Open Access
ARTICLE
Weighted Forwarding in Graph Convolution Networks for Recommendation Information Systems
Department of Computer Science, Kyonggi University, Suwon, Korea
* Corresponding Author: Namgi Kim. Email:
Computers, Materials & Continua 2024, 78(2), 1897-1914. https://doi.org/10.32604/cmc.2023.046346
Received 27 September 2023; Accepted 21 December 2023; Issue published 27 February 2024
Abstract
Recommendation Information Systems (RIS) are pivotal in helping users in swiftly locating desired content from the vast amount of information available on the Internet. Graph Convolution Network (GCN) algorithms have been employed to implement the RIS efficiently. However, the GCN algorithm faces limitations in terms of performance enhancement owing to the due to the embedding value-vanishing problem that occurs during the learning process. To address this issue, we propose a Weighted Forwarding method using the GCN (WF-GCN) algorithm. The proposed method involves multiplying the embedding results with different weights for each hop layer during graph learning. By applying the WF-GCN algorithm, which adjusts weights for each hop layer before forwarding to the next, nodes with many neighbors achieve higher embedding values. This approach facilitates the learning of more hop layers within the GCN framework. The efficacy of the WF-GCN was demonstrated through its application to various datasets. In the MovieLens dataset, the implementation of WF-GCN in LightGCN resulted in significant performance improvements, with recall and NDCG increasing by up to +163.64% and +132.04%, respectively. Similarly, in the Last.FM dataset, LightGCN using WF-GCN enhanced with WF-GCN showed substantial improvements, with the recall and NDCG metrics rising by up to +174.40% and +169.95%, respectively. Furthermore, the application of WF-GCN to Self-supervised Graph Learning (SGL) and Simple Graph Contrastive Learning (SimGCL) also demonstrated notable enhancements in both recall and NDCG across these datasets.Keywords
Cite This Article
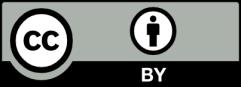