Open Access
ARTICLE
Deep Learning-Based Classification of Rotten Fruits and Identification of Shelf Life
1 Centre for Smart Grid Technologies, School of Electronics Engineering, Vellore Institute of Technology, Chennai, 600127, India
2 School of Electronics Engineering, Vellore Institute of Technology, Chennai, 600127, India
3 Department of Electrical Engineering, Dr. B R Ambedkar National Institute of Technology, Jalandhar, Punjab, 144008, India
* Corresponding Author: Prakash Venugopal. Email:
Computers, Materials & Continua 2024, 78(1), 781-794. https://doi.org/10.32604/cmc.2023.043369
Received 30 June 2023; Accepted 10 November 2023; Issue published 30 January 2024
Abstract
The freshness of fruits is considered to be one of the essential characteristics for consumers in determining their quality, flavor and nutritional value. The primary need for identifying rotten fruits is to ensure that only fresh and high-quality fruits are sold to consumers. The impact of rotten fruits can foster harmful bacteria, molds and other microorganisms that can cause food poisoning and other illnesses to the consumers. The overall purpose of the study is to classify rotten fruits, which can affect the taste, texture, and appearance of other fresh fruits, thereby reducing their shelf life. The agriculture and food industries are increasingly adopting computer vision technology to detect rotten fruits and forecast their shelf life. Hence, this research work mainly focuses on the Convolutional Neural Network’s (CNN) deep learning model, which helps in the classification of rotten fruits. The proposed methodology involves real-time analysis of a dataset of various types of fruits, including apples, bananas, oranges, papayas and guavas. Similarly, machine learning models such as Gaussian Naïve Bayes (GNB) and random forest are used to predict the fruit’s shelf life. The results obtained from the various pre-trained models for rotten fruit detection are analysed based on an accuracy score to determine the best model. In comparison to other pre-trained models, the visual geometry group16 (VGG16) obtained a higher accuracy score of 95%. Likewise, the random forest model delivers a better accuracy score of 88% when compared with GNB in forecasting the fruit’s shelf life. By developing an accurate classification model, only fresh and safe fruits reach consumers, reducing the risks associated with contaminated produce. Thereby, the proposed approach will have a significant impact on the food industry for efficient fruit distribution and also benefit customers to purchase fresh fruits.Keywords
Cite This Article
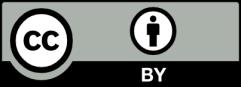