Open Access
ARTICLE
Leveraging Augmented Reality, Semantic-Segmentation, and VANETs for Enhanced Driver’s Safety Assistance
1 Mixed Reality and Interaction Lab, Department of Software, Sejong University, Seoul, 05006, Korea
2 Faculty of Engineering and Digital Technologies, School of Computer Science, AI and Electronics, University of Bradford, Bradford, UK
* Corresponding Author: Jong Weon Lee. Email:
(This article belongs to the Special Issue: Deep Learning based Object Detection and Tracking in Videos)
Computers, Materials & Continua 2024, 78(1), 1443-1460. https://doi.org/10.32604/cmc.2023.046707
Received 11 October 2023; Accepted 28 November 2023; Issue published 30 January 2024
Abstract
Overtaking is a crucial maneuver in road transportation that requires a clear view of the road ahead. However, limited visibility of ahead vehicles can often make it challenging for drivers to assess the safety of overtaking maneuvers, leading to accidents and fatalities. In this paper, we consider atrous convolution, a powerful tool for explicitly adjusting the field-of-view of a filter as well as controlling the resolution of feature responses generated by Deep Convolutional Neural Networks in the context of semantic image segmentation. This article explores the potential of seeing-through vehicles as a solution to enhance overtaking safety. See-through vehicles leverage advanced technologies such as cameras, sensors, and displays to provide drivers with a real-time view of the vehicle ahead, including the areas hidden from their direct line of sight. To address the problems of safe passing and occlusion by huge vehicles, we designed a see-through vehicle system in this study, we employed a windshield display in the back car together with cameras in both cars. The server within the back car was used to segment the car, and the segmented portion of the car displayed the video from the front car. Our see-through system improves the driver’s field of vision and helps him change lanes, cross a large car that is blocking their view, and safely overtake other vehicles. Our network was trained and tested on the Cityscape dataset using semantic segmentation. This transparent technique will instruct the driver on the concealed traffic situation that the front vehicle has obscured. For our findings, we have achieved 97.1% F1-score. The article also discusses the challenges and opportunities of implementing see-through vehicles in real-world scenarios, including technical, regulatory, and user acceptance factors.Keywords
Cite This Article
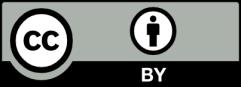