Open Access
ARTICLE
Efficient Object Segmentation and Recognition Using Multi-Layer Perceptron Networks
1 Department of Computer Science, Air University, Islamabad, 44000, Pakistan
2 Department of Information Systems, College of Computer and Information Sciences, Princess Nourah bint Abdulrahman University, P.O. Box 84428, Riyadh, 11671, Saudi Arabia
3 Information System Department, Umm Al-Qura University, Makkah, Saudi Arabia
4 Department of Computer Science, College of Computer Science and Information System, Najran University, Najran, 55461, Saudi Arabia
5 Department of Computer Engineering, Tech University of Korea, Gyeonggi-do, 15073, South Korea
* Corresponding Author: Jeongmin Park. Email:
Computers, Materials & Continua 2024, 78(1), 1381-1398. https://doi.org/10.32604/cmc.2023.042963
Received 17 June 2023; Accepted 13 November 2023; Issue published 30 January 2024
Abstract
Object segmentation and recognition is an imperative area of computer vision and machine learning that identifies and separates individual objects within an image or video and determines classes or categories based on their features. The proposed system presents a distinctive approach to object segmentation and recognition using Artificial Neural Networks (ANNs). The system takes RGB images as input and uses a k-means clustering-based segmentation technique to fragment the intended parts of the images into different regions and label them based on their characteristics. Then, two distinct kinds of features are obtained from the segmented images to help identify the objects of interest. An Artificial Neural Network (ANN) is then used to recognize the objects based on their features. Experiments were carried out with three standard datasets, MSRC, MS COCO, and Caltech 101 which are extensively used in object recognition research, to measure the productivity of the suggested approach. The findings from the experiment support the suggested system’s validity, as it achieved class recognition accuracies of 89%, 83%, and 90.30% on the MSRC, MS COCO, and Caltech 101 datasets, respectively.Keywords
Cite This Article
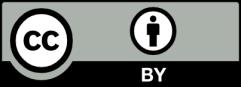
This work is licensed under a Creative Commons Attribution 4.0 International License , which permits unrestricted use, distribution, and reproduction in any medium, provided the original work is properly cited.