Open Access
ARTICLE
Enhanced Wolf Pack Algorithm (EWPA) and Dense-kUNet Segmentation for Arterial Calcifications in Mammograms
College of Computing and Information Technology, Shaqra University, Shaqra, 11961, Saudi Arabia
* Corresponding Author: Afnan M. Alhassan. Email:
(This article belongs to the Special Issue: Deep Learning in Medical Imaging-Disease Segmentation and Classification)
Computers, Materials & Continua 2024, 78(2), 2207-2223. https://doi.org/10.32604/cmc.2024.046427
Received 30 September 2023; Accepted 03 January 2024; Issue published 27 February 2024
Abstract
Breast Arterial Calcification (BAC) is a mammographic decision dissimilar to cancer and commonly observed in elderly women. Thus identifying BAC could provide an expense, and be inaccurate. Recently Deep Learning (DL) methods have been introduced for automatic BAC detection and quantification with increased accuracy. Previously, classification with deep learning had reached higher efficiency, but designing the structure of DL proved to be an extremely challenging task due to overfitting models. It also is not able to capture the patterns and irregularities presented in the images. To solve the overfitting problem, an optimal feature set has been formed by Enhanced Wolf Pack Algorithm (EWPA), and their irregularities are identified by Dense-kUNet segmentation. In this paper, Dense-kUNet for segmentation and optimal feature has been introduced for classification (severe, mild, light) that integrates DenseUNet and kU-Net. Longer bound links exist among adjacent modules, allowing relatively rough data to be sent to the following component and assisting the system in finding higher qualities. The major contribution of the work is to design the best features selected by Enhanced Wolf Pack Algorithm (EWPA), and Modified Support Vector Machine (MSVM) based learning for classification. k-Dense-UNet is introduced which combines the procedure of Dense-UNet and kU-Net for image segmentation. Longer bound associations occur among nearby sections, allowing relatively granular data to be sent to the next subsystem and benefiting the system in recognizing smaller characteristics. The proposed techniques and the performance are tested using several types of analysis techniques 826 filled digitized mammography. The proposed method achieved the highest precision, recall, F-measure, and accuracy of 84.4333%, 84.5333%, 84.4833%, and 86.8667% when compared to other methods on the Digital Database for Screening Mammography (DDSM).Keywords
Cite This Article
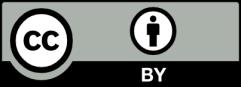
This work is licensed under a Creative Commons Attribution 4.0 International License , which permits unrestricted use, distribution, and reproduction in any medium, provided the original work is properly cited.