Open Access
ARTICLE
A Normalizing Flow-Based Bidirectional Mapping Residual Network for Unsupervised Defect Detection
1 School of Mechanical Engineering, Guizhou University, Guiyang, 550025, China
2 School of Computer Science and Engineering, Central South University, Changsha, 410083, China
3 School of Computer and Information Technology, Beijing Jiaotong University, Beijing, 100044, China
4 College of Civil Engineering, Guizhou University, Guiyang, 550025, China
5 Guizhou Lianjian Civil Engineering Quality Inspection Monitoring Center Co., Ltd., Guiyang, 550025, China
* Corresponding Author: Linna Zhang. Email:
Computers, Materials & Continua 2024, 78(2), 1631-1648. https://doi.org/10.32604/cmc.2024.046924
Received 19 October 2023; Accepted 30 November 2023; Issue published 27 February 2024
Abstract
Unsupervised methods based on density representation have shown their abilities in anomaly detection, but detection performance still needs to be improved. Specifically, approaches using normalizing flows can accurately evaluate sample distributions, mapping normal features to the normal distribution and anomalous features outside it. Consequently, this paper proposes a Normalizing Flow-based Bidirectional Mapping Residual Network (NF-BMR). It utilizes pre-trained Convolutional Neural Networks (CNN) and normalizing flows to construct discriminative source and target domain feature spaces. Additionally, to better learn feature information in both domain spaces, we propose the Bidirectional Mapping Residual Network (BMR), which maps sample features to these two spaces for anomaly detection. The two detection spaces effectively complement each other’s deficiencies and provide a comprehensive feature evaluation from two perspectives, which leads to the improvement of detection performance. Comparative experimental results on the MVTec AD and DAGM datasets against the Bidirectional Pre-trained Feature Mapping Network (B-PFM) and other state-of-the-art methods demonstrate that the proposed approach achieves superior performance. On the MVTec AD dataset, NF-BMR achieves an average AUROC of 98.7% for all 15 categories. Especially, it achieves 100% optimal detection performance in five categories. On the DAGM dataset, the average AUROC across ten categories is 98.7%, which is very close to supervised methods.Graphic Abstract
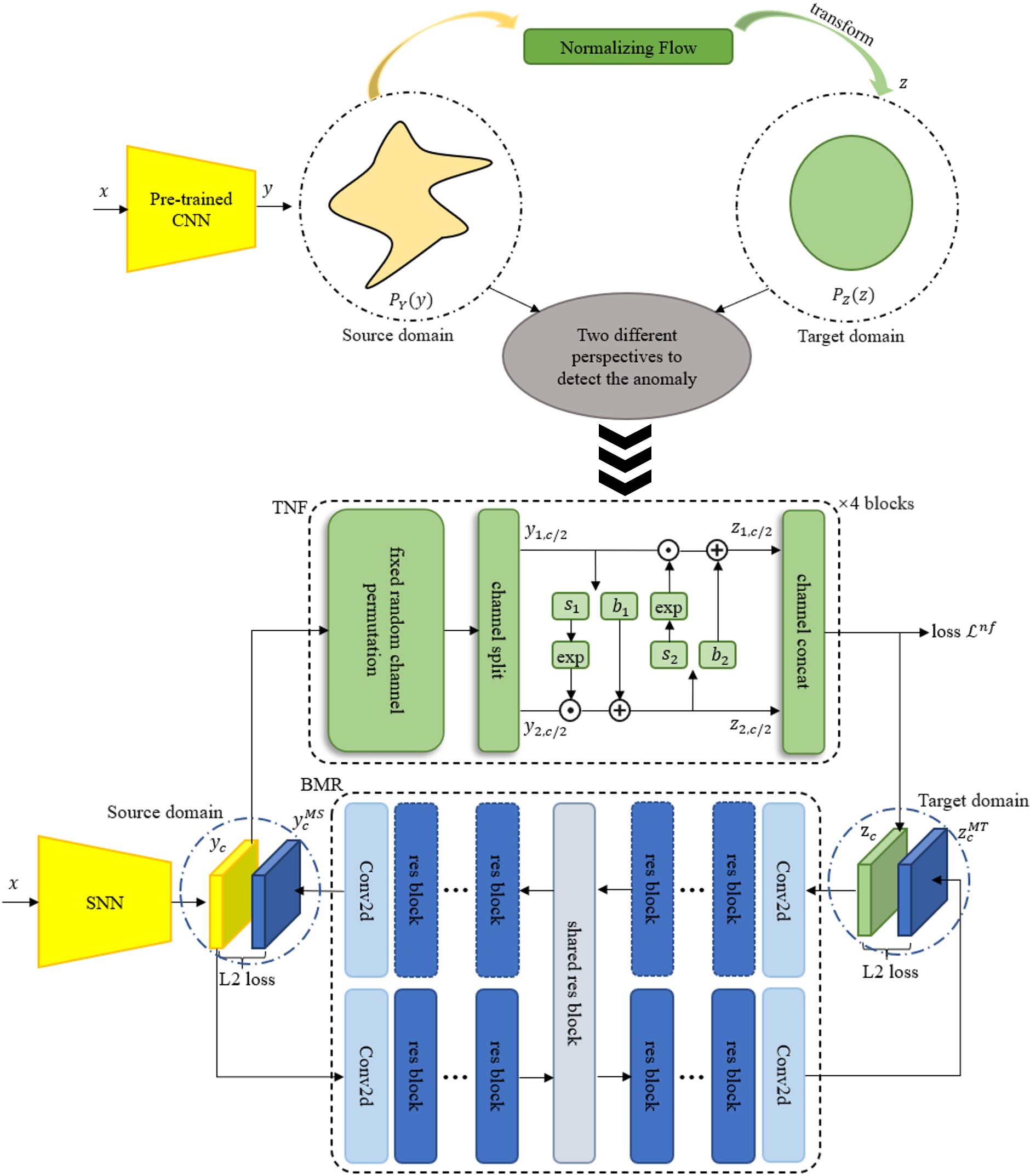
Keywords
Cite This Article
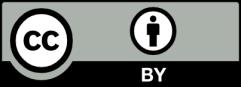
This work is licensed under a Creative Commons Attribution 4.0 International License , which permits unrestricted use, distribution, and reproduction in any medium, provided the original work is properly cited.