Open Access
ARTICLE
Social Robot Detection Method with Improved Graph Neural Networks
Institute of Systems Security and Control, College of Computer Science and Technology, Xi’an University of Science and Technology, Xi’an, 710054, China
* Corresponding Author: Ou Ye. Email:
Computers, Materials & Continua 2024, 78(2), 1773-1795. https://doi.org/10.32604/cmc.2023.047130
Received 26 October 2023; Accepted 15 December 2023; Issue published 27 February 2024
Abstract
Social robot accounts controlled by artificial intelligence or humans are active in social networks, bringing negative impacts to network security and social life. Existing social robot detection methods based on graph neural networks suffer from the problem of many social network nodes and complex relationships, which makes it difficult to accurately describe the difference between the topological relations of nodes, resulting in low detection accuracy of social robots. This paper proposes a social robot detection method with the use of an improved neural network. First, social relationship subgraphs are constructed by leveraging the user’s social network to disentangle intricate social relationships effectively. Then, a linear modulated graph attention residual network model is devised to extract the node and network topology features of the social relation subgraph, thereby generating comprehensive social relation subgraph features, and the feature-wise linear modulation module of the model can better learn the differences between the nodes. Next, user text content and behavioral gene sequences are extracted to construct social behavioral features combined with the social relationship subgraph features. Finally, social robots can be more accurately identified by combining user behavioral and relationship features. By carrying out experimental studies based on the publicly available datasets TwiBot-20 and Cresci-15, the suggested method’s detection accuracies can achieve 86.73% and 97.86%, respectively. Compared with the existing mainstream approaches, the accuracy of the proposed method is 2.2% and 1.35% higher on the two datasets. The results show that the method proposed in this paper can effectively detect social robots and maintain a healthy ecological environment of social networks.Keywords
Cite This Article
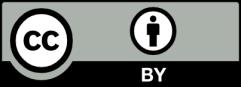