Open Access
ARTICLE
Novel Rifle Number Recognition Based on Improved YOLO in Military Environment
1 Department of Artificial Intelligence and Data Science, Korea Military Academy, Seoul, Korea
2 Graduate School of Information Security, Korea Advanced Institute of Science and Technology, Daejeon, Korea
* Corresponding Author: Hyun Kwon. Email:
Computers, Materials & Continua 2024, 78(1), 249-263. https://doi.org/10.32604/cmc.2023.042466
Received 31 May 2023; Accepted 25 July 2023; Issue published 30 January 2024
Abstract
Deep neural networks perform well in image recognition, object recognition, pattern analysis, and speech recognition. In military applications, deep neural networks can detect equipment and recognize objects. In military equipment, it is necessary to detect and recognize rifle management, which is an important piece of equipment, using deep neural networks. There have been no previous studies on the detection of real rifle numbers using real rifle image datasets. In this study, we propose a method for detecting and recognizing rifle numbers when rifle image data are insufficient. The proposed method was designed to improve the recognition rate of a specific dataset using data fusion and transfer learning methods. In the proposed method, real rifle images and existing digit images are fused as training data, and the final layer is transferred to the Yolov5 algorithm model. The detection and recognition performance of rifle numbers was improved and analyzed using rifle image and numerical datasets. We used actual rifle image data (K-2 rifle) and numeric image datasets, as an experimental environment. TensorFlow was used as the machine learning library. Experimental results show that the proposed method maintains 84.42% accuracy, 73.54% precision, 81.81% recall, and 77.46% F1-score in detecting and recognizing rifle numbers. The proposed method is effective in detecting rifle numbers.Keywords
Cite This Article
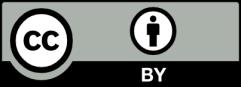
This work is licensed under a Creative Commons Attribution 4.0 International License , which permits unrestricted use, distribution, and reproduction in any medium, provided the original work is properly cited.