Open Access
ARTICLE
Enhancing Multicriteria-Based Recommendations by Alleviating Scalability and Sparsity Issues Using Collaborative Denoising Autoencoder
School of Computer Science & Engineering, Vellore Institute of Technology, Chennai, Tamilnadu, 600 127, India
* Corresponding Author: S. Abinaya. Email:
Computers, Materials & Continua 2024, 78(2), 2269-2286. https://doi.org/10.32604/cmc.2024.047167
Received 27 October 2023; Accepted 05 December 2023; Issue published 27 February 2024
Abstract
A Recommender System (RS) is a crucial part of several firms, particularly those involved in e-commerce. In conventional RS, a user may only offer a single rating for an item-that is insufficient to perceive consumer preferences. Nowadays, businesses in industries like e-learning and tourism enable customers to rate a product using a variety of factors to comprehend customers’ preferences. On the other hand, the collaborative filtering (CF) algorithm utilizing AutoEncoder (AE) is seen to be effective in identifying user-interested items. However, the cost of these computations increases nonlinearly as the number of items and users increases. To triumph over the issues, a novel expanded stacked autoencoder (ESAE) with Kernel Fuzzy C-Means Clustering (KFCM) technique is proposed with two phases. In the first phase of offline, the sparse multicriteria rating matrix is smoothened to a complete matrix by predicting the users’ intact rating by the ESAE approach and users are clustered using the KFCM approach. In the next phase of online, the top-N recommendation prediction is made by the ESAE approach involving only the most similar user from multiple clusters. Hence the ESAE_KFCM model upgrades the prediction accuracy of 98.2% in Top-N recommendation with a minimized recommendation generation time. An experimental check on the Yahoo! Movies (YM) movie dataset and TripAdvisor (TA) travel dataset confirmed that the ESAE_KFCM model constantly outperforms conventional RS algorithms on a variety of assessment measures.Keywords
Cite This Article
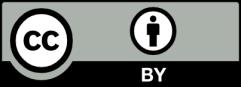
This work is licensed under a Creative Commons Attribution 4.0 International License , which permits unrestricted use, distribution, and reproduction in any medium, provided the original work is properly cited.