Open Access
ARTICLE
IndRT-GCNets: Knowledge Reasoning with Independent Recurrent Temporal Graph Convolutional Representations
1 College of Information Science and Engineering, Xinjiang University, Urumqi, 830017, China
2 Xinjiang Laboratory of Multilanguage Information Technology, Xinjiang University, Urumqi, 830017, China
3 The Base of Kazakh and Kirghiz Language of National Language Resource Monitoring and Research Center on Minority Languages, Xinjiang University, Urumqi, 830017, China
* Corresponding Author: Gulila Altenbek. Email:
Computers, Materials & Continua 2024, 78(1), 695-712. https://doi.org/10.32604/cmc.2023.045486
Received 28 August 2023; Accepted 20 November 2023; Issue published 30 January 2024
Abstract
Due to the structural dependencies among concurrent events in the knowledge graph and the substantial amount of sequential correlation information carried by temporally adjacent events, we propose an Independent Recurrent Temporal Graph Convolution Networks (IndRT-GCNets) framework to efficiently and accurately capture event attribute information. The framework models the knowledge graph sequences to learn the evolutionary representations of entities and relations within each period. Firstly, by utilizing the temporal graph convolution module in the evolutionary representation unit, the framework captures the structural dependency relationships within the knowledge graph in each period. Meanwhile, to achieve better event representation and establish effective correlations, an independent recurrent neural network is employed to implement auto-regressive modeling. Furthermore, static attributes of entities in the entity-relation events are constrained and merged using a static graph constraint to obtain optimal entity representations. Finally, the evolution of entity and relation representations is utilized to predict events in the next subsequent step. On multiple real-world datasets such as Freebase13 (FB13), Freebase 15k (FB15K), WordNet11 (WN11), WordNet18 (WN18), FB15K-237, WN18RR, YAGO3-10, and Nell-995, the results of multiple evaluation indicators show that our proposed IndRT-GCNets framework outperforms most existing models on knowledge reasoning tasks, which validates the effectiveness and robustness.Keywords
Cite This Article
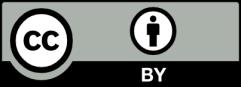
This work is licensed under a Creative Commons Attribution 4.0 International License , which permits unrestricted use, distribution, and reproduction in any medium, provided the original work is properly cited.