Open Access
ARTICLE
Local Adaptive Gradient Variance Attack for Deep Fake Fingerprint Detection
1 Engineering Research Center of Digital Forensics, Ministry of Education, Nanjing University of Information Science and Technology, Nanjing, 210044, China
2 School of Computer Science, Nanjing University of Information Science and Technology, Nanjing, 210044, China
3 Institute of Artificial Intelligence and Blockchain, Guangzhou University, Guangzhou, 510006, China
4 School of International Relations, National University of Defense Technology, Nanjing, 210039, China
5 Department of Electrical and Computer Engineering, University of Windsor, Windsor, N9B 3P4, Canada
* Corresponding Author: Xinting Li. Email:
Computers, Materials & Continua 2024, 78(1), 899-914. https://doi.org/10.32604/cmc.2023.045854
Received 09 September 2023; Accepted 20 November 2023; Issue published 30 January 2024
Abstract
In recent years, deep learning has been the mainstream technology for fingerprint liveness detection (FLD) tasks because of its remarkable performance. However, recent studies have shown that these deep fake fingerprint detection (DFFD) models are not resistant to attacks by adversarial examples, which are generated by the introduction of subtle perturbations in the fingerprint image, allowing the model to make fake judgments. Most of the existing adversarial example generation methods are based on gradient optimization, which is easy to fall into local optimal, resulting in poor transferability of adversarial attacks. In addition, the perturbation added to the blank area of the fingerprint image is easily perceived by the human eye, leading to poor visual quality. In response to the above challenges, this paper proposes a novel adversarial attack method based on local adaptive gradient variance for DFFD. The ridge texture area within the fingerprint image has been identified and designated as the region for perturbation generation. Subsequently, the images are fed into the targeted white-box model, and the gradient direction is optimized to compute gradient variance. Additionally, an adaptive parameter search method is proposed using stochastic gradient ascent to explore the parameter values during adversarial example generation, aiming to maximize adversarial attack performance. Experimental results on two publicly available fingerprint datasets show that our method achieves higher attack transferability and robustness than existing methods, and the perturbation is harder to perceive.Keywords
Cite This Article
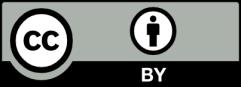
This work is licensed under a Creative Commons Attribution 4.0 International License , which permits unrestricted use, distribution, and reproduction in any medium, provided the original work is properly cited.