Open Access
ARTICLE
Multi-Perspective Data Fusion Framework Based on Hierarchical BERT: Provide Visual Predictions of Business Processes
1 School of Mathematics and Big Data, Anhui University of Science and Technology, Huainan, 232001, China
2 School of Economics and Management, Anhui University of Science and Technology, Huainan, 232001, China
3 The Key Laboratory of Embedded System and Service Computing Ministry of Education, Tongji University, Shanghai, 201804, China
* Corresponding Author: Xiangwei Liu. Email:
Computers, Materials & Continua 2024, 78(1), 1227-1252. https://doi.org/10.32604/cmc.2023.046937
Received 19 October 2023; Accepted 13 December 2023; Issue published 30 January 2024
Abstract
Predictive Business Process Monitoring (PBPM) is a significant research area in Business Process Management (BPM) aimed at accurately forecasting future behavioral events. At present, deep learning methods are widely cited in PBPM research, but no method has been effective in fusing data information into the control flow for multi-perspective process prediction. Therefore, this paper proposes a process prediction method based on the hierarchical BERT and multi-perspective data fusion. Firstly, the first layer BERT network learns the correlations between different category attribute data. Then, the attribute data is integrated into a weighted event-level feature vector and input into the second layer BERT network to learn the impact and priority relationship of each event on future predicted events. Next, the multi-head attention mechanism within the framework is visualized for analysis, helping to understand the decision-making logic of the framework and providing visual predictions. Finally, experimental results show that the predictive accuracy of the framework surpasses the current state-of-the-art research methods and significantly enhances the predictive performance of BPM.Keywords
Cite This Article
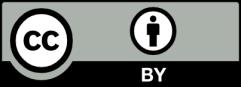
This work is licensed under a Creative Commons Attribution 4.0 International License , which permits unrestricted use, distribution, and reproduction in any medium, provided the original work is properly cited.