Open Access
ARTICLE
A Time Series Intrusion Detection Method Based on SSAE, TCN and Bi-LSTM
School of Cyberspace Security, Gansu University of Political Science and Law, Lanzhou, 730000, China
* Corresponding Author: Zhenxiang He. Email:
Computers, Materials & Continua 2024, 78(1), 845-871. https://doi.org/10.32604/cmc.2023.046607
Received 08 October 2023; Accepted 23 November 2023; Issue published 30 January 2024
Abstract
In the fast-evolving landscape of digital networks, the incidence of network intrusions has escalated alarmingly. Simultaneously, the crucial role of time series data in intrusion detection remains largely underappreciated, with most systems failing to capture the time-bound nuances of network traffic. This leads to compromised detection accuracy and overlooked temporal patterns. Addressing this gap, we introduce a novel SSAE-TCN-BiLSTM (STL) model that integrates time series analysis, significantly enhancing detection capabilities. Our approach reduces feature dimensionality with a Stacked Sparse Autoencoder (SSAE) and extracts temporally relevant features through a Temporal Convolutional Network (TCN) and Bidirectional Long Short-term Memory Network (Bi-LSTM). By meticulously adjusting time steps, we underscore the significance of temporal data in bolstering detection accuracy. On the UNSW-NB15 dataset, our model achieved an F1-score of 99.49%, Accuracy of 99.43%, Precision of 99.38%, Recall of 99.60%, and an inference time of 4.24 s. For the CICDS2017 dataset, we recorded an F1-score of 99.53%, Accuracy of 99.62%, Precision of 99.27%, Recall of 99.79%, and an inference time of 5.72 s. These findings not only confirm the STL model’s superior performance but also its operational efficiency, underpinning its significance in real-world cybersecurity scenarios where rapid response is paramount. Our contribution represents a significant advance in cybersecurity, proposing a model that excels in accuracy and adaptability to the dynamic nature of network traffic, setting a new benchmark for intrusion detection systems.Keywords
Cite This Article
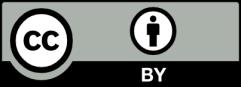
This work is licensed under a Creative Commons Attribution 4.0 International License , which permits unrestricted use, distribution, and reproduction in any medium, provided the original work is properly cited.