Open Access
ARTICLE
Multi-Stream Temporally Enhanced Network for Video Salient Object Detection
School of Computer Science, Jiangsu University of Science and Technology, Zhenjiang, 212100, China
* Corresponding Author: Dan Xu. Email:
(This article belongs to the Special Issue: Development and Industrial Application of AI Technologies)
Computers, Materials & Continua 2024, 78(1), 85-104. https://doi.org/10.32604/cmc.2023.045258
Received 21 August 2023; Accepted 08 November 2023; Issue published 30 January 2024
Abstract
Video salient object detection (VSOD) aims at locating the most attractive objects in a video by exploring the spatial and temporal features. VSOD poses a challenging task in computer vision, as it involves processing complex spatial data that is also influenced by temporal dynamics. Despite the progress made in existing VSOD models, they still struggle in scenes of great background diversity within and between frames. Additionally, they encounter difficulties related to accumulated noise and high time consumption during the extraction of temporal features over a long-term duration. We propose a multi-stream temporal enhanced network (MSTENet) to address these problems. It investigates saliency cues collaboration in the spatial domain with a multi-stream structure to deal with the great background diversity challenge. A straightforward, yet efficient approach for temporal feature extraction is developed to avoid the accumulative noises and reduce time consumption. The distinction between MSTENet and other VSOD methods stems from its incorporation of both foreground supervision and background supervision, facilitating enhanced extraction of collaborative saliency cues. Another notable differentiation is the innovative integration of spatial and temporal features, wherein the temporal module is integrated into the multi-stream structure, enabling comprehensive spatial-temporal interactions within an end-to-end framework. Extensive experimental results demonstrate that the proposed method achieves state-of-the-art performance on five benchmark datasets while maintaining a real-time speed of 27 fps (Titan XP). Our code and models are available at .Keywords
Cite This Article
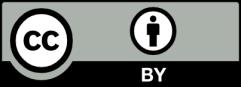
This work is licensed under a Creative Commons Attribution 4.0 International License , which permits unrestricted use, distribution, and reproduction in any medium, provided the original work is properly cited.