Open Access
ARTICLE
DGConv: A Novel Convolutional Neural Network Approach for Weld Seam Depth Image Detection
1 State Key Laboratory of Robotics, Shenyang Institute of Automation, Chinese Academy of Sciences, Shenyang, 110016, China
2 Institutes for Robotics and Intelligent Manufacturing, Chinese Academy of Sciences, Shenyang, 110169, China
3 University of Chinese Academy of Sciences, Beijing, 100049, China
4 Shenyang SIASUN Robot & Automation Co., Ltd., Shenyang, 110168, China
* Corresponding Author: Pengchao Li. Email:
Computers, Materials & Continua 2024, 78(2), 1755-1771. https://doi.org/10.32604/cmc.2023.047057
Received 23 October 2023; Accepted 08 December 2023; Issue published 27 February 2024
Abstract
We propose a novel image segmentation algorithm to tackle the challenge of limited recognition and segmentation performance in identifying welding seam images during robotic intelligent operations. Initially, to enhance the capability of deep neural networks in extracting geometric attributes from depth images, we developed a novel deep geometric convolution operator (DGConv). DGConv is utilized to construct a deep local geometric feature extraction module, facilitating a more comprehensive exploration of the intrinsic geometric information within depth images. Secondly, we integrate the newly proposed deep geometric feature module with the Fully Convolutional Network (FCN8) to establish a high-performance deep neural network algorithm tailored for depth image segmentation. Concurrently, we enhance the FCN8 detection head by separating the segmentation and classification processes. This enhancement significantly boosts the network’s overall detection capability. Thirdly, for a comprehensive assessment of our proposed algorithm and its applicability in real-world industrial settings, we curated a line-scan image dataset featuring weld seams. This dataset, named the Standardized Linear Depth Profile (SLDP) dataset, was collected from actual industrial sites where autonomous robots are in operation. Ultimately, we conducted experiments utilizing the SLDP dataset, achieving an average accuracy of 92.7%. Our proposed approach exhibited a remarkable performance improvement over the prior method on the identical dataset. Moreover, we have successfully deployed the proposed algorithm in genuine industrial environments, fulfilling the prerequisites of unmanned robot operations.Keywords
Cite This Article
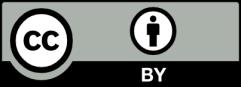
This work is licensed under a Creative Commons Attribution 4.0 International License , which permits unrestricted use, distribution, and reproduction in any medium, provided the original work is properly cited.