Open Access
ARTICLE
Personality Trait Detection via Transfer Learning
1 Department of Information Technologies and Systems, University of Castilla-La Mancha, Ciudad Real, 13071, Spain
2 Institute of Artificial Intelligence, School of Computer Science and Informatics, De Montfort University, The Gateway, Leicester, LE1 9BH, UK
* Corresponding Author: Jesus Serrano-Guerrero. Email:
(This article belongs to the Special Issue: Transfroming from Data to Knowledge and Applications in Intelligent Systems)
Computers, Materials & Continua 2024, 78(2), 1933-1956. https://doi.org/10.32604/cmc.2023.046711
Received 12 October 2023; Accepted 18 December 2023; Issue published 27 February 2024
Abstract
Personality recognition plays a pivotal role when developing user-centric solutions such as recommender systems or decision support systems across various domains, including education, e-commerce, or human resources. Traditional machine learning techniques have been broadly employed for personality trait identification; nevertheless, the development of new technologies based on deep learning has led to new opportunities to improve their performance. This study focuses on the capabilities of pre-trained language models such as BERT, RoBERTa, ALBERT, ELECTRA, ERNIE, or XLNet, to deal with the task of personality recognition. These models are able to capture structural features from textual content and comprehend a multitude of language facets and complex features such as hierarchical relationships or long-term dependencies. This makes them suitable to classify multi-label personality traits from reviews while mitigating computational costs. The focus of this approach centers on developing an architecture based on different layers able to capture the semantic context and structural features from texts. Moreover, it is able to fine-tune the previous models using the MyPersonality dataset, which comprises 9,917 status updates contributed by 250 Facebook users. These status updates are categorized according to the well-known Big Five personality model, setting the stage for a comprehensive exploration of personality traits. To test the proposal, a set of experiments have been performed using different metrics such as the exact match ratio, hamming loss, zero-one-loss, precision, recall, F1-score, and weighted averages. The results reveal ERNIE is the top-performing model, achieving an exact match ratio of 72.32%, an accuracy rate of 87.17%, and 84.41% of F1-score. The findings demonstrate that the tested models substantially outperform other state-of-the-art studies, enhancing the accuracy by at least 3% and confirming them as powerful tools for personality recognition. These findings represent substantial advancements in personality recognition, making them appropriate for the development of user-centric applications.Keywords
Cite This Article
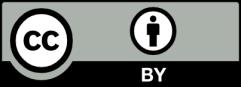
This work is licensed under a Creative Commons Attribution 4.0 International License , which permits unrestricted use, distribution, and reproduction in any medium, provided the original work is properly cited.