Open Access
ARTICLE
A Real-Time Small Target Vehicle Detection Algorithm with an Improved YOLOv5m Network Model
School of Automation, Xi’an University of Posts and Telecommunications, Xi’an, 710121, China
* Corresponding Author: Xiangkui Jiang. Email:
(This article belongs to the Special Issue: Machine Vision Detection and Intelligent Recognition)
Computers, Materials & Continua 2024, 78(1), 303-327. https://doi.org/10.32604/cmc.2023.046068
Received 17 September 2023; Accepted 14 November 2023; Issue published 30 January 2024
Abstract
To address the challenges of high complexity, poor real-time performance, and low detection rates for small target vehicles in existing vehicle object detection algorithms, this paper proposes a real-time lightweight architecture based on You Only Look Once (YOLO) v5m. Firstly, a lightweight upsampling operator called Content-Aware Reassembly of Features (CARAFE) is introduced in the feature fusion layer of the network to maximize the extraction of deep-level features for small target vehicles, reducing the missed detection rate and false detection rate. Secondly, a new prediction layer for tiny targets is added, and the feature fusion network is redesigned to enhance the detection capability for small targets. Finally, this paper applies L1 regularization to train the improved network, followed by pruning and fine-tuning operations to remove redundant channels, reducing computational and parameter complexity and enhancing the detection efficiency of the network. Training is conducted on the VisDrone2019-DET dataset. The experimental results show that the proposed algorithm reduces parameters and computation by 63.8% and 65.8%, respectively. The average detection accuracy improves by 5.15%, and the detection speed reaches 47 images per second, satisfying real-time requirements. Compared with existing approaches, including YOLOv5m and classical vehicle detection algorithms, our method achieves higher accuracy and faster speed for real-time detection of small target vehicles in edge computing.Keywords
Cite This Article
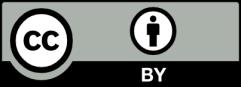
This work is licensed under a Creative Commons Attribution 4.0 International License , which permits unrestricted use, distribution, and reproduction in any medium, provided the original work is properly cited.