Open Access
ARTICLE
A Novel Fall Detection Framework Using Skip-DSCGAN Based on Inertial Sensor Data
College of Information Engineering, China Jiliang University, Hangzhou, 310018, China
* Corresponding Author: Julong Pan. Email:
(This article belongs to the Special Issue: Development and Industrial Application of AI Technologies)
Computers, Materials & Continua 2024, 78(1), 493-514. https://doi.org/10.32604/cmc.2023.045008
Received 14 August 2023; Accepted 10 November 2023; Issue published 30 January 2024
Abstract
With the widespread use of Internet of Things (IoT) technology in daily life and the considerable safety risks of falls for elderly individuals, research on IoT-based fall detection systems has gained much attention. This paper proposes an IoT-based spatiotemporal data processing framework based on a depthwise separable convolution generative adversarial network using skip-connection (Skip-DSCGAN) for fall detection. The method uses spatiotemporal data from accelerometers and gyroscopes in inertial sensors as input data. A semisupervised learning approach is adopted to train the model using only activities of daily living (ADL) data, which can avoid data imbalance problems. Furthermore, a quantile-based approach is employed to determine the fall threshold, which makes the fall detection framework more robust. This proposed fall detection framework is evaluated against four other generative adversarial network (GAN) models with superior anomaly detection performance using two fall public datasets (SisFall & MobiAct). The test results show that the proposed method achieves better results, reaching 96.93% and 92.75% accuracy on the above two test datasets, respectively. At the same time, the proposed method also achieves satisfactory results in terms of model size and inference delay time, making it suitable for deployment on wearable devices with limited resources. In addition, this paper also compares GAN-based semisupervised learning methods with supervised learning methods commonly used in fall detection. It clarifies the advantages of GAN-based semisupervised learning methods in fall detection.Keywords
Cite This Article
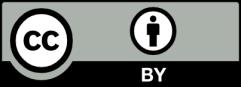
This work is licensed under a Creative Commons Attribution 4.0 International License , which permits unrestricted use, distribution, and reproduction in any medium, provided the original work is properly cited.