Open Access
ARTICLE
Machine Learning Security Defense Algorithms Based on Metadata Correlation Features
College of Computer Science, Sichuan University, Chengdu, 610065, China
* Corresponding Author: Jianwei Zhang. Email:
(This article belongs to the Special Issue: Advanced Data Mining Techniques: Security, Intelligent Systems and Applications)
Computers, Materials & Continua 2024, 78(2), 2391-2418. https://doi.org/10.32604/cmc.2024.044149
Received 22 July 2023; Accepted 25 December 2023; Issue published 27 February 2024
Abstract
With the popularization of the Internet and the development of technology, cyber threats are increasing day by day. Threats such as malware, hacking, and data breaches have had a serious impact on cybersecurity. The network security environment in the era of big data presents the characteristics of large amounts of data, high diversity, and high real-time requirements. Traditional security defense methods and tools have been unable to cope with the complex and changing network security threats. This paper proposes a machine-learning security defense algorithm based on metadata association features. Emphasize control over unauthorized users through privacy, integrity, and availability. The user model is established and the mapping between the user model and the metadata of the data source is generated. By analyzing the user model and its corresponding mapping relationship, the query of the user model can be decomposed into the query of various heterogeneous data sources, and the integration of heterogeneous data sources based on the metadata association characteristics can be realized. Define and classify customer information, automatically identify and perceive sensitive data, build a behavior audit and analysis platform, analyze user behavior trajectories, and complete the construction of a machine learning customer information security defense system. The experimental results show that when the data volume is 5 × 103 bit, the data storage integrity of the proposed method is 92%. The data accuracy is 98%, and the success rate of data intrusion is only 2.6%. It can be concluded that the data storage method in this paper is safe, the data accuracy is always at a high level, and the data disaster recovery performance is good. This method can effectively resist data intrusion and has high air traffic control security. It can not only detect all viruses in user data storage, but also realize integrated virus processing, and further optimize the security defense effect of user big data.Keywords
Cite This Article
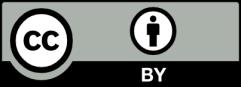
This work is licensed under a Creative Commons Attribution 4.0 International License , which permits unrestricted use, distribution, and reproduction in any medium, provided the original work is properly cited.