Open Access
ARTICLE
Image Splicing Forgery Detection Using Feature-Based of Sonine Functions and Deep Features
1 Department of Computer Science, College of Computer & Information Sciences, Prince Sultan University, Riyadh, 11586, Saudi Arabia
2 Department of Computer Science and Mathematics, Lebanese American University, Beirut, 1102 2801, Lebanon
3 Department of Mathematics, Mathematics Research Center, Near East University, Nicosia, 99138, Turkey
4 Information and Communication Technology Research Group, Scientific Research Center, Alayen University, Dhi Qar, 64001, Iraq
* Corresponding Author: Rabha W. Ibrahim. Email:
Computers, Materials & Continua 2024, 78(1), 795-810. https://doi.org/10.32604/cmc.2023.042755
Received 11 June 2023; Accepted 13 September 2023; Issue published 30 January 2024
Abstract
The growing prevalence of fake images on the Internet and social media makes image integrity verification a crucial research topic. One of the most popular methods for manipulating digital images is image splicing, which involves copying a specific area from one image and pasting it into another. Attempts were made to mitigate the effects of image splicing, which continues to be a significant research challenge. This study proposes a new splicing detection model, combining Sonine functions-derived convex-based features and deep features. Two stages make up the proposed method. The first step entails feature extraction, then classification using the “support vector machine” (SVM) to differentiate authentic and spliced images. The proposed Sonine functions-based feature extraction model reveals the spliced texture details by extracting some clues about the probability of image pixels. The proposed model achieved an accuracy of 98.93% when tested with the CASIA V2.0 dataset “Chinese Academy of Sciences, Institute of Automation” which is a publicly available dataset for forgery classification. The experimental results show that, for image splicing forgery detection, the proposed Sonine functions-derived convex-based features and deep features outperform state-of-the-art techniques in terms of accuracy, precision, and recall. Overall, the obtained detection accuracy attests to the benefit of using the Sonine functions alongside deep feature representations. Finding the regions or locations where image tampering has taken place is limited by the study. Future research will need to look into advanced image analysis techniques that can offer a higher degree of accuracy in identifying and localizing tampering regions.Keywords
Cite This Article
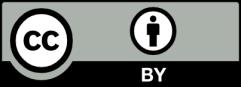
This work is licensed under a Creative Commons Attribution 4.0 International License , which permits unrestricted use, distribution, and reproduction in any medium, provided the original work is properly cited.