Open Access
ARTICLE
Defect Detection Model Using Time Series Data Augmentation and Transformation
1 Department of Computer Science, Kyonggi University, Suwon-si, 16227, Korea
2 Contents Convergence Software Research Institue, Kyonggi University, Suwon-si, 16227, Korea
3 Department of Energy IT Engineering, Far East University, Eumsung-gun, 27601, Korea
4 Division of AI Computer Science and Engineering, Kyonggi University, Suwon-si, 16227, Korea
* Corresponding Author: Kyungyong Chung. Email:
Computers, Materials & Continua 2024, 78(2), 1713-1730. https://doi.org/10.32604/cmc.2023.046324
Received 26 September 2023; Accepted 15 December 2023; Issue published 27 February 2024
Abstract
Time-series data provide important information in many fields, and their processing and analysis have been the focus of much research. However, detecting anomalies is very difficult due to data imbalance, temporal dependence, and noise. Therefore, methodologies for data augmentation and conversion of time series data into images for analysis have been studied. This paper proposes a fault detection model that uses time series data augmentation and transformation to address the problems of data imbalance, temporal dependence, and robustness to noise. The method of data augmentation is set as the addition of noise. It involves adding Gaussian noise, with the noise level set to 0.002, to maximize the generalization performance of the model. In addition, we use the Markov Transition Field (MTF) method to effectively visualize the dynamic transitions of the data while converting the time series data into images. It enables the identification of patterns in time series data and assists in capturing the sequential dependencies of the data. For anomaly detection, the PatchCore model is applied to show excellent performance, and the detected anomaly areas are represented as heat maps. It allows for the detection of anomalies, and by applying an anomaly map to the original image, it is possible to capture the areas where anomalies occur. The performance evaluation shows that both F1-score and Accuracy are high when time series data is converted to images. Additionally, when processed as images rather than as time series data, there was a significant reduction in both the size of the data and the training time. The proposed method can provide an important springboard for research in the field of anomaly detection using time series data. Besides, it helps solve problems such as analyzing complex patterns in data lightweight.Keywords
Cite This Article
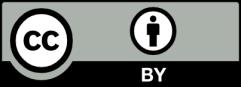
This work is licensed under a Creative Commons Attribution 4.0 International License , which permits unrestricted use, distribution, and reproduction in any medium, provided the original work is properly cited.