Open Access
ARTICLE
Explainable Conformer Network for Detection of COVID-19 Pneumonia from Chest CT Scan: From Concepts toward Clinical Explainability
1 Faculty of Computers and Informatics, Zagazig University, Zagazig, 44519, Egypt
2 Department of Computational Mathematics, Science, and Engineering (CMSE), Michigan State University, East Lansing, MI, 48824, USA
3 Department of Mathematics, Faculty of Science, Mansoura University, Mansoura, 35516, Egypt
4 Department of Statistics and Operations Research, College of Science, King Saud University, P.O. Box 2455, Riyadh, 11451, Saudi Arabia
* Corresponding Author: Mohamed Abouhawwash. Email:
Computers, Materials & Continua 2024, 78(1), 1171-1187. https://doi.org/10.32604/cmc.2023.044425
Received 30 July 2023; Accepted 29 November 2023; Issue published 30 January 2024
Abstract
The early implementation of treatment therapies necessitates the swift and precise identification of COVID-19 pneumonia by the analysis of chest CT scans. This study aims to investigate the indispensable need for precise and interpretable diagnostic tools for improving clinical decision-making for COVID-19 diagnosis. This paper proposes a novel deep learning approach, called Conformer Network, for explainable discrimination of viral pneumonia depending on the lung Region of Infections (ROI) within a single modality radiographic CT scan. Firstly, an efficient U-shaped transformer network is integrated for lung image segmentation. Then, a robust transfer learning technique is introduced to design a robust feature extractor based on pre-trained lightweight Big Transfer (BiT-L) and finetuned on medical data to effectively learn the patterns of infection in the input image. Secondly, this work presents a visual explanation method to guarantee clinical explainability for decisions made by Conformer Network. Experimental evaluation of real-world CT data demonstrated that the diagnostic accuracy of our model outperforms cutting-edge studies with statistical significance. The Conformer Network achieves 97.40% of detection accuracy under cross-validation settings. Our model not only achieves high sensitivity and specificity but also affords visualizations of salient features contributing to each classification decision, enhancing the overall transparency and trustworthiness of our model. The findings provide obvious implications for the ability of our model to empower clinical staff by generating transparent intuitions about the features driving diagnostic decisions.Keywords
Cite This Article
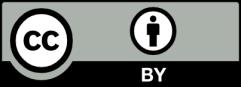