for training and evaluating our model’s performance. We further tested our model on four other challenging public natural scene text detection benchmark datasets, demonstrating its strong generalization ability and real-time detection speed. This model holds potential for practical applications in real-world scenarios. The code is publicly available at: .Keywords
Cite This Article
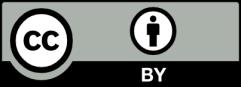